“大数据”的版本间的差异
(→技术) |
|||
第308行: | 第308行: | ||
===COVID-19=== | ===COVID-19=== | ||
+ | 在2019冠状病毒疾病流行期间,大数据被认为是减少疾病影响的一种方法。大数据的重要应用包括最小化病毒传播、病例识别和医疗发展。<ref>{{cite journal |last1=Haleem |first1=Abid |last2=Javaid |first2=Mohd |last3=Khan |first3=Ibrahim |last4=Vaishya |first4=Raju |title=Significant Applications of Big Data in COVID-19 Pandemic |journal=Indian Journal of Orthopaedics |date=2020 |volume=54 |issue=4 |pages=526–528 |doi=10.1007/s43465-020-00129-z |pmid=32382166 |pmc=7204193 }}</ref> | ||
− | |||
− | |||
− | |||
− | |||
− | |||
− | |||
− | |||
− | |||
− | |||
− | |||
+ | 各国政府使用大数据追踪感染者,以最大限度地减少传播。早期采用者包括中国、台湾、韩国和以色列。<ref>{{cite news |last1=Manancourt |first1=Vincent |title=Coronavirus tests Europe's resolve on privacy |url=https://www.politico.eu/article/coronavirus-tests-europe-resolve-on-privacy-tracking-apps-germany-italy/ |access-date=30 October 2020 |work=Politico |date=10 March 2020}}</ref><ref>{{cite news |last1=Choudhury |first1=Amit Roy |title=Gov in the Time of Corona |url=https://govinsider.asia/innovation/gov-in-the-time-of-corona/ |access-date=30 October 2020 |work=Gov Insider |date=27 March 2020}}</ref><ref>{{cite news |last1=Cellan-Jones |first1=Rory |title=China launches coronavirus 'close contact detector' app |url=https://www.bbc.com/news/technology-51439401 |access-date=30 October 2020 |work=BBC |date=11 February 2020|archive-url=https://web.archive.org/web/20200228003957/https://www.bbc.com/news/technology-51439401 |archive-date=28 February 2020 }}</ref> | ||
== 研究活动 == | == 研究活动 == | ||
− | + | 2014年3月,美国工程教育学会(American Society of Engineering Education)展示了大数据中的加密搜索和集群形成。麻省理工学院计算机科学和人工智能实验室的Gautam Siwach和UNH研究小组的Amir Esmailpour致力于解决大数据的挑战,他们研究了大数据的关键特征,如集群的形成及其相互关联。他们通过提供技术中的原始定义和实时示例,重点关注大数据的安全性,以及该术语在云接口以加密形式存在不同类型数据的方向。此外,他们还提出了一种识别编码技术的方法,以加快对加密文本的搜索,从而增强大数据的安全性。<ref>{{cite conference |url=http://asee-ne.org/proceedings/2014/Student%20Papers/210.pdf |title=Encrypted Search & Cluster Formation in Big Data |last1=Siwach |first1=Gautam |last2=Esmailpour |first2=Amir |date=March 2014 |conference=ASEE 2014 Zone I Conference |conference-url=http://ubconferences.org/ |location=[[University of Bridgeport]], [[Bridgeport, Connecticut|Bridgeport]], Connecticut, US |access-date=26 July 2014 |archive-url=https://web.archive.org/web/20140809045242/http://asee-ne.org/proceedings/2014/Student%20Papers/210.pdf |archive-date=9 August 2014 |url-status=dead }}</ref> | |
− | |||
− | |||
− | |||
− | |||
− | |||
− | |||
− | |||
− | |||
− | |||
− | |||
− | |||
− | |||
− | |||
− | |||
− | |||
− | |||
− | |||
− | |||
− | |||
− | |||
− | |||
− | |||
− | |||
− | |||
− | |||
− | |||
− | |||
− | |||
− | |||
− | |||
− | |||
− | |||
− | |||
− | + | 2012年3月,白宫宣布了一项国家“大数据倡议”,由六个联邦部门和机构组成,承诺向大数据研究项目投入2亿多美元。<ref>{{cite web|title=Obama Administration Unveils "Big Data" Initiative:Announces $200 Million in New R&D Investments| url=https://obamawhitehouse.archives.gov/sites/default/files/microsites/ostp/big_data_press_release_final_2.pdf |url-status =live| archive-url =https://web.archive.org/web/20170121233309/https://obamawhitehouse.archives.gov/sites/default/files/microsites/ostp/big_data_press_release_final_2.pdf |via=[[NARA|National Archives]]|work=[[Office of Science and Technology Policy]]|archive-date=21 January 2017}}</ref> | |
− | |||
− | + | 该举措包括一个国家科学基金会“计算远征”,该项目将在五年内向加州大学伯克利分校的 AMPLab 提供1000万美元的资助。<ref>{{cite web|url=http://amplab.cs.berkeley.edu |title=AMPLab at the University of California, Berkeley |publisher=Amplab.cs.berkeley.edu |access-date=5 March 2013}}</ref> at the University of California, Berkeley.<ref>{{cite web |title=NSF Leads Federal Efforts in Big Data|date=29 March 2012|publisher=National Science Foundation (NSF) |url= https://www.nsf.gov/news/news_summ.jsp?cntn_id=123607&org=NSF&from=news}}</ref>AMPLab还从DARPA和十几家行业赞助商那里获得资金,并利用大数据解决从预测交通拥堵<ref>{{cite conference| url=https://amplab.cs.berkeley.edu/publication/scaling-the-mobile-millennium-system-in-the-cloud-2/|author1=Timothy Hunter|date=October 2011|author2=Teodor Moldovan|author3=Matei Zaharia| author4 =Justin Ma|author5=Michael Franklin|author6-link=Pieter Abbeel|author6=Pieter Abbeel|author7=Alexandre Bayen |title=Scaling the Mobile Millennium System in the Cloud}}</ref>到抗击癌症等一系列问题。<ref>{{cite news|title=Computer Scientists May Have What It Takes to Help Cure Cancer|author=David Patterson|work=The New York Times| date=5 December 2011 |url=https://www.nytimes.com/2011/12/06/science/david-patterson-enlist-computer-scientists-in-cancer-fight.html}}</ref> | |
+ | 白宫大数据倡议还包括能源部承诺在五年内提供2500万美元的资金,以建立由能源部劳伦斯·伯克利国家实验室领导的可扩展数据管理、分析和可视化(SDAV)研究所。<ref>{{cite web|title=Secretary Chu Announces New Institute to Help Scientists Improve Massive Data Set Research on DOE Supercomputers |publisher=energy.gov |url=http://energy.gov/articles/secretary-chu-announces-new-institute-help-scientists-improve-massive-data-set-research-doe}}</ref>SDAV研究所旨在汇集六个国家实验室和七所大学的专业知识,开发新的工具,帮助科学家管理和可视化国防部超级计算机上的数据。 | ||
− | |||
− | + | 2012年5月,美国马萨诸塞州宣布了马萨诸塞州大数据计划,该计划由州政府和私营公司向各种研究机构提供资金。<ref>{{Cite news|last=Young|first=Shannon|date=2012-05-30|title=Mass. governor, MIT announce big data initiative|work=Boston.com|url=http://archive.boston.com/news/local/massachusetts/articles/2012/05/30/mass_gov_and_mit_to_announce_data_initiative/|access-date=2021-07-29}}</ref>麻省理工学院(Massachusetts Institute of Technology)在麻省理工学院计算机科学与人工智能实验室(MIT Computer Science and Artificial Intelligence Laboratory)设立了英特尔大数据科学技术中心(Intel Science and Technology Center for Big Data),该中心将政府、企业和机构的资金和研究工作结合起来。<ref>{{cite web|url=http://bigdata.csail.mit.edu/ |title=Big Data @ CSAIL |publisher= Bigdata.csail.mit.edu |date=22 February 2013 |access-date=5 March 2013}}</ref> | |
+ | 欧盟委员会正在通过其第七个框架计划为为期两年的大数据公私论坛提供资金,让公司、学者和其他利益相关者参与讨论大数据问题。该项目旨在确定一项研究和创新战略,以指导欧盟委员会在成功实施大数据经济方面的支持行动。该项目的成果将作为其下一个框架项目“地平线2020”的投入。<ref>{{cite web |url=https://cordis.europa.eu/project/id/318062 |title=Big Data Public Private Forum |publisher=cordis.europa.eu |date=1 September 2012 |access-date=16 March 2020 }}</ref> | ||
− | |||
− | + | 2014年3月,英国政府宣布成立艾伦·图灵研究所(Alan Turing Institute),该研究所以计算机先驱和破译者的名字命名,将专注于收集和分析大型数据集的新方法。<ref>{{cite news|url=https://www.bbc.co.uk/news/technology-26651179|title=Alan Turing Institute to be set up to research big data|work=[[BBC News]]|access-date=19 March 2014|date=19 March 2014}}</ref> | |
+ | 在滑铁卢大学斯特拉特福校园加拿大开放数据体验(CODE)启示日,与会者演示了如何使用数据可视化可以增加对大数据集的理解和吸引力,并向世界传达他们的故事。<ref>{{cite web|url= http://www.betakit.com/event/inspiration-day-at-university-of-waterloo-stratford-campus/| title=Inspiration day at University of Waterloo, Stratford Campus |publisher=betakit.com/ |access-date=28 February 2014}}</ref> | ||
− | |||
− | Big | + | 计算社会科学——任何人都可以使用谷歌和Twitter等大数据持有者提供的应用程序编程接口(API)进行社会和行为科学研究。<ref name=pigdata>{{cite journal|last=Reips|first=Ulf-Dietrich|author2=Matzat, Uwe |title=Mining "Big Data" using Big Data Services |journal=International Journal of Internet Science |year=2014|volume=1|issue=1|pages=1–8 | url=http://www.ijis.net/ijis9_1/ijis9_1_editorial_pre.html}}</ref>这些API通常是免费提供的。<ref name="pigdata" />Tobias Preis等人利用谷歌趋势数据证明,来自人均国内生产总值(GDP)较高国家的互联网用户搜索未来信息的可能性大于搜索过去信息的可能性。研究结果表明,在线行为与现实世界的经济指标之间可能存在联系。<ref>{{cite journal | vauthors = Preis T, Moat HS, Stanley HE, Bishop SR | title = Quantifying the advantage of looking forward | journal = Scientific Reports | volume = 2 | pages = 350 | year = 2012 | pmid = 22482034 | pmc = 3320057 | doi = 10.1038/srep00350 | bibcode = 2012NatSR...2E.350P }}</ref><ref>{{cite news | url=https://www.newscientist.com/article/dn21678-online-searches-for-future-linked-to-economic-success.html | title=Online searches for future linked to economic success |first=Paul |last=Marks |work=New Scientist | date=5 April 2012 | access-date=9 April 2012}}</ref><ref>{{cite news | url=https://arstechnica.com/gadgets/news/2012/04/google-trends-reveals-clues-about-the-mentality-of-richer-nations.ars | title=Google Trends reveals clues about the mentality of richer nations |first=Casey |last=Johnston |work=Ars Technica | date=6 April 2012 | access-date=9 April 2012}}</ref>这项研究的作者根据下一年(2011年)的搜索量与上一年(2009年)的搜索量之比来检查谷歌的查询日志,他们称之为“未来方向指数”。<ref>{{cite web | url = http://www.tobiaspreis.de/bigdata/future_orientation_index.pdf | title = Supplementary Information: The Future Orientation Index is available for download | author = Tobias Preis | date = 24 May 2012 | access-date = 24 May 2012}}</ref>他们将未来导向指数与每个国家的人均GDP进行了比较,发现谷歌用户查询更多关于未来的国家有更高GDP的强烈趋势。 |
− | |||
− | + | Tobias Preis和他的同事Helen Susannah Moat和H.Eugene Stanley介绍了一种方法,使用基于谷歌趋势(Google Trends)提供的搜索量数据的交易策略,识别股市走势的在线前兆。他们在科学报告中对谷歌98个不同财务相关性的搜索量进行的分析表明,财务相关搜索量的增加往往先于金融市场的巨大损失。<ref>{{cite journal | url =http://www.nature.com/news/counting-google-searches-predicts-market-movements-1.12879 | title=Counting Google searches predicts market movements | author=Philip Ball | journal=Nature | date=26 April 2013 | doi=10.1038/nature.2013.12879 | s2cid=167357427 | access-date=9 August 2013| author-link=Philip Ball }}</ref> Their analysis of [[Google]] search volume for 98 terms of varying financial relevance, published in ''[[Scientific Reports]]'',<ref>{{cite journal | vauthors = Preis T, Moat HS, Stanley HE | title = Quantifying trading behavior in financial markets using Google Trends | journal = Scientific Reports | volume = 3 | pages = 1684 | year = 2013 | pmid = 23619126 | pmc = 3635219 | doi = 10.1038/srep01684 | bibcode = 2013NatSR...3E1684P }}</ref> suggests that increases in search volume for financially relevant search terms tend to precede large losses in financial markets.<ref>{{cite news | url=http://bits.blogs.nytimes.com/2013/04/26/google-search-terms-can-predict-stock-market-study-finds/ | title= Google Search Terms Can Predict Stock Market, Study Finds | author=Nick Bilton | work=[[The New York Times]] | date=26 April 2013 | access-date=9 August 2013}}</ref><ref>{{cite magazine | url=http://business.time.com/2013/04/26/trouble-with-your-investment-portfolio-google-it/ | title=Trouble With Your Investment Portfolio? Google It! | author=Christopher Matthews | magazine=[[Time (magazine)|Time]] | date=26 April 2013 | access-date=9 August 2013}}</ref><ref>{{cite journal | url= http://www.nature.com/news/counting-google-searches-predicts-market-movements-1.12879 | title=Counting Google searches predicts market movements | author=Philip Ball |journal=[[Nature (journal)|Nature]] | date=26 April 2013 | doi=10.1038/nature.2013.12879 | s2cid=167357427 | access-date=9 August 2013}}</ref><ref>{{cite news | url=http://www.businessweek.com/articles/2013-04-25/big-data-researchers-turn-to-google-to-beat-the-markets | title='Big Data' Researchers Turn to Google to Beat the Markets | author=Bernhard Warner | work=[[Bloomberg Businessweek]] | date=25 April 2013 | access-date=9 August 2013}}</ref><ref>{{cite news | url=https://www.independent.co.uk/news/business/comment/hamish-mcrae/hamish-mcrae-need-a-valuable-handle-on-investor-sentiment-google-it-8590991.html | title=Hamish McRae: Need a valuable handle on investor sentiment? Google it | author=Hamish McRae | work=[[The Independent]] | date=28 April 2013 | access-date=9 August 2013 | location=London}}</ref><ref>{{cite web | url=http://www.ft.com/intl/cms/s/0/e5d959b8-acf2-11e2-b27f-00144feabdc0.html | title= Google search proves to be new word in stock market prediction | author=Richard Waters | work=[[Financial Times]] | date=25 April 2013 | access-date=9 August 2013}}</ref><ref>{{cite news | url =https://www.bbc.co.uk/news/science-environment-22293693 | title=Google searches predict market moves | author=Jason Palmer | work=[[BBC]] | date=25 April 2013 | access-date=9 August 2013}}</ref> | |
− | |||
− | + | 大数据集带来了以前不存在的算法挑战。因此,一些人认为有必要从根本上改变处理方式。<ref>E. Sejdić (March 2014). "Adapt current tools for use with big data". ''Nature''. '''507''' (7492): 306.</ref> | |
+ | 现代海量数据集(MMD)算法研讨会汇集了计算机科学家、统计学家、数学家和数据分析从业者,讨论大数据的算法挑战。<ref>Stanford. [https://web.stanford.edu/group/mmds/ "MMDS. Workshop on Algorithms for Modern Massive Data Sets"].</ref>关于大数据,这样的量级概念是相对的。正如它所说,“如果说过去的数据有什么指导意义的话,那么今天的大数据在不久的将来很可能不会被认为是这样的。”<ref name=CAD7challenges/> | ||
− | |||
− | |||
=== 大数据采样 === | === 大数据采样 === | ||
− | + | 关于大数据集的一个研究问题是,是否有必要查看完整的数据或者样本要足够好,以得出关于数据属性的某些结论。大数据这个名称本身包含一个与规模相关的术语,这是大数据的一个重要特征。但抽样可以从更大的数据集中选择正确的数据点,以估计整个人口的特征。在制造过程中,不同类型的感官数据(如声学、振动、压力、电流、电压和控制器数据)在短时间间隔内可用。要预测停机时间,可能不需要查看所有数据,仅一个样本就足够了。大数据可以按不同的数据点分类,如人口统计、心理、行为和交易数据。有了大量的数据,营销人员可以创建和使用更多定制的消费者群体,以实现更具战略性的目标。 | |
− | |||
− | |||
− | |||
− | |||
− | |||
− | |||
− | |||
− | |||
− | |||
− | |||
− | 在大数据采样算法方面已经有了一些成果。比如抽样 Twitter 数据的理论公式已被开发出。 | + | 在大数据采样算法方面已经有了一些成果。比如抽样 Twitter 数据的理论公式已被开发出。<ref>{{cite conference |author1=Deepan Palguna |author2= Vikas Joshi |author3=Venkatesan Chakravarthy |author4=Ravi Kothari |author5=L. V. Subramaniam |name-list-style=amp | title=Analysis of Sampling Algorithms for Twitter | journal=[[International Joint Conference on Artificial Intelligence]] | year=2015 }}</ref> |
== 批评 == | == 批评 == | ||
− | + | 针对大数据范式的批评有两种:一种是质疑方法本身,另一种是质疑目前的方法。对这种批评的一个形式是批判性数据研究领域。<ref name="Kimble and Milolidakis (2015)" /> | |
− | |||
− | |||
− | |||
=== 针对大数据范式的批评 === | === 针对大数据范式的批评 === | ||
− | + | “一个关键问题是,我们对导致大数据典型网络特征出现的潜在经验微观过程知之甚少。”<ref name="Editorial" /> Snijders、Matzat和Reips在他们的评论中指出,通常对数学性质做出非常强烈的假设,这些假设可能根本无法反映微观过程上的真实情况。马克·格雷厄姆 Mark Graham批评了克里斯·安德森Chris Anderson关于大数据将意味着理论的终结的断言:<ref>{{Cite magazine|url=https://www.wired.com/science/discoveries/magazine/16-07/pb_theory|title=The End of Theory: The Data Deluge Makes the Scientific Method Obsolete|author=Chris Anderson|date=23 June 2008|magazine=Wired}}</ref>他特别关注大数据必须始终在其社会、经济和政治背景下进行语境化的概念。<ref>{{cite news |author=Graham M. |title=Big data and the end of theory? |newspaper=The Guardian |url= https://www.theguardian.com/news/datablog/2012/mar/09/big-data-theory |location=London |date=9 March 2012}}</ref>尽管公司投入8位数甚至9位数的资金,从供应商和客户的信息流中获取洞察力,但只有不到40%的员工拥有足够成熟的技能。根据《哈佛商业评论》(Harvard Business Review)上的一篇文章,为了克服这种洞察力缺陷,大数据无论多么全面或分析得多么好,都必须辅之以“综合判断力”。<ref>{{cite journal|title=Good Data Won't Guarantee Good Decisions |journal=[[Harvard Business Review]]|url=http://hbr.org/2012/04/good-data-wont-guarantee-good-decisions/ar/1|author=Shah, Shvetank|author2=Horne, Andrew|author3=Capellá, Jaime |access-date=8 September 2012|date=April 2012}}</ref> | |
− | |||
− | |||
− | |||
− | |||
− | |||
− | |||
− | |||
− | |||
− | |||
− | |||
− | |||
− | |||
− | |||
− | |||
− | |||
− | |||
− | |||
− | |||
− | |||
− | |||
− | |||
− | |||
+ | 与此大致相同的是,有人指出,基于大数据分析的决策不可避免地“像过去一样,或者充其量也像现在一样,受到世界的影响”。<ref name="HilbertBigData2013">Hilbert, M. (2016). Big Data for Development: A Review of Promises and Challenges. Development Policy Review, 34(1), 135–174. https://doi.org/10.1111/dpr.12142 free access: https://www.martinhilbert.net/big-data-for-development/</ref>如果未来与过去相似,通过大量关于过去经验的数据,算法可以预测未来的发展。如果系统对未来的动态变化(如果它不是一个平稳的过程),那么过去对未来的影响就很小。<ref name="HilbertTEDx">[https://www.youtube.com/watch?v=UXef6yfJZAI Big Data requires Big Visions for Big Change.], Hilbert, M. (2014). London: TEDx UCL, x=independently organized TED talks</ref>为了在不断变化的环境中做出预测,有必要对系统动力学有一个透彻的了解。<ref name="HilbertTEDx"/>作为对这一批评的回应,Alemany Oliver和Vayre建议使用“诱因推理作为研究过程的第一步,以便为消费者的数字痕迹提供背景,并使新的理论出现”。<ref>{{cite journal|last=Alemany Oliver|first=Mathieu |author2=Vayre, Jean-Sebastien |s2cid=111360835 |title= Big Data and the Future of Knowledge Production in Marketing Research: Ethics, Digital Traces, and Abductive Reasoning|journal=Journal of Marketing Analytics |year=2015|volume=3|issue=1|doi= 10.1057/jma.2015.1|pages=5–13}}</ref>此外,有人建议将大数据方法与计算机模拟相结合,例如基于代理的模型<ref name="HilbertBigData2013" />和复杂系统。通过基于一系列相互依赖的算法的计算机模拟,基于代理的模型在预测甚至未知场景的社会复杂性的结果方面越来越好。<ref>{{cite web|url= https://www.theatlantic.com/magazine/archive/2002/04/seeing-around-corners/302471/| title=Seeing Around Corners|author=Jonathan Rauch|date=1 April 2002|work=[[The Atlantic]]}}</ref><ref>Epstein, J. M., & Axtell, R. L. (1996). Growing Artificial Societies: Social Science from the Bottom Up. A Bradford Book.</ref>最后,探索数据潜在结构的多变量方法的使用,如因子分析和聚类分析,已被证明是有用的分析方法,远远超出了通常用于较小数据集的双变量方法。 | ||
− | + | 在健康和生物学领域,传统的科学方法是基于实验的。对于这些方法,限制因素是相关的数据,可以证实或反驳最初的假设。<ref>{{cite web|url=http://www.bigdataparis.com/documents/Pierre-Delort-INSERM.pdf#page=5| title=Delort P., Big data in Biosciences, Big Data Paris, 2012|website =Bigdataparis.com |access-date=8 October 2017}}</ref>如今,生物科学界接受了一个新的假设:大量数据(组学)提供的信息(无需事先假设)是对基于实验的传统方法的补充,有时是必需的。<ref>{{cite web|url=https://www.cs.cmu.edu/~durand/03-711/2011/Literature/Next-Gen-Genomics-NRG-2010.pdf|title=Next-generation genomics: an integrative approach|date=July 2010|publisher=nature|access-date=18 October 2016}}</ref><ref>{{cite web|url= https://www.researchgate.net/publication/283298499|title=Big Data in Biosciences| date=October 2015|access-date=18 October 2016}}</ref>在大数据方法中,相关假设的表述是解释数据的限制因素。<ref>{{cite news|url=https://next.ft.com/content/21a6e7d8-b479-11e3-a09a-00144feabdc0|title=Big data: are we making a big mistake?|date=28 March 2014|work=Financial Times|access-date=20 October 2016}}</ref>搜索逻辑被颠倒以及归纳的局限性是需要考虑的。 | |
− | |||
+ | 隐私倡导者担心个人身份信息的存储和收集增加了对隐私的威胁;专家小组发布了各种政策建议,以使实践符合对隐私的期望。<ref>{{cite magazine |first=Paul |last=Ohm |title=Don't Build a Database of Ruin |magazine=Harvard Business Review |url=http://blogs.hbr.org/cs/2012/08/dont_build_a_database_of_ruin.html|date=23 August 2012 }}</ref> 媒体、公司甚至政府在几起案件中滥用大数据,导致几乎所有支撑社会的基本机构都失去了信任。<ref>Bond-Graham, Darwin (2018). [https://www.theperspective.com/debates/the-perspective-on-big-data/ "The Perspective on Big Data"]. [[The Perspective]].</ref> | ||
− | |||
− | = | + | Nayef Al-Rodhan认为,在大数据和拥有大量信息的大公司的背景下,需要一种新的社会契约来保护个人自由,大数据的使用应该在国家和国际层面受到更好的监管。<ref>{{Cite news|url=http://hir.harvard.edu/the-social-contract-2-0-big-data-and-the-need-to-guarantee-privacy-and-civil-liberties/|title=The Social Contract 2.0: Big Data and the Need to Guarantee Privacy and Civil Liberties – Harvard International Review|last=Al-Rodhan|first=Nayef|date=16 September 2014|work=Harvard International Review|access-date=3 April 2017|archive-url=https://web.archive.org/web/20170413090835/http://hir.harvard.edu/the-social-contract-2-0-big-data-and-the-need-to-guarantee-privacy-and-civil-liberties/|archive-date=13 April 2017|url-status=dead}}</ref>Barocas和Nissenbaum认为,保护个人用户的一种方法是,让用户了解所收集的信息类型、与谁共享信息、在什么约束下以及出于什么目的。<ref>{{Cite book|title=Big Data's End Run around Anonymity and Consent| last1 =Barocas |first1=Solon |last2=Nissenbaum |first2=Helen|last3=Lane|first3=Julia|last4=Stodden|first4=Victoria|last5=Bender|first5=Stefan|last6=Nissenbaum|first6=Helen| s2cid =152939392|date=June 2014| publisher =Cambridge University Press|isbn=9781107067356|pages=44–75|doi =10.1017/cbo9781107590205.004}}</ref> |
− | |||
− | |||
− | |||
− | |||
− | |||
=== 针对“ v”模型的批评 === | === 针对“ v”模型的批评 === | ||
− | 大数据的“V”模型令人担忧,因为它以计算的可延展性为中心,缺乏信息的可感知性和可理解性。这导致了认知大数据框架的形成,该框架根据以下特点描述了大数据应用: | + | 大数据的“V”模型令人担忧,因为它以计算的可延展性为中心,缺乏信息的可感知性和可理解性。这导致了认知大数据框架的形成,该框架根据以下特点描述了大数据应用:<ref>{{Cite journal|last1=Lugmayr|first1=Artur|last2=Stockleben|first2=Bjoern|last3=Scheib|first3=Christoph|last4=Mailaparampil|first4=Mathew|last5=Mesia|first5=Noora|last6=Ranta|first6=Hannu|last7=Lab|first7=Emmi|date=1 June 2016|title=A Comprehensive Survey On Big-Data Research and Its Implications – What is Really 'New' in Big Data? – It's Cognitive Big Data! |url=https://www.researchgate.net/publication/304784955}}</ref> |
− | |||
− | |||
− | |||
− | |||
− | |||
− | |||
− | |||
− | |||
+ | *数据完整性:从数据中理解意义不明的信息。 | ||
+ | *数据相关性、因果关系和可预测性:因果关系不是实现可预测性的必要条件。 | ||
+ | *可解释性和可解释性:人类渴望理解并接受他们所理解的,而算法无法实现这一目标。 | ||
+ | *自动决策的水平:支持自动决策算法和自我学习算法。 | ||
第482行: | 第393行: | ||
=== 针对大数据执行的批评 === | === 针对大数据执行的批评 === | ||
− | + | Ulf Dietrich Reips和Uwe Matzat在2014年写道,大数据已经成为科学研究的“风潮”。<ref name="pigdata" />研究人员Danah Boyd对大数据在科学中的使用提出了担忧,因为研究往往忽略了一些原则,比如选择代表性样本时过于关注处理大量数据,<ref name="danah">{{cite web | url=http://www.danah.org/papers/talks/2010/WWW2010.html | title=Privacy and Publicity in the Context of Big Data | author=danah boyd | work=[[World Wide Web Conference|WWW 2010 conference]] | date=29 April 2010 | access-date = 18 April 2011| author-link=danah boyd }}</ref>这种方法可能会导致结果在某种程度上存在偏差。<ref>{{Cite journal|last=Katyal|first=Sonia K.|date=2019|title=Artificial Intelligence, Advertising, and Disinformation|url=https://muse.jhu.edu/article/745987|journal=Advertising & Society Quarterly|language=en|volume=20|issue=4|doi=10.1353/asr.2019.0026|s2cid=213397212|issn=2475-1790}}</ref>大量异构数据资源的集成(有些被认为是大数据,有些则不是)带来巨大的后勤和分析挑战,但许多研究人员认为,这种集成可能代表着科学领域最有前途的新前沿。<ref>{{cite journal |last1=Jones |first1=MB |last2=Schildhauer |first2=MP |last3=Reichman |first3=OJ |last4=Bowers | first4=S |title=The New Bioinformatics: Integrating Ecological Data from the Gene to the Biosphere | journal=Annual Review of Ecology, Evolution, and Systematics |volume=37 |issue=1 |pages=519–544 |year=2006 |doi=10.1146/annurev.ecolsys.37.091305.110031 |url= http://www.pnamp.org/sites/default/files/Jones2006_AREES.pdf }}</ref>在这篇颇具煽动性的文章《大数据的关键问题》(Critical Questions for Big Data)中,<ref name="danah2">{{cite journal | doi = 10.1080/1369118X.2012.678878| title = Critical Questions for Big Data| journal = Information, Communication & Society| volume = 15| issue = 5| pages = 662–679| year = 2012| last1 = Boyd | first1 = D. | last2 = Crawford | first2 = K. | s2cid = 51843165| hdl = 10983/1320| hdl-access = free}}</ref>作者将大数据称为神话的一部分:“大数据集提供了更高形式的智能和知识……大数据的用户往往“迷失在庞大的数据量中”,而且“使用大数据仍然是主观的,它量化的东西不一定能够更接近客观事实”。<ref name="danah2" />BI领域的最新发展,例如前瞻性报告,特别是通过自动过滤无用数据及相关性来改善大数据的可用性。<ref name="Big Decisions White Paper">[http://www.fortewares.com/Administrator/userfiles/Banner/forte-wares--pro-active-reporting_EN.pdf Failure to Launch: From Big Data to Big Decisions] Forte Wares.</ref>大数据充满了虚假的相关性,<ref>{{Cite web | url=https://www.tylervigen.com/spurious-correlations | title=15 Insane Things That Correlate with Each Other}}</ref>要么是因为非因果巧合(真大数定律),要么是大随机数的唯一性<ref>[https://onlinelibrary.wiley.com/loi/10982418 Random structures & algorithms]</ref> (拉姆齐理论)或其他未发现的因素,因此早期实验者建立大型数字数据库“用数据说话”以及宣称的革新科学方法都受到了质疑。<ref>Cristian S. Calude, Giuseppe Longo, (2016), The Deluge of Spurious Correlations in Big Data, [[Foundations of Science]]</ref> | |
− | |||
− | |||
+ | 与对较小数据集的分析相比,大数据分析往往是肤浅的。<ref name="kdnuggets-berchthold">{{cite web|url=http://www.kdnuggets.com/2014/08/interview-michael-berthold-knime-research-big-data-privacy-part2.html|title=Interview: Michael Berthold, KNIME Founder, on Research, Creativity, Big Data, and Privacy, Part 2|date=12 August 2014|author=Gregory Piatetsky| author-link= Gregory I. Piatetsky-Shapiro|publisher=KDnuggets|access-date=13 August 2014}}</ref>在许多大数据项目中,没有进行大数据分析,但挑战在于提取、转换、加载和预处理数据的部分。<ref name="kdnuggets-berchthold" /> | ||
− | |||
− | + | 大数据是一个时髦的“模糊术语”,<ref>{{cite news|last1=Pelt|first1=Mason|title="Big Data" is an over used buzzword and this Twitter bot proves it|url= http://siliconangle.com/blog/2015/10/26/big-data-is-an-over-used-buzzword-and-this-twitter-bot-proves-it/ |newspaper=Siliconangle|access-date=4 November 2015|date=26 October 2015}}</ref><ref name="ft-harford">{{cite web |url=http://www.ft.com/cms/s/2/21a6e7d8-b479-11e3-a09a-00144feabdc0.html |title=Big data: are we making a big mistake? |last1=Harford |first1=Tim |date=28 March 2014 |website=[[Financial Times]] |access-date=7 April 2014}}</ref>但同时也是企业家、咨询师、科学家和媒体的关注热点。<ref name="ft-harford" />近年来,谷歌流感趋势(Google Flu Trends)等大数据应用在最近几年未能提供好的预测,将流感疫情高估了两倍。类似地,基于Twitter的奥斯卡奖和选举预测往往偏离目标。大数据往往与小数据面临同样的挑战;添加更多数据并不能解决偏见问题,甚至可能会强调其他问题。尤其是Twitter等数据源不能代表整体人口的意见,从这些数据源得出的结果可能会导致错误结论。基于文本大数据统计分析的谷歌翻译在翻译网页方面做得很好。然而,来自专门领域的结果可能会有很大的偏差。另一方面,大数据也可能带来新的问题,比如多重比较问题:同时测试大量假设可能会产生许多错误的结果,这些结果被错误地认为是重要的。Ioannidis认为,“大多数已发表的研究结果都是错误的”,<ref name="Ioannidis">{{cite journal | vauthors = Ioannidis JP | title = Why most published research findings are false | journal = PLOS Medicine | volume = 2 | issue = 8 | pages = e124 | date = August 2005 | pmid = 16060722 | pmc = 1182327 | doi = 10.1371/journal.pmed.0020124 | author-link1 = John P. A. Ioannidis }}</ref>其原因基本上是相同的:当许多科学团队和研究人员各自进行许多实验(即处理大量科学数据;尽管没有使用大数据技术),一个“重大”结果被错误的可能性会迅速增加。而当只有正面的结果被公布时,这种可能性更大。 | |
− | + | 此外,大数据分析结果只能与预测模型一样好。例如,大数据参与了对2016年美国总统选举结果的预测,<ref>{{Cite news|url=https://www.nytimes.com/2016/11/10/technology/the-data-said-clinton-would-win-why-you-shouldnt-have-believed-it.html|title=How Data Failed Us in Calling an Election |last1=Lohr|first1=Steve|date=10 November 2016|last2=Singer|first2=Natasha|newspaper=The New York Times|issn=0362-4331|access-date=27 November 2016}}</ref>并取得了与预测模型类似的不同程度的成功。 | |
− | |||
− | |||
− | |||
− | |||
− | |||
− | |||
− | |||
− | |||
− | |||
− | |||
− | |||
− | |||
− | |||
− | |||
− | |||
− | |||
− | |||
− | |||
− | |||
=== 针对大数据监管和监视批评 === | === 针对大数据监管和监视批评 === | ||
− | + | 大数据已被执法和企业等机构用于警务和监视。<ref>{{Cite news|url=https://www.economist.com/open-future/2018/06/04/how-data-driven-policing-threatens-human-freedom|title=How data-driven policing threatens human freedom|date=4 June 2018|newspaper=The Economist|access-date=27 October 2019|issn=0013-0613}}</ref> 与传统的警务方法相比,基于数据的监控不那么明显,因此反对大数据警务的可能性较小。根据Sarah Brayne的《大数据监控:警务案例 Big Data Surveillance: The Case of Policing》,<ref>{{Cite journal|last=Brayne|first=Sarah|s2cid=3609838|date=29 August 2017|title=Big Data Surveillance: The Case of Policing|journal=American Sociological Review |volume=82|issue=5|pages=977–1008|language=en|doi=10.1177/0003122417725865}}</ref> 大数据警务会通过三种方式加剧现有的社会不平等: | |
− | 通过使用一个数学的无偏算法,将嫌疑犯置于更严格的监视之下。 | + | *通过使用一个数学的无偏算法,将嫌疑犯置于更严格的监视之下。 |
− | 增加执法跟踪的范围和人数,并加剧刑事司法系统中存在的特定种族比例过高的现象。 | + | *增加执法跟踪的范围和人数,并加剧刑事司法系统中存在的特定种族比例过高的现象。 |
− | 鼓励社会成员放弃与产生数字痕迹的机构的互动,从而为社会包容制造障碍。 | + | *鼓励社会成员放弃与产生数字痕迹的机构的互动,从而为社会包容制造障碍。 |
如果以上潜在的问题得不到纠正或监管,大数据监管的影响可能会继续影响社会分化。Brayne还指出,谨慎地使用大数据监管可以防止个人层面的偏见变成制度层面的偏见。 | 如果以上潜在的问题得不到纠正或监管,大数据监管的影响可能会继续影响社会分化。Brayne还指出,谨慎地使用大数据监管可以防止个人层面的偏见变成制度层面的偏见。 | ||
− | == | + | ==在流行文化中== |
− | === | + | ===书籍=== |
− | * | + | *《点球成金》(Moneyball)是一本非虚构的书,书中探讨了探讨奥克兰田径队如何利用统计分析来超越那些预算较大的球队。2011年,由布拉德·皮特主演的改编电影上映。 |
− | * | + | ===电影=== |
+ | *在《美国队长:寒冬战士 Captain America: The Winter Soldier》中,H.Y.D.R.A (伪装成神盾局)开发了一种利用数据来确定和消除全球威胁的飞行母舰。 | ||
− | + | *在《蝙蝠侠: 黑暗骑士 The Dark Knight》中,蝙蝠侠使用的声纳设备可以监视整个哥谭市,这些数据是通过市内居民的手机收集的。 | |
− | |||
− | == | + | == 参见 == |
− | * | + | {{columns-list|colwidth=26em| |
− | * | + | *[[大数据伦理]] |
− | + | *[[大数据成熟度模型]] | |
− | * | + | *[[大内存]] |
− | * | + | *[[数据整理]] |
− | + | *[[数据定义存储]] | |
− | * | + | *[[数据谱系]] |
− | * | + | *[[数据慈善]] |
− | + | *[[数据科学]] | |
+ | *[[数据化]] | ||
+ | *[[面向文档的数据库]] | ||
+ | *[[内存处理]] | ||
+ | *[[城市信息学]] | ||
− | |||
− | |||
− | |||
− | |||
− | |||
− | |||
− | |||
− | |||
− | |||
− | |||
− | |||
− | |||
− | |||
− | |||
− | |||
− | |||
− | |||
− | |||
− | |||
− | |||
− | |||
− | |||
== 参考文献 == | == 参考文献 == | ||
第583行: | 第455行: | ||
}} | }} | ||
− | = 拓展材料 = | + | |
+ | ==拓展材料== | ||
*{{cite magazine|editor1=Peter Kinnaird |editor2=Inbal Talgam-Cohen|magazine=[[XRDS (magazine)|XRDS: Crossroads, The ACM Magazine for Students]]|title=Big Data|volume=19 |issue=1|date=2012|publisher=[[Association for Computing Machinery]]|issn=1528-4980 |oclc=779657714 |url=http://dl.acm.org/citation.cfm?id=2331042}} | *{{cite magazine|editor1=Peter Kinnaird |editor2=Inbal Talgam-Cohen|magazine=[[XRDS (magazine)|XRDS: Crossroads, The ACM Magazine for Students]]|title=Big Data|volume=19 |issue=1|date=2012|publisher=[[Association for Computing Machinery]]|issn=1528-4980 |oclc=779657714 |url=http://dl.acm.org/citation.cfm?id=2331042}} | ||
*{{cite book|title=Mining of massive datasets|author1=Jure Leskovec|author2-link=Anand Rajaraman|author2=Anand Rajaraman|author3-link=Jeffrey D. Ullman|author3=Jeffrey D. Ullman|year=2014|publisher=Cambridge University Press|url=http://mmds.org/|isbn=9781107077232 |oclc=888463433|author1-link=Jure Leskovec}} | *{{cite book|title=Mining of massive datasets|author1=Jure Leskovec|author2-link=Anand Rajaraman|author2=Anand Rajaraman|author3-link=Jeffrey D. Ullman|author3=Jeffrey D. Ullman|year=2014|publisher=Cambridge University Press|url=http://mmds.org/|isbn=9781107077232 |oclc=888463433|author1-link=Jure Leskovec}} | ||
第594行: | 第467行: | ||
− | [[Category: | + | [[Category:大数据]] |
− | [[Category: | + | [[Category:数据管理]] |
− | [[Category: | + | [[Category:分布式计算问题]] |
− | [[Category: | + | [[Category:事务处理]] |
− | [[Category: | + | [[Category:技术预测]] |
− | [[Category: | + | [[Category:数据分析]] |
− | [[Category: | + | [[Category:数据库]] |
2022年3月6日 (日) 19:15的版本
This article is about large collections of data. For the band, see Big Data (band). For the practice of buying and selling of personal and consumer data, see Surveillance capitalism.
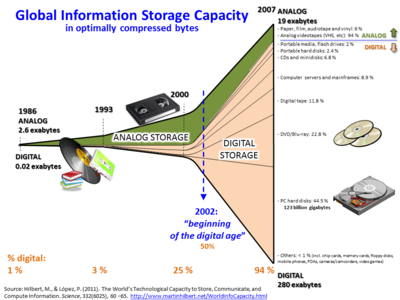
大数据 Big data领域研究如何系统地从传统数据处理应用软件无法处理的太大或太复杂的数据集中提取、分析并处理信息。由于具有多个字段(列)的数据提供了更大的统计能力,同样,具有更高复杂性(更多属性或列)的数据也可能会导致更高的错误率。[2]大数据分析挑战包括捕获数据、数据存储、数据分析、数据搜索、数据共享、数据传输、数据可视化、数据查询、数据更新、信息隐私和数据源。大数据最初与三个关键概念有关:大数据量、多样性和高速度。[3]大数据分析意味着抽样存在挑战,因此旧技术只能进行观察和抽样。而大数据分析通常包括超过传统软件在有限时间及性能内能处理的数据量。
“大数据”一词的当前用法倾向于指预测分析、用户行为分析或其他从大数据中提取价值的高级数据分析方法,很少涉及特定规模的数据集。“毫无疑问,现在可用的数据量确实很大,但这并不是这个新数据生态系统最显著的特征。”[4]对数据集的分析可以揭示“商业趋势、疾病预防、打击犯罪等”的新关联。在互联网搜索、金融科技、医疗分析、地理信息系统、城市信息学和商业信息学等领域,科学家、企业高管、医生、广告和政府都经常面对处理大型数据集的困难。科学家也在电子科学工作中遇到了局限,包括气象学、基因组学、[5]连接组学、复杂物理模拟、生物学和环境研究。[6]
随着移动设备以及众多廉价的信息传感物联网设备、天线(遥感)、软件日志、相机、麦克风、射频识别(RFID)阅读器和无线传感器网络等设备收集数据,可用数据集的规模和数量在迅速增长。[7][8]自20世纪80年代以来,世界人均存储信息的技术能力大约每40个月翻一番,[9] 每天约生成2.5 EB (Exabytes )(2.5×260字节)的数据。[10]根据IDC的一份报告预测,2013年至2020年间,全球数据量将从4.4 ZB (zettabytes)呈指数增长至44 ZB (zettabytes)。IDC还预测,到2025年,数据量将达到163兆字节。[11]因此大型企业正面临的问题是,谁应该开始计划覆盖全企业的大数据转型计划。[12]
用于可视化数据的关系型数据库管理系统 Relational Database Management Systems和桌面统计软件包通常难以处理和分析大数据。大数据的处理和分析可能需要“运行在数十台、数百台甚至数千台服务器上的大规模软件”,[13]“大数据”的具体定义取决于分析者及其工具的能力。此外,不断扩充数据的能力使大数据的定义也比较灵活。“对于一些组织来说,首次面对数百GB的数据可能就需要调整数据管理策略,而对于另一些组织来说,可能需要数十或数百TB的数据大小才需要这种改变。”[14]
定义
大数据这个词从20世纪90年代开始使用,一些人认为是约翰·马西 John Mashey推广了这个词。[15][16]大数据通常包括大小超出常用软件工具能力(在可承受的时间内捕获、管理和处理数据)的数据集。[17] 大数据包括非结构化、半结构化和结构化数据,但主要关注非结构化数据。[18]大数据的“规模”是一个比较灵活的衡量标准;从几十兆字节到许多兆字节的数据。[19]大数据需要一套具有新集成技术来处理多样化、复杂和大规模的数据集。[20]
一些组织增加了“多样性”、“准确性”和其他各种“V”开头的字母来描述它,但这一修订受到了一些行业权威的质疑。[21]大数据的V通常被称为三V、四V和V。它们代表了大数据的大数量、多样性、速度、准确性和价值(volume, variety, velocity, veracity, and value)。[3] 可变性通常被视为大数据的额外属性。
2018年的一项定义指出,“大数据技术是需要并行计算工具来处理数据的”,并指出,“这代表了通过并行编程理论使用的计算机科学发生了一个明显而清晰的变化,以及丧失了Codd的关系型数据库的一些保障和功能。”[22]
在一项大数据集的对比研究中,Kitchin和McArdle发现,在所有分析案例中,大数据的常见特征并不都一致。[23]因此,其他研究将知识发现中权力动力学的重新定义确定为知识发现的定义特征。[24]这种另类视角没有关注大数据的内在特征,而是推动了对对象的关系理解,声称重要的是数据的收集、存储、可用和分析方式。
大数据vs商业智能
这个概念的日益成熟更鲜明地描绘了“大数据”和“商业智能”之间的区别:
- 商业智能使用应用数学工具和描述性统计以及高信息密度的数据来衡量事物、检测趋势等。[25]
- 大数据使用数学分析、优化、归纳统计和非线性系统[26]识别从低信息密度的大数据集推断规律(回归、非线性关系和因果效应),以揭示关系和相关性,或对结果和行为进行预测。[27] to reveal relationships and dependencies, or to perform predictions of outcomes and behaviors.[26][28]
特征
大数据可以用以下特征来描述:
- 大数据量
生成和存储的数据量大小决定了价值和潜在分析价值,以及它是否可以被视为大数据。大数据的大小通常大于TB和PB。
- 多样性
数据的类型和性质。像RDBMS这样的早期技术能够高效地处理结构化数据。然而,从结构化到半结构化或非结构化的类型和性质的变化对现有的工具和技术提出了挑战。大数据技术的发展初衷是捕获、存储和处理以高速和大量的半结构化和非结构化数据。后来,这些工具和技术也被用于处理结构化数据,并且更适合用于存储。最终,结构化数据的处理仍然是可选的,可以使用大数据,也可以使用传统的RDBMS。这有助于分析数据,以有效利用通过社交媒体、日志文件、传感器等收集的数据中暴露出来的隐藏内容。大数据来自文本、图像、音频和视频;此外,它还通过数据融合完成缺失的部分。
- 高速度
数据生成和处理的速度。为满足增长的需求和挑战。大数据通常是实时可用的。与小数据相比,大数据的产生周期是持续的。与大数据相关的两种速度是生成频率和处理、记录和发布频率。
- 真实性
数据的真实性或可靠性,指数据质量和数据价值。大数据不仅必须是大数据,而且必须是可靠的,以便在分析中实现价值。捕获数据的数据质量可能会有很大差异,从而影响准确的分析。
- 价值性
通过处理和分析大型数据集可以获得的信息价值。价值也可以通过评估大数据的其他质量来衡量。价值也可能代表从大数据分析中获取的信息的盈利能力。
- 易变性
大数据的格式、结构或来源不断变化的特点。大数据可以包括结构化、非结构化或结构化与非结构化数据的组合。大数据分析可以整合来自多个来源的原始数据。原始数据的处理还可能涉及将非结构化数据转换为结构化数据。
大数据的其他可能特征包括:
- 详尽性
是否捕获或记录整个系统(即n=all)。大数据可能包括也可能不包括所有来源的可用数据。
- 细粒度和独特的词汇
每个元素的特定数据在每个元素中所占的比例,以及元素及其特征是否被正确索引或识别。
- 关系的
如果收集的数据包含公共字段,则可以对不同的数据集进行连接或元分析。
- 延展性
如果可以轻松添加或更改所收集数据的每个元素中的新字段。
- 可扩展性
如果大数据存储系统的规模能够迅速扩大。
数据结构
大数据存储库以多种形式存在,通常由有特殊需求的公司建立。从20世纪90年代开始,商业供应商就开始为大数据提供并行数据库管理系统。多年来,WinterCorp公司发布了最大的数据库报告。[29]
天睿(Teradata Corporation)于1984年推出了并行处理DBC 1012系统。1992年,Teradata系统是第一个存储和分析1TB数据的系统。1991年硬盘驱动器为2.5GB,因此大数据的定义不断演变。Teradata于2007年安装了第一个基于PB级RDBMS的系统,安装了几十个PB级的Teradata关系数据库,其中最大的超过50PB。直到2008年,系统都是100%结构化的关系数据。从那时起,Teradata添加了非结构化数据类型,包括XML、JSON和Avro。
2000年,Seisint公司(现为LexisNexis Risk Solutions)开发了一个基于C++的分布式数据处理和查询平台,称为HPCC系统平台。该系统可以跨多个商品服务器自动划分、分发、存储和交付结构化、半结构化和非结构化数据。用户可以用一种称为ECL的声明性数据流编程语言编写数据处理管道和查询。在ECL工作的数据分析师不需要预先定义数据模式,而是可以专注于手头的特定问题,并在开发解决方案时以最佳方式重塑数据。2004年,LexisNexis收购了Seisint公司及其高速并行处理平台,[30]并在2008年收购Choicepoint公司时成功利用该平台集成了该公司的数据系统。[31]《华盛顿邮报》2011年报道,HPCC 系统平台根据 Apache v2.0许可证开源。
欧洲核子研究中心(CERN)和其他物理实验几十年来一直在收集大数据集,这些数据集通常是通过高通量计算进行分析的,而不是当前“大数据”运动通常指的map-reduce架构。
2004年,谷歌发表了一篇关于MapReduce流程的论文,该流程使用了类似的架构。MapReduce概念提供了一个并行处理模型,并发布了一个相关的实现来处理大量数据。使用MapReduce,查询被拆分并分布在并行节点上,并进行并行处理(“映射”步骤)。然后收集并交付结果(“归约”步骤)。该框架非常成功,[32]所以其他人想复制这个算法。因此,一个名为“Hadoop”的Apache开源项目采用了MapReduce框架的实现。[33]ApacheSpark是在2012年针对MapReduce范例中的局限性开发的,因为它增加了设置许多操作的能力(而不仅仅是Map之后的Reduce)。
MIKE2.0是一个开放的信息管理方法,由于在一篇题为《大数据解决方案提供 Big Data Solution Offering》的文章中指出的大数据影响,该方法仍需要进行修订。[34]这种方法论通过数据源的有用排列、相互关系的复杂性以及删除(或修改)单个记录的难度等方面处理大数据。[35]
2012年的研究表明,多层架构是解决大数据带来的问题的一种途径。分布式并行体系结构将数据分布在多个服务器上;这些并行执行环境可以显著提高数据处理速度。这种类型的体系结构将数据插入到并行DBMS中,后者实现了MapReduce和Hadoop框架的使用。这种类型的框架希望通过使用前端应用服务器,使处理能力对最终用户透明。[36]
数据池允许组织将其重点从集中控制转移到共享模型,以响应信息管理不断变化的动态。这样可以将数据快速分离到数据池中,从而减少开销时间。[37][38]
技术
麦肯锡全球研究所 McKinsey Global Institute2011年的一份报告将大数据的主要组成部分和生态系统描述如下:
- 分析数据的技术,如A/B测试、机器学习和自然语言处理
- 大数据技术,如商业智能、云计算和数据库
- 可视化,如图表、图表和其他数据显示
多维大数据也可以表示为OLAP数据立方体或数学上的张量。阵列数据库系统已经支持这种数据类型的存储和高级查询。[39]应用于大数据的其他技术包括基于张量的高效计算,如多线性子空间学习、[40]大规模并行处理(MPP)数据库、基于搜索的应用、数据挖掘、[41]分布式文件系统、分布式缓存(如burst buffer和Memcached)、分布式数据库,基于云和HPC的基础设施(应用程序、存储和计算资源)[42]以及互联网。尽管已经开发了许多方法和技术,但使用大数据进行机器学习仍然很困难。[43]
一些MPP关系型数据库能够存储和管理PB级的数据。隐式是加载、监视、备份和优化RDBMS中大型数据表使用的能力。[44]
DARPA的拓扑数据分析项目寻求海量数据集的基本结构,2008年,这项技术随着一家名为“Ayasdi”的公司的成立而公之于众。[45]
大数据分析流程的从业者通常不喜欢速度较慢的共享存储,[46]他们更喜欢各种形式的直连存储(DAS),从固态驱动器(SSD)到加入并行处理节点中的高容量SATA磁盘。共享存储体系结构存储区域网络(SAN)和网络连接存储(NAS)的概念是它们相对缓慢、复杂且昂贵。这些品质与依赖系统性能、商品基础设施和低成本的大数据分析系统不一致。
实时或近实时信息交付是大数据分析的特征之一。因此,无论何时何地都可以避免延迟。直连内存或磁盘中的数据是良好的,而FC SAN连接另一端的内存或磁盘中的数据则不是。分析应用程序所需规模的SAN的成本比其他存储技术高得多。
应用
大数据极大地增加了对信息管理专家的需求,以至于Software AG、甲骨文、IBM、微软、SAP、EMC、惠普和戴尔在专门从事数据管理和分析的软件公司上花费了150多亿美元。2010年,这个行业的价值超过1000亿美元,并以每年近10%的速度增长:大约是整个软件行业的两倍。
发达经济体越来越多地使用数据密集型技术。全世界有46亿手机用户,有10亿到20亿人上网。从1990年到2005年,全世界有超过10亿人进入中产阶级,这意味着更多的人变得更有文化,进而导致了信息的增长。1986年,世界通过电信网络交换信息的有效容量为281 PB,1993年为471 PB,2000年为2.2 EB,2007年为65 EB。[9]据预测,到2014年,互联网流量将达到每年667 EB。据估计,全球存储信息的三分之一是字母数字文本和静态图像数据,[47] 这是大多数大数据应用最有用的格式。这也显示了尚未使用的(以视频和音频内容的形式)数据的潜力。
虽然许多供应商为大数据提供现成的产品,但如果公司有足够的技术能力,专家则会开发内部定制系统。[48]
政府
在政府流程中应用大数据可以提高成本、生产率和创新效率,[49] 但也并非没有缺陷。数据分析通常需要政府的多个部门(中央和地方)合作,创建新的流程以实现预期结果。国家安全局(NSA)是一个利用大数据的常见政府组织,它不断监控互联网的活动,寻找其系统可能发现的可疑或非法活动的潜在模式。
民事登记和人口动态统计局(CRVS)收集从出生到死亡的所有身份证明。CRV是政府大数据的来源。
国际发展
关于有效利用信息和通信技术促进发展(也称为“ICT4D”)的研究表明,大数据技术可以做出重要贡献,但也对国际发展提出了独特的挑战。[50][51]大数据分析的进步为改善关键发展领域的决策提供了高成本效益的机会,如医疗保健、就业、经济生产率、犯罪、安全、自然灾害和资源管理。[52][53][54]此外,用户生成的数据提供了闻所未闻的新机会。[55] 然而,发展中地区面临的长期挑战,如技术基础设施不足、经济和人力资源匮乏,加剧了对大数据的现有担忧,如隐私、不完善的方法和互操作性问题。[52] “大数据促进发展”[52]的挑战目前正朝着通过机器学习的方向发展,称为“人工智能促进发展”(AI4D)。[56]
效益
大数据促进发展的一个主要实际应用是“用数据战胜贫困”。[57]2015年,Blumenstock及其同事通过手机元数据预测贫困和财富,[58] and in 2016 Jean and colleagues combined satellite imagery and machine learning to predict poverty.[59]2016年Jean及其同事结合卫星图像和机器学习预测贫困。Hilbert及其同事利用数字跟踪数据研究拉丁美洲的劳动力市场和数字经济,[60][61]认为数字跟踪数据有以下几个好处:
- 领域覆盖范围:包括以前难以或无法衡量的领域。
- 地理覆盖范围:我们的国际来源提供了几乎所有国家的大量可比数据,包括许多通常不包括在国际清单中的小国。
- 详细程度:提供具有许多相关变量和新方面(如网络连接)的细粒度数据。
- 及时性:图表可以在收集后的几天内生成。
挑战
与此同时,使用数字痕迹数据而不是传统调查数据并不能消除在国际定量分析领域工作时所面临的传统挑战。优先顺序改变了,但是基本的挑战仍然没有改变。主要挑战包括:
- 代表性。虽然传统的发展统计主要关注随机调查样本的代表性,但数字跟踪数据绝不是随机样本。[62]
- 普遍性。虽然观测数据总是很好地代表了这个来源,但它只代表了它所代表的东西。虽然从一个平台的具体观察概括到更广泛的环境是很有诱惑力的,但这通常非常具有欺骗性。
- 整合协调。数字跟踪数据仍然需要指标的国际间整合协调。它增加了“数据融合”的挑战,即不同来源的整合协调。
- 数据过载。分析师和机构不习惯有效地处理大量变量,这是通过交互式仪表盘能有效地完成。从业仍者然缺乏一个标准的工作流程,使研究人员、用户和决策者能够高效、高效地执行任务。[60]
医疗
大数据分析通过提供个性化医疗和处方分析、临床风险干预和预测分析、减少废物和护理变异性、患者数据的自动外部和内部报告、标准化医疗术语和患者登记,大数据分析在医疗保健中得到了应用。[63][64][65][66]有些领域的改进比实际执行的更具雄心壮志。医疗保健系统内生成的数据水平并非微不足道。随着移动健康、电子健康和可穿戴技术的广泛应用,数据量将继续增加。这包括电子健康记录数据、成像数据、患者生成的数据、传感器数据和其他难以处理的数据。现在,这种环境更加需要关注数据和信息质量。[67]“大数据通常意味着‘脏数据’,数据不准确的比例随着数据量的增长而增加。”在大数据范围内进行人体检查是不可能的,卫生服务部门迫切需要智能工具来准确、可信地控制和处理丢失的信息。[68]虽然医疗保健领域的大量信息现在是电子化的,但它符合大数据的要求,因为大多数信息都是非结构化的,难以使用。[69]在医疗保健中使用大数据引发了重大的道德挑战,从个人权利、隐私和自主权的风险,到透明度和信任。[70]
在探索性生物医学研究方面,健康研究中的大数据尤其有希望,因为数据驱动的分析比假设驱动的研究进展更快。[71]然后,数据分析中的趋势可以在传统的、假设驱动的后续生物学研究和最终的临床研究中得到检验。
医疗领域中一个严重依赖大数据的子领域是医学中的计算机辅助诊断。[72]例如,对于癫痫监测,通常每天创建5到10GB的数据。[73]类似地,一张未压缩的乳房断层合成图像的平均数据量为450 MB。[74]这些只是计算机辅助诊断使用大数据的众多例子中的一小部分。因此,大数据被认为是计算机辅助诊断系统需要克服的七大关键挑战之一。[75]
教育
麦肯锡全球研究所发现,受过高等培训的数据专业人员和管理人员[76]的需求存在150万人的短缺,包括田纳西大学和加州大学伯克利分校在内的一些大学[77]已经建立了硕士课程来满足这一需求。私营培训班也开发了一些项目来满足这一需求,包括数据孵化器等免费项目或大会等付费项目。[78]在营销的特定领域,Wedel和Kannan强调的一个问题是,[79]营销有几个子领域(例如广告、促销、产品开发、品牌推广),它们都使用不同类型的数据。
媒体
为了理解媒体如何使用大数据,首先需要为媒体处理所使用的机制提供一些场景。尼克·库尔德利(Nick Couldry)和约瑟夫·图罗(Joseph Turow)曾建议,媒体和广告从业者在处理大数据时,应尽可能多地处理数百万个人的可操作信息点。该行业似乎正在摆脱使用特定媒体环境(如报纸、杂志或电视节目)的传统方式,转而利用技术在最佳时间、最佳地点接触目标人群,以吸引消费者。最终目的是提供或传达符合消费者心态的信息或内容(在统计学上)。例如,发布环境越来越多地定制消息(广告)和内容(文章),以吸引专门通过各种数据挖掘活动收集的消费者。[80]
- 以消费者为目标(针对营销人员的广告)。[81]
- 数据捕获。
- 数据新闻:出版商和记者使用大数据工具提供独特和创新的见解和信息图表。
英国公共服务电视广播公司第四频道是大数据和数据分析领域的领导者。[82]
保险
健康保险提供商正在收集有关社会“健康决定因素”的数据,如食品和电视消费、婚姻状况、服装尺寸和购买习惯,并根据这些数据预测健康成本,以便发现客户的健康问题。目前,这些预测是否可被用于定价还存在争议。[83]
物联网
大数据和物联网协同工作,从物联网设备提取的数据提供了设备间连接的映射。媒体行业、公司和政府已经使用这种映射来更准确地定位受众并提高媒体效率。物联网也越来越多地被用作收集感官数据的手段,这种感官数据已被用于医疗[84]、制造[85]和运输[86] 环境。
数字创新专家凯文·阿什顿 Kevin Ashton创造了这个词,[87]在这句话中定义了物联网:“如果我们有一台计算机,它知道所有关于事物的知识,使用它们在没有我们任何帮助的情况下收集的数据,我们将能够跟踪和计算所有东西,并大大减少浪费、损失和成本。我们将知道什么时候需要更换、修理或召回,以及它们是新鲜的还是过时的。”
信息技术
特别是自2015年以来,大数据作为一种帮助员工更高效地工作并简化信息技术收集和分发的工具,在企业运营中日益突出。利用大数据解决企业内部的IT和数据收集问题称为IT运营分析 IT operations analytics(ITOA)。[88]通过将大数据原理应用到机器智能和深度计算的概念中,IT部门可以预测潜在问题并加以预防。[88]ITOA企业提供系统管理平台,将数据仓库整合在一起,从整个系统而不是从孤立的数据包中产生见解。
案例研究
国家
中国
- 一体化联合作战平台 The Integrated Joint Operations Platform(IJOP)被政府用来监控人口,尤其是维吾尔族。[89]通过免费体检项目收集生物特征(包括DNA样本)。[90]
- 到2020年,中国计划根据所有公民的行为给他们个人“社会信用”评分。[91]目前正在中国多个城市试点的社会信用体系被认为是一种使用大数据分析技术的大规模监控。[92][93]
印度
- 为了赢得2014年印度大选,印度人民党尝试了大数据分析。[94]
- 印度政府使用多种技术来确定印度选民对政府行动的反应,以及对政策的看法。
以色列
- 通过GlucoMe的大数据解决方案创建了个性化的糖尿病治疗。[95]
英国
大数据在公共服务中的应用示例:
- 处方药数据:通过连接每种处方药的产地、地点和时间,研究单位能够检查任何给定药物的释放与英国全国卫生保健研究所 National Institute for Health and Care Excellence指南在全英范围内的调整之间存在的延迟。以往,新的或最新的药物需要一些时间才能渗透到普通患者身上。[96]
- 整合数据:地方当局将道路沙砾摊等服务的数据与为高危人群提供的服务(如轮上用餐)混合在一起。数据的连接使地方当局得以避免天气导致的延迟。[97]
美国
- 2012年,奥巴马政府宣布了大数据研发计划,以探索如何利用大数据解决政府面临的重要问题。[98]该计划由分布在六个部门的84个不同的大数据项目组成。[99]
- 大数据分析在奥巴马2012年成功连任竞选中发挥了重要作用。[100]
- 美国联邦政府拥有世界上最强大的十台超级计算机中的五台。[101][102]
- 犹他州数据中心由美国国家安全局建造。完成后,该设施将能够处理NSA通过互联网收集的大量信息。确切的存储空间数量不得而知,但最近的消息来源称,存储空间大约为几EB。[103][104][105]这对所收集数据的匿名性提出了安全担忧。
零售
- 沃尔玛每小时处理超过100万笔客户交易,这些交易被导入数据库,据估计包含超过2.5 PB(2560 TB)的数据,相当于美国国会图书馆所有书籍所包含信息的167倍。
- Windermere Real Estate利用近1亿名司机的位置信息,帮助新购房者确定一天中不同时间上下班的典型驾驶时间。[106]
- FICO卡检测系统保护世界各地的账户。[107]
科学
- 大型强子对撞机的实验有着大约1.5亿个传感器每秒传送4000万次数据。每秒有近6亿次碰撞。在过滤并避免记录超过99.99995%的流之后,[108]每秒有1000次感兴趣的碰撞。[109][110][111]
- 因此,仅使用不到0.001%的传感器流数据,所有四个LHC实验的数据流在复制前代表25 PB的年速率。复制后,这将变成近200 PB。
- 如果所有传感器数据都记录在LHC中,数据流将非常难以处理。在复制之前,数据流的年速率将超过1.5亿PB,即每天近500 EB。从长远来看,这个数字相当于每天500五百万(5×1020)字节,几乎是世界上所有其他数据源总和的200倍。
- 平方公里阵列(Square Kilometre Array)是一个由数千根天线组成的射电望远镜。预计将于2024年投入使用。这些天线的总容量预计为14 EB,每天存储1 PB。[112][113]它被认为是有史以来最雄心勃勃的科学项目之一。[114]
- 斯隆数字天空测量(SDSS)在2000年开始收集天文数据时,它在最初几周收集的数据比之前天文学史上收集的所有数据都多。SDS以每晚约200 GB的速度运行,已经积累了超过140 TB的信息。[115] 当SDSS的后继者大型天气观测望远镜在2020年上线时,其设计者预计它将每五天获取如此数量的数据。
- 解码人类基因组最初需要10年的时间;现在不到一天就可以实现。在过去十年中,DNA测序仪将测序成本除以10000,比摩尔定律预测的成本低100倍。[116]
- 美国国家航空航天局气候模拟中心(NCCS)在探索超级计算集群上存储了32 PB的气候观测和模拟数据。[117][118]
- 谷歌的DNAStack对来自世界各地的基因数据的DNA样本进行编译和组织,以识别疾病和其他医疗缺陷。这些快速而精确的计算消除了任何“摩擦点”,或是众多研究DNA的科学和生物学专家中可能出现的人为错误。DNAStack是谷歌基因组学的一部分,它允许科学家使用谷歌搜索服务器上的大量样本资源来规模化社会实验,这些实验通常需要数年的时间。[119][120]
- 23andMe的DNA数据库包含全世界100多万人的基因信息。[121]该公司探索在患者同意的情况下,将“匿名聚合基因数据”出售给其他研究人员和制药公司用于研究目的。[122][123][124][125][126]杜克大学(Duke University)心理学和神经科学教授艾哈迈德·哈里里(Ahmad Hariri)自2009年以来一直在使用23andMe进行研究。他表示,该公司新服务的最重要方面是,它使科学家可以进行基因研究,而且成本相对较低。[122]一项研究在23andMe的数据库中确定了15个与抑郁症相关的基因组位点,导致访问存储库的需求激增,23andMe在论文发表后的两周内提出了近20个访问抑郁症数据的请求。[127]
- 计算流体力学(CFD)和流体动力湍流研究产生了大量数据集。约翰·霍普金斯湍流数据库(JHTDB)包含超过350 TB的时空场,这些场来自各种湍流的直接数值模拟。使用下载平面模拟输出文件等传统方法很难共享此类数据。JHTDB中的数据可以使用“虚拟传感器”进行访问,其访问模式多种多样,从直接网络浏览器查询、通过在客户平台上执行的Matlab、Python、Fortran和C程序进行访问,到切断服务下载原始数据。这些数据已用于150多份科学出版物。
运动
大数据可以通过运动传感器来改善训练和了解竞争对手。还可以使用大数据分析预测比赛的赢家。[128]球员未来的表现也可以预测。因此,球员的价值和薪水是由整个赛季收集的数据决定的。[129]
在一级方程式赛车比赛中,装有数百个传感器的赛车会产生TB的数据。这些传感器收集从轮胎压力到燃油燃烧效率的数据点。[130]根据这些数据,工程师和数据分析师决定是否应该进行调整以赢得比赛。此外,使用大数据,比赛团队试图根据赛季收集的数据进行模拟,预测他们将提前完成比赛的时间。[131]
技术
- 易趣网使用两个7.5 PB和40PB的数据仓库,以及一个40PB的Hadoop集群来进行搜索、消费者推荐和商品销售。[132]
- 亚马逊每天处理数以百万计的后端操作,以及来自50多万第三方卖家的查询。保持亚马逊运行的核心技术是基于Linux的,他们拥有世界上三大Linux数据库,容量分别为7.8 TB、18.5 TB和24.7 TB。[133]
- 谷歌每月处理大约1000亿次搜索。[136]
COVID-19
在2019冠状病毒疾病流行期间,大数据被认为是减少疾病影响的一种方法。大数据的重要应用包括最小化病毒传播、病例识别和医疗发展。[137]
各国政府使用大数据追踪感染者,以最大限度地减少传播。早期采用者包括中国、台湾、韩国和以色列。[138][139][140]
研究活动
2014年3月,美国工程教育学会(American Society of Engineering Education)展示了大数据中的加密搜索和集群形成。麻省理工学院计算机科学和人工智能实验室的Gautam Siwach和UNH研究小组的Amir Esmailpour致力于解决大数据的挑战,他们研究了大数据的关键特征,如集群的形成及其相互关联。他们通过提供技术中的原始定义和实时示例,重点关注大数据的安全性,以及该术语在云接口以加密形式存在不同类型数据的方向。此外,他们还提出了一种识别编码技术的方法,以加快对加密文本的搜索,从而增强大数据的安全性。[141]
2012年3月,白宫宣布了一项国家“大数据倡议”,由六个联邦部门和机构组成,承诺向大数据研究项目投入2亿多美元。[142]
该举措包括一个国家科学基金会“计算远征”,该项目将在五年内向加州大学伯克利分校的 AMPLab 提供1000万美元的资助。[143] at the University of California, Berkeley.[144]AMPLab还从DARPA和十几家行业赞助商那里获得资金,并利用大数据解决从预测交通拥堵[145]到抗击癌症等一系列问题。[146]
白宫大数据倡议还包括能源部承诺在五年内提供2500万美元的资金,以建立由能源部劳伦斯·伯克利国家实验室领导的可扩展数据管理、分析和可视化(SDAV)研究所。[147]SDAV研究所旨在汇集六个国家实验室和七所大学的专业知识,开发新的工具,帮助科学家管理和可视化国防部超级计算机上的数据。
2012年5月,美国马萨诸塞州宣布了马萨诸塞州大数据计划,该计划由州政府和私营公司向各种研究机构提供资金。[148]麻省理工学院(Massachusetts Institute of Technology)在麻省理工学院计算机科学与人工智能实验室(MIT Computer Science and Artificial Intelligence Laboratory)设立了英特尔大数据科学技术中心(Intel Science and Technology Center for Big Data),该中心将政府、企业和机构的资金和研究工作结合起来。[149]
欧盟委员会正在通过其第七个框架计划为为期两年的大数据公私论坛提供资金,让公司、学者和其他利益相关者参与讨论大数据问题。该项目旨在确定一项研究和创新战略,以指导欧盟委员会在成功实施大数据经济方面的支持行动。该项目的成果将作为其下一个框架项目“地平线2020”的投入。[150]
2014年3月,英国政府宣布成立艾伦·图灵研究所(Alan Turing Institute),该研究所以计算机先驱和破译者的名字命名,将专注于收集和分析大型数据集的新方法。[151]
在滑铁卢大学斯特拉特福校园加拿大开放数据体验(CODE)启示日,与会者演示了如何使用数据可视化可以增加对大数据集的理解和吸引力,并向世界传达他们的故事。[152]
计算社会科学——任何人都可以使用谷歌和Twitter等大数据持有者提供的应用程序编程接口(API)进行社会和行为科学研究。[153]这些API通常是免费提供的。[153]Tobias Preis等人利用谷歌趋势数据证明,来自人均国内生产总值(GDP)较高国家的互联网用户搜索未来信息的可能性大于搜索过去信息的可能性。研究结果表明,在线行为与现实世界的经济指标之间可能存在联系。[154][155][156]这项研究的作者根据下一年(2011年)的搜索量与上一年(2009年)的搜索量之比来检查谷歌的查询日志,他们称之为“未来方向指数”。[157]他们将未来导向指数与每个国家的人均GDP进行了比较,发现谷歌用户查询更多关于未来的国家有更高GDP的强烈趋势。
Tobias Preis和他的同事Helen Susannah Moat和H.Eugene Stanley介绍了一种方法,使用基于谷歌趋势(Google Trends)提供的搜索量数据的交易策略,识别股市走势的在线前兆。他们在科学报告中对谷歌98个不同财务相关性的搜索量进行的分析表明,财务相关搜索量的增加往往先于金融市场的巨大损失。[158] Their analysis of Google search volume for 98 terms of varying financial relevance, published in Scientific Reports,[159] suggests that increases in search volume for financially relevant search terms tend to precede large losses in financial markets.[160][161][162][163][164][165][166]
大数据集带来了以前不存在的算法挑战。因此,一些人认为有必要从根本上改变处理方式。[167]
现代海量数据集(MMD)算法研讨会汇集了计算机科学家、统计学家、数学家和数据分析从业者,讨论大数据的算法挑战。[168]关于大数据,这样的量级概念是相对的。正如它所说,“如果说过去的数据有什么指导意义的话,那么今天的大数据在不久的将来很可能不会被认为是这样的。”[72]
大数据采样
关于大数据集的一个研究问题是,是否有必要查看完整的数据或者样本要足够好,以得出关于数据属性的某些结论。大数据这个名称本身包含一个与规模相关的术语,这是大数据的一个重要特征。但抽样可以从更大的数据集中选择正确的数据点,以估计整个人口的特征。在制造过程中,不同类型的感官数据(如声学、振动、压力、电流、电压和控制器数据)在短时间间隔内可用。要预测停机时间,可能不需要查看所有数据,仅一个样本就足够了。大数据可以按不同的数据点分类,如人口统计、心理、行为和交易数据。有了大量的数据,营销人员可以创建和使用更多定制的消费者群体,以实现更具战略性的目标。
在大数据采样算法方面已经有了一些成果。比如抽样 Twitter 数据的理论公式已被开发出。[169]
批评
针对大数据范式的批评有两种:一种是质疑方法本身,另一种是质疑目前的方法。对这种批评的一个形式是批判性数据研究领域。[170]
针对大数据范式的批评
“一个关键问题是,我们对导致大数据典型网络特征出现的潜在经验微观过程知之甚少。”[17] Snijders、Matzat和Reips在他们的评论中指出,通常对数学性质做出非常强烈的假设,这些假设可能根本无法反映微观过程上的真实情况。马克·格雷厄姆 Mark Graham批评了克里斯·安德森Chris Anderson关于大数据将意味着理论的终结的断言:[171]他特别关注大数据必须始终在其社会、经济和政治背景下进行语境化的概念。[172]尽管公司投入8位数甚至9位数的资金,从供应商和客户的信息流中获取洞察力,但只有不到40%的员工拥有足够成熟的技能。根据《哈佛商业评论》(Harvard Business Review)上的一篇文章,为了克服这种洞察力缺陷,大数据无论多么全面或分析得多么好,都必须辅之以“综合判断力”。[173]
与此大致相同的是,有人指出,基于大数据分析的决策不可避免地“像过去一样,或者充其量也像现在一样,受到世界的影响”。[52]如果未来与过去相似,通过大量关于过去经验的数据,算法可以预测未来的发展。如果系统对未来的动态变化(如果它不是一个平稳的过程),那么过去对未来的影响就很小。[174]为了在不断变化的环境中做出预测,有必要对系统动力学有一个透彻的了解。[174]作为对这一批评的回应,Alemany Oliver和Vayre建议使用“诱因推理作为研究过程的第一步,以便为消费者的数字痕迹提供背景,并使新的理论出现”。[175]此外,有人建议将大数据方法与计算机模拟相结合,例如基于代理的模型[52]和复杂系统。通过基于一系列相互依赖的算法的计算机模拟,基于代理的模型在预测甚至未知场景的社会复杂性的结果方面越来越好。[176][177]最后,探索数据潜在结构的多变量方法的使用,如因子分析和聚类分析,已被证明是有用的分析方法,远远超出了通常用于较小数据集的双变量方法。
在健康和生物学领域,传统的科学方法是基于实验的。对于这些方法,限制因素是相关的数据,可以证实或反驳最初的假设。[178]如今,生物科学界接受了一个新的假设:大量数据(组学)提供的信息(无需事先假设)是对基于实验的传统方法的补充,有时是必需的。[179][180]在大数据方法中,相关假设的表述是解释数据的限制因素。[181]搜索逻辑被颠倒以及归纳的局限性是需要考虑的。
隐私倡导者担心个人身份信息的存储和收集增加了对隐私的威胁;专家小组发布了各种政策建议,以使实践符合对隐私的期望。[182] 媒体、公司甚至政府在几起案件中滥用大数据,导致几乎所有支撑社会的基本机构都失去了信任。[183]
Nayef Al-Rodhan认为,在大数据和拥有大量信息的大公司的背景下,需要一种新的社会契约来保护个人自由,大数据的使用应该在国家和国际层面受到更好的监管。[184]Barocas和Nissenbaum认为,保护个人用户的一种方法是,让用户了解所收集的信息类型、与谁共享信息、在什么约束下以及出于什么目的。[185]
针对“ v”模型的批评
大数据的“V”模型令人担忧,因为它以计算的可延展性为中心,缺乏信息的可感知性和可理解性。这导致了认知大数据框架的形成,该框架根据以下特点描述了大数据应用:[186]
- 数据完整性:从数据中理解意义不明的信息。
- 数据相关性、因果关系和可预测性:因果关系不是实现可预测性的必要条件。
- 可解释性和可解释性:人类渴望理解并接受他们所理解的,而算法无法实现这一目标。
- 自动决策的水平:支持自动决策算法和自我学习算法。
对新颖性的批评
一个多世纪以来,计算机一直在分析大型数据集,包括IBM的穿孔卡片机进行的美国人口普查分析,该机器计算统计数据,包括整个大陆人口的均值和方差。近几十年来,欧洲核子研究中心(CERN)等科学实验产生的数据规模与当前的商业“大数据”类似。然而,科学实验倾向于使用专门定制的高性能计算(超级计算)集群和网格来分析数据,而不是像当前的商业应用那样使用廉价商品计算机云,这意味着文化和技术堆栈上的差异。
针对大数据执行的批评
Ulf Dietrich Reips和Uwe Matzat在2014年写道,大数据已经成为科学研究的“风潮”。[153]研究人员Danah Boyd对大数据在科学中的使用提出了担忧,因为研究往往忽略了一些原则,比如选择代表性样本时过于关注处理大量数据,[187]这种方法可能会导致结果在某种程度上存在偏差。[188]大量异构数据资源的集成(有些被认为是大数据,有些则不是)带来巨大的后勤和分析挑战,但许多研究人员认为,这种集成可能代表着科学领域最有前途的新前沿。[189]在这篇颇具煽动性的文章《大数据的关键问题》(Critical Questions for Big Data)中,[190]作者将大数据称为神话的一部分:“大数据集提供了更高形式的智能和知识……大数据的用户往往“迷失在庞大的数据量中”,而且“使用大数据仍然是主观的,它量化的东西不一定能够更接近客观事实”。[190]BI领域的最新发展,例如前瞻性报告,特别是通过自动过滤无用数据及相关性来改善大数据的可用性。[191]大数据充满了虚假的相关性,[192]要么是因为非因果巧合(真大数定律),要么是大随机数的唯一性[193] (拉姆齐理论)或其他未发现的因素,因此早期实验者建立大型数字数据库“用数据说话”以及宣称的革新科学方法都受到了质疑。[194]
与对较小数据集的分析相比,大数据分析往往是肤浅的。[195]在许多大数据项目中,没有进行大数据分析,但挑战在于提取、转换、加载和预处理数据的部分。[195]
大数据是一个时髦的“模糊术语”,[196][197]但同时也是企业家、咨询师、科学家和媒体的关注热点。[197]近年来,谷歌流感趋势(Google Flu Trends)等大数据应用在最近几年未能提供好的预测,将流感疫情高估了两倍。类似地,基于Twitter的奥斯卡奖和选举预测往往偏离目标。大数据往往与小数据面临同样的挑战;添加更多数据并不能解决偏见问题,甚至可能会强调其他问题。尤其是Twitter等数据源不能代表整体人口的意见,从这些数据源得出的结果可能会导致错误结论。基于文本大数据统计分析的谷歌翻译在翻译网页方面做得很好。然而,来自专门领域的结果可能会有很大的偏差。另一方面,大数据也可能带来新的问题,比如多重比较问题:同时测试大量假设可能会产生许多错误的结果,这些结果被错误地认为是重要的。Ioannidis认为,“大多数已发表的研究结果都是错误的”,[198]其原因基本上是相同的:当许多科学团队和研究人员各自进行许多实验(即处理大量科学数据;尽管没有使用大数据技术),一个“重大”结果被错误的可能性会迅速增加。而当只有正面的结果被公布时,这种可能性更大。
此外,大数据分析结果只能与预测模型一样好。例如,大数据参与了对2016年美国总统选举结果的预测,[199]并取得了与预测模型类似的不同程度的成功。
针对大数据监管和监视批评
大数据已被执法和企业等机构用于警务和监视。[200] 与传统的警务方法相比,基于数据的监控不那么明显,因此反对大数据警务的可能性较小。根据Sarah Brayne的《大数据监控:警务案例 Big Data Surveillance: The Case of Policing》,[201] 大数据警务会通过三种方式加剧现有的社会不平等:
- 通过使用一个数学的无偏算法,将嫌疑犯置于更严格的监视之下。
- 增加执法跟踪的范围和人数,并加剧刑事司法系统中存在的特定种族比例过高的现象。
- 鼓励社会成员放弃与产生数字痕迹的机构的互动,从而为社会包容制造障碍。
如果以上潜在的问题得不到纠正或监管,大数据监管的影响可能会继续影响社会分化。Brayne还指出,谨慎地使用大数据监管可以防止个人层面的偏见变成制度层面的偏见。
在流行文化中
书籍
- 《点球成金》(Moneyball)是一本非虚构的书,书中探讨了探讨奥克兰田径队如何利用统计分析来超越那些预算较大的球队。2011年,由布拉德·皮特主演的改编电影上映。
电影
- 在《美国队长:寒冬战士 Captain America: The Winter Soldier》中,H.Y.D.R.A (伪装成神盾局)开发了一种利用数据来确定和消除全球威胁的飞行母舰。
- 在《蝙蝠侠: 黑暗骑士 The Dark Knight》中,蝙蝠侠使用的声纳设备可以监视整个哥谭市,这些数据是通过市内居民的手机收集的。
参见
{{columns-list|colwidth=26em|
参考文献
- ↑ Hilbert, Martin; López, Priscila (2011). "The World's Technological Capacity to Store, Communicate, and Compute Information". Science. 332 (6025): 60–65. Bibcode:2011Sci...332...60H. doi:10.1126/science.1200970. PMID 21310967. S2CID 206531385. Retrieved 13 April 2016.
- ↑ Breur, Tom (July 2016). "Statistical Power Analysis and the contemporary "crisis" in social sciences". Journal of Marketing Analytics. London, England: Palgrave Macmillan. 4 (2–3): 61–65. doi:10.1057/s41270-016-0001-3. ISSN 2050-3318.
- ↑ 3.0 3.1 "The 5 V's of big data". Watson Health Perspectives (in English). 2016-09-17. Retrieved 2021-01-20.
- ↑ boyd, dana; Crawford, Kate (21 September 2011). "Six Provocations for Big Data". Social Science Research Network: A Decade in Internet Time: Symposium on the Dynamics of the Internet and Society. doi:10.2139/ssrn.1926431. S2CID 148610111.
- ↑ "Community cleverness required". Nature. 455 (7209): 1. September 2008. Bibcode:2008Natur.455....1.. doi:10.1038/455001a. PMID 18769385.
- ↑ Reichman OJ, Jones MB, Schildhauer MP (February 2011). "Challenges and opportunities of open data in ecology". Science. 331 (6018): 703–5. Bibcode:2011Sci...331..703R. doi:10.1126/science.1197962. PMID 21311007. S2CID 22686503.
- ↑ Hellerstein, Joe (9 November 2008). "Parallel Programming in the Age of Big Data". Gigaom Blog.
- ↑ Segaran, Toby; Hammerbacher, Jeff (2009). Beautiful Data: The Stories Behind Elegant Data Solutions. O'Reilly Media. p. 257. ISBN 978-0-596-15711-1. https://books.google.com/books?id=zxNglqU1FKgC.
- ↑ 9.0 9.1 Hilbert M, López P (April 2011). "The world's technological capacity to store, communicate, and compute information" (PDF). Science. 332 (6025): 60–5. Bibcode:2011Sci...332...60H. doi:10.1126/science.1200970. PMID 21310967. S2CID 206531385.
- ↑ "IBM What is big data? – Bringing big data to the enterprise". ibm.com. Retrieved 26 August 2013.
- ↑ Reinsel, David; Gantz, John; Rydning, John (13 April 2017). "Data Age 2025: The Evolution of Data to Life-Critical" (PDF). seagate.com. Framingham, MA, US: International Data Corporation. Retrieved 2 November 2017.
- ↑ Oracle and FSN, "Mastering Big Data: CFO Strategies to Transform Insight into Opportunity", December 2012
- ↑ Jacobs, A. (6 July 2009). "The Pathologies of Big Data". ACMQueue.
- ↑ Magoulas, Roger; Lorica, Ben (February 2009). "Introduction to Big Data". Release 2.0. Sebastopol CA: O'Reilly Media (11).
- ↑ John R. Mashey (25 April 1998). "Big Data ... and the Next Wave of InfraStress" (PDF). Slides from invited talk. Usenix. Retrieved 28 September 2016.
- ↑ Steve Lohr (1 February 2013). "The Origins of 'Big Data': An Etymological Detective Story". The New York Times. Retrieved 28 September 2016.
- ↑ 17.0 17.1 Snijders, C.; Matzat, U.; Reips, U.-D. (2012). "'Big Data': Big gaps of knowledge in the field of Internet". International Journal of Internet Science. 7: 1–5.
- ↑ Dedić, N.; Stanier, C. (2017). "Towards Differentiating Business Intelligence, Big Data, Data Analytics and Knowledge Discovery". Innovations in Enterprise Information Systems Management and Engineering. Lecture Notes in Business Information Processing. 285. Berlin ; Heidelberg: Springer International Publishing. pp. 114–122. doi:10.1007/978-3-319-58801-8_10. ISBN 978-3-319-58800-1. ISSN 1865-1356. OCLC 909580101. http://eprints.staffs.ac.uk/3551/1/Towards%20Differentiating%20Business%20Intelligence%20Big%20Data%20Data%20Analytics%20and%20Knowldge%20Discovery.docx.
- ↑ Everts, Sarah (2016). "Information Overload". Distillations. Vol. 2, no. 2. pp. 26–33. Retrieved 22 March 2018.
- ↑ Ibrahim; Targio Hashem, Abaker; Yaqoob, Ibrar; Badrul Anuar, Nor; Mokhtar, Salimah; Gani, Abdullah; Ullah Khan, Samee (2015). "big data" on cloud computing: Review and open research issues". Information Systems. 47: 98–115. doi:10.1016/j.is.2014.07.006.
- ↑ Grimes, Seth. "Big Data: Avoid 'Wanna V' Confusion". InformationWeek. Retrieved 5 January 2016.
- ↑ Fox, Charles (25 March 2018). Data Science for Transport. Springer Textbooks in Earth Sciences, Geography and Environment. Springer. ISBN 9783319729527. https://www.springer.com/us/book/9783319729527.
- ↑ Kitchin, Rob; McArdle, Gavin (2016). "What makes Big Data, Big Data? Exploring the ontological characteristics of 26 datasets". Big Data & Society. 3: 1–10. doi:10.1177/2053951716631130. S2CID 55539845.
- ↑ Balazka, Dominik; Rodighiero, Dario (2020). "Big Data and the Little Big Bang: An Epistemological (R)evolution". Frontiers in Big Data. 3: 31. doi:10.3389/fdata.2020.00031. hdl:1721.1/128865. PMC 7931920. PMID 33693404.
- ↑ "avec focalisation sur Big Data & Analytique" (PDF). Bigdataparis.com. Retrieved 8 October 2017.
- ↑ 26.0 26.1 Billings S.A. "Nonlinear System Identification: NARMAX Methods in the Time, Frequency, and Spatio-Temporal Domains". Wiley, 2013
- ↑ "le Blog ANDSI » DSI Big Data". Andsi.fr. Retrieved 8 October 2017.
- ↑ Les Echos (3 April 2013). "Les Echos – Big Data car Low-Density Data ? La faible densité en information comme facteur discriminant – Archives". Lesechos.fr. Retrieved 8 October 2017.
- ↑ "Survey: Biggest Databases Approach 30 Terabytes". Eweek.com. 8 November 2003. Retrieved 8 October 2017.
- ↑ "LexisNexis To Buy Seisint For $775 Million". The Washington Post. Retrieved 15 July 2004.
- ↑ The Washington Post
- ↑ Bertolucci, Jeff "Hadoop: From Experiment To Leading Big Data Platform", "Information Week", 2013. Retrieved on 14 November 2013.
- ↑ Webster, John. "MapReduce: Simplified Data Processing on Large Clusters", "Search Storage", 2004. Retrieved on 25 March 2013.
- ↑ "Big Data Solution Offering". MIKE2.0. Retrieved 8 December 2013.
- ↑ "Big Data Definition". MIKE2.0. Retrieved 9 March 2013.
- ↑ Boja, C; Pocovnicu, A; Bătăgan, L. (2012). "Distributed Parallel Architecture for Big Data". Informatica Economica. 16 (2): 116–127.
- ↑ "Solving Key Business Challenges With a Big Data Lake" (PDF). Hcltech.com. August 2014. Retrieved 8 October 2017.
- ↑ "Method for testing the fault tolerance of MapReduce frameworks" (PDF). Computer Networks. 2015.
- ↑ "Future Directions in Tensor-Based Computation and Modeling" (PDF). May 2009.
- ↑ Lu, Haiping; Plataniotis, K.N.; Venetsanopoulos, A.N. (2011). "A Survey of Multilinear Subspace Learning for Tensor Data" (PDF). Pattern Recognition. 44 (7): 1540–1551. Bibcode:2011PatRe..44.1540L. doi:10.1016/j.patcog.2011.01.004.
- ↑ Pllana, Sabri; Janciak, Ivan; Brezany, Peter; Wöhrer, Alexander (2016). "A Survey of the State of the Art in Data Mining and Integration Query Languages". 2011 14th International Conference on Network-Based Information Systems. IEEE Computer Society. pp. 341–348. arXiv:1603.01113. Bibcode 2016arXiv160301113P. doi:10.1109/NBiS.2011.58. ISBN 978-1-4577-0789-6.
- ↑ Wang, Yandong; Goldstone, Robin; Yu, Weikuan; Wang, Teng (October 2014). "Characterization and Optimization of Memory-Resident MapReduce on HPC Systems". 2014 IEEE 28th International Parallel and Distributed Processing Symposium. IEEE. pp. 799–808. doi:10.1109/IPDPS.2014.87. ISBN 978-1-4799-3800-1.
- ↑ L'Heureux, A.; Grolinger, K.; Elyamany, H. F.; Capretz, M. A. M. (2017). "Machine Learning With Big Data: Challenges and Approaches". IEEE Access. 5: 7776–7797. doi:10.1109/ACCESS.2017.2696365. ISSN 2169-3536.
- ↑ Monash, Curt (30 April 2009). "eBay's two enormous data warehouses".
Monash, Curt (6 October 2010). "eBay followup – Greenplum out, Teradata > 10 petabytes, Hadoop has some value, and more". - ↑ "Resources on how Topological Data Analysis is used to analyze big data". Ayasdi.
- ↑ CNET News (1 April 2011). "Storage area networks need not apply".
- ↑ Hilbert, Martin (2014). "What is the Content of the World's Technologically Mediated Information and Communication Capacity: How Much Text, Image, Audio, and Video?". The Information Society. 30 (2): 127–143. doi:10.1080/01972243.2013.873748. S2CID 45759014.
- ↑ Rajpurohit, Anmol (11 July 2014). "Interview: Amy Gershkoff, Director of Customer Analytics & Insights, eBay on How to Design Custom In-House BI Tools". KDnuggets. Retrieved 14 July 2014.
Generally, I find that off-the-shelf business intelligence tools do not meet the needs of clients who want to derive custom insights from their data. Therefore, for medium-to-large organizations with access to strong technical talent, I usually recommend building custom, in-house solutions.
- ↑ "The Government and big data: Use, problems and potential". Computerworld. 21 March 2012. Retrieved 12 September 2016.
- ↑ "White Paper: Big Data for Development: Opportunities & Challenges (2012) – United Nations Global Pulse". Unglobalpulse.org. Retrieved 13 April 2016.
- ↑ "WEF (World Economic Forum), & Vital Wave Consulting. (2012). Big Data, Big Impact: New Possibilities for International Development". World Economic Forum. Retrieved 24 August 2012.
- ↑ 52.0 52.1 52.2 52.3 52.4 Hilbert, M. (2016). Big Data for Development: A Review of Promises and Challenges. Development Policy Review, 34(1), 135–174. https://doi.org/10.1111/dpr.12142 free access: https://www.martinhilbert.net/big-data-for-development/
- ↑ "Elena Kvochko, Four Ways To talk About Big Data (Information Communication Technologies for Development Series)". worldbank.org. 4 December 2012. Retrieved 30 May 2012.
- ↑ "Daniele Medri: Big Data & Business: An on-going revolution". Statistics Views. 21 October 2013. Archived from the original on 17 June 2015. Retrieved 21 June 2015.
- ↑ Tobias Knobloch and Julia Manske (11 January 2016). "Responsible use of data". D+C, Development and Cooperation.
- ↑ Mann, S., & Hilbert, M. (2020). AI4D: Artificial Intelligence for Development. International Journal of Communication, 14(0), 21. https://www.martinhilbert.net/ai4d-artificial-intelligence-for-development/
- ↑ Blumenstock, J. E. (2016). Fighting poverty with data. Science, 353(6301), 753–754. https://doi.org/10.1126/science.aah5217
- ↑ Blumenstock, J., Cadamuro, G., & On, R. (2015). Predicting poverty and wealth from mobile phone metadata. Science, 350(6264), 1073–1076. https://doi.org/10.1126/science.aac4420
- ↑ Jean, N., Burke, M., Xie, M., Davis, W. M., Lobell, D. B., & Ermon, S. (2016). Combining satellite imagery and machine learning to predict poverty. Science, 353(6301), 790–794. https://doi.org/10.1126/science.aaf7894
- ↑ 60.0 60.1 Hilbert, M., & Lu, K. (2020). The online job market trace in Latin America and the Caribbean (UN ECLAC LC/TS.2020/83; p. 79). United Nations Economic Commission for Latin America and the Caribbean. https://www.cepal.org/en/publications/45892-online-job-market-trace-latin-america-and-caribbean
- ↑ UN ECLAC, (United Nations Economic Commission for Latin America and the Caribbean). (2020). Tracking the digital footprint in Latin America and the Caribbean: Lessons learned from using big data to assess the digital economy (Productive Development, Gender Affairs LC/TS.2020/12; Documentos de Proyecto). United Nations ECLAC. https://repositorio.cepal.org/handle/11362/45484
- ↑ Banerjee, Amitav; Chaudhury, Suprakash (2010). "Statistics without tears: Populations and samples". Industrial Psychiatry Journal. 19 (1): 60–65. doi:10.4103/0972-6748.77642. ISSN 0972-6748. PMC 3105563. PMID 21694795.
- ↑ Huser V, Cimino JJ (July 2016). "Impending Challenges for the Use of Big Data". International Journal of Radiation Oncology, Biology, Physics. 95 (3): 890–894. doi:10.1016/j.ijrobp.2015.10.060. PMC 4860172. PMID 26797535.
- ↑ Sejdic, Ervin; Falk, Tiago H. (4 July 2018). Signal Processing and Machine Learning for Biomedical Big Data.. Sejdić, Ervin, Falk, Tiago H.. [Place of publication not identified]. ISBN 9781351061216. OCLC 1044733829.
- ↑ Raghupathi W, Raghupathi V (December 2014). "Big data analytics in healthcare: promise and potential". Health Information Science and Systems. 2 (1): 3. doi:10.1186/2047-2501-2-3. PMC 4341817. PMID 25825667.
- ↑ Viceconti M, Hunter P, Hose R (July 2015). "Big data, big knowledge: big data for personalized healthcare" (PDF). IEEE Journal of Biomedical and Health Informatics. 19 (4): 1209–15. doi:10.1109/JBHI.2015.2406883. PMID 26218867. S2CID 14710821.
- ↑ O'Donoghue, John; Herbert, John (1 October 2012). "Data Management Within mHealth Environments: Patient Sensors, Mobile Devices, and Databases". Journal of Data and Information Quality. 4 (1): 5:1–5:20. doi:10.1145/2378016.2378021. S2CID 2318649.
- ↑ Mirkes EM, Coats TJ, Levesley J, Gorban AN (August 2016). "Handling missing data in large healthcare dataset: A case study of unknown trauma outcomes". Computers in Biology and Medicine. 75: 203–16. arXiv:1604.00627. Bibcode:2016arXiv160400627M. doi:10.1016/j.compbiomed.2016.06.004. PMID 27318570. S2CID 5874067.
- ↑ Murdoch TB, Detsky AS (April 2013). "The inevitable application of big data to health care". JAMA. 309 (13): 1351–2. doi:10.1001/jama.2013.393. PMID 23549579.
- ↑ Vayena E, Salathé M, Madoff LC, Brownstein JS (February 2015). "Ethical challenges of big data in public health". PLOS Computational Biology. 11 (2): e1003904. Bibcode:2015PLSCB..11E3904V. doi:10.1371/journal.pcbi.1003904. PMC 4321985. PMID 25664461.
- ↑ Copeland, CS (Jul–Aug 2017). "Data Driving Discovery" (PDF). Healthcare Journal of New Orleans: 22–27.
- ↑ 72.0 72.1 Yanase J, Triantaphyllou E (2019). "A Systematic Survey of Computer-Aided Diagnosis in Medicine: Past and Present Developments". Expert Systems with Applications. 138: 112821. doi:10.1016/j.eswa.2019.112821. S2CID 199019309.
- ↑ Dong X, Bahroos N, Sadhu E, Jackson T, Chukhman M, Johnson R, Boyd A, Hynes D (2013). "Leverage Hadoop framework for large scale clinical informatics applications". AMIA Joint Summits on Translational Science Proceedings. AMIA Joint Summits on Translational Science. 2013: 53. PMID 24303235.
- ↑ Clunie D (2013). "Breast tomosynthesis challenges digital imaging infrastructure".
{{cite journal}}
: Cite journal requires|journal=
(help) - ↑ Yanase J, Triantaphyllou E (2019). "The Seven Key Challenges for the Future of Computer-Aided Diagnosis in Medicine". International Journal of Medical Informatics. 129: 413–422. doi:10.1016/j.ijmedinf.2019.06.017. PMID 31445285. S2CID 198287435.
- ↑ 引用错误:无效
<ref>
标签;未给name属性为McKinsey
的引用提供文字 - ↑ "Degrees in Big Data: Fad or Fast Track to Career Success". Forbes. Retrieved 21 February 2016.
- ↑ "NY gets new boot camp for data scientists: It's free but harder to get into than Harvard". Venture Beat. Retrieved 21 February 2016.
- ↑ Wedel, Michel; Kannan, PK (2016). "Marketing Analytics for Data-Rich Environments". Journal of Marketing. 80 (6): 97–121. doi:10.1509/jm.15.0413. S2CID 168410284.
- ↑ Couldry, Nick; Turow, Joseph (2014). "Advertising, Big Data, and the Clearance of the Public Realm: Marketers' New Approaches to the Content Subsidy". International Journal of Communication. 8: 1710–1726.
- ↑ "Why Digital Advertising Agencies Suck at Acquisition and are in Dire Need of an AI Assisted Upgrade". Ishti.org. 15 April 2018. Archived from the original on 12 February 2019. Retrieved 15 April 2018.
- ↑ "Big data and analytics: C4 and Genius Digital". Ibc.org. Retrieved 8 October 2017.
- ↑ Marshall Allen (17 July 2018). "Health Insurers Are Vacuuming Up Details About You – And It Could Raise Your Rates". www.propublica.org. Retrieved 21 July 2018.
- ↑ "QuiO Named Innovation Champion of the Accenture HealthTech Innovation Challenge". Businesswire.com. 10 January 2017. Retrieved 8 October 2017.
- ↑ "A Software Platform for Operational Technology Innovation" (PDF). Predix.com. Retrieved 8 October 2017.
- ↑ Z. Jenipher Wang (March 2017). "Big Data Driven Smart Transportation: the Underlying Story of IoT Transformed Mobility".
- ↑ "That Internet Of Things Thing".
- ↑ 88.0 88.1 Solnik, Ray. "The Time Has Come: Analytics Delivers for IT Operations". Data Center Journal. Retrieved 21 June 2016.
- ↑ Josh Rogin (2 August 2018). "Ethnic cleansing makes a comeback – in China". No. Washington Post. Archived from the original on 31 March 2019. Retrieved 4 August 2018.
Add to that the unprecedented security and surveillance state in Xinjiang, which includes all-encompassing monitoring based on identity cards, checkpoints, facial recognition and the collection of DNA from millions of individuals. The authorities feed all this data into an artificial-intelligence machine that rates people's loyalty to the Communist Party in order to control every aspect of their lives.
- ↑ "China: Big Data Fuels Crackdown in Minority Region: Predictive Policing Program Flags Individuals for Investigations, Detentions". hrw.org. Human Rights Watch. 26 February 2018. Retrieved 4 August 2018.
- ↑ "Discipline and Punish: The Birth of China's Social-Credit System". The Nation. 23 January 2019.
- ↑ "China's behavior monitoring system bars some from travel, purchasing property". CBS News. 24 April 2018.
- ↑ "The complicated truth about China's social credit system". WIRED. 21 January 2019.
- ↑ "News: Live Mint". Are Indian companies making enough sense of Big Data?. Live Mint. 23 June 2014. Retrieved 22 November 2014.
- ↑ "Israeli startup uses big data, minimal hardware to treat diabetes". The Times of Israel. Retrieved 28 February 2018.
- ↑ Singh, Gurparkash, Duane Schulthess, Nigel Hughes, Bart Vannieuwenhuyse, and Dipak Kalra (2018). "Real world big data for clinical research and drug development". Drug Discovery Today. 23 (3): 652–660. doi:10.1016/j.drudis.2017.12.002. PMID 29294362.
{{cite journal}}
: CS1 maint: multiple names: authors list (link) - ↑ "Recent advances delivered by Mobile Cloud Computing and Internet of Things for Big Data applications: a survey". International Journal of Network Management. 11 March 2016. Retrieved 14 September 2016.
- ↑ Kalil, Tom (29 March 2012). "Big Data is a Big Deal". whitehouse.gov. Retrieved 26 September 2012 – via National Archives.
- ↑ Executive Office of the President (March 2012). "Big Data Across the Federal Government" (PDF). Office of Science and Technology Policy. Archived (PDF) from the original on 21 January 2017. Retrieved 26 September 2012 – via National Archives.
- ↑ Lampitt, Andrew (14 February 2013). "The real story of how big data analytics helped Obama win". InfoWorld. Retrieved 31 May 2014.
- ↑ "November 2018 | TOP500 Supercomputer Sites".
- ↑ Hoover, J. Nicholas. "Government's 10 Most Powerful Supercomputers". Information Week. UBM. Retrieved 26 September 2012.
- ↑ Bamford, James (15 March 2012). "The NSA Is Building the Country's Biggest Spy Center (Watch What You Say)". Wired. Retrieved 18 March 2013.
- ↑ "Groundbreaking Ceremony Held for $1.2 Billion Utah Data Center". National Security Agency Central Security Service. Archived from the original on 5 September 2013. Retrieved 18 March 2013.
- ↑ Hill, Kashmir. "Blueprints of NSA's Ridiculously Expensive Data Center in Utah Suggest It Holds Less Info Than Thought". Forbes. Retrieved 31 October 2013.
- ↑ Wingfield, Nick (12 March 2013). "Predicting Commutes More Accurately for Would-Be Home Buyers". The New York Times. Retrieved 21 July 2013.
- ↑ "FICO® Falcon® Fraud Manager". Fico.com. Retrieved 21 July 2013.
- ↑ Alexandru, Dan. "Prof" (PDF). cds.cern.ch. CERN. Retrieved 24 March 2015.
- ↑ "LHC Brochure, English version. A presentation of the largest and the most powerful particle accelerator in the world, the Large Hadron Collider (LHC), which started up in 2008. Its role, characteristics, technologies, etc. are explained for the general public". CERN-Brochure-2010-006-Eng. LHC Brochure, English version. CERN. Retrieved 20 January 2013.
- ↑ "LHC Guide, English version. A collection of facts and figures about the Large Hadron Collider (LHC) in the form of questions and answers". CERN-Brochure-2008-001-Eng. LHC Guide, English version. CERN. Retrieved 20 January 2013.
- ↑ Brumfiel, Geoff (19 January 2011). "High-energy physics: Down the petabyte highway". Nature. Vol. 469. pp. 282–83. Bibcode:2011Natur.469..282B. doi:10.1038/469282a.
- ↑ "IBM Research – Zurich" (PDF). Zurich.ibm.com. Retrieved 8 October 2017.
- ↑ "Future telescope array drives development of Exabyte processing". Ars Technica. 2 April 2012. Retrieved 15 April 2015.
- ↑ "Australia's bid for the Square Kilometre Array – an insider's perspective". The Conversation. 1 February 2012. Retrieved 27 September 2016.
- ↑ "Data, data everywhere". The Economist. 25 February 2010. Retrieved 9 December 2012.
- ↑ "Delort P., OECD ICCP Technology Foresight Forum, 2012" (PDF). Oecd.org. Retrieved 8 October 2017.
- ↑ "NASA – NASA Goddard Introduces the NASA Center for Climate Simulation". Nasa.gov. Retrieved 13 April 2016.
- ↑ Webster, Phil. "Supercomputing the Climate: NASA's Big Data Mission". CSC World. Computer Sciences Corporation. Archived from the original on 4 January 2013. Retrieved 18 January 2013.
- ↑ "These six great neuroscience ideas could make the leap from lab to market". The Globe and Mail. 20 November 2014. Retrieved 1 October 2016.
- ↑ "DNAstack tackles massive, complex DNA datasets with Google Genomics". Google Cloud Platform. Retrieved 1 October 2016.
- ↑ "23andMe – Ancestry". 23andme.com. Retrieved 29 December 2016.
- ↑ 122.0 122.1 Potenza, Alessandra (13 July 2016). "23andMe wants researchers to use its kits, in a bid to expand its collection of genetic data". The Verge. Retrieved 29 December 2016.
- ↑ "This Startup Will Sequence Your DNA, So You Can Contribute To Medical Research". Fast Company. 23 December 2016. Retrieved 29 December 2016.
- ↑ Seife, Charles. "23andMe Is Terrifying, but Not for the Reasons the FDA Thinks". Scientific American. Retrieved 29 December 2016.
- ↑ Zaleski, Andrew (22 June 2016). "This biotech start-up is betting your genes will yield the next wonder drug". CNBC. Retrieved 29 December 2016.
- ↑ Regalado, Antonio. "How 23andMe turned your DNA into a $1 billion drug discovery machine". MIT Technology Review. Retrieved 29 December 2016.
- ↑ "23andMe reports jump in requests for data in wake of Pfizer depression study | FierceBiotech". fiercebiotech.com. Retrieved 29 December 2016.
- ↑ Admire Moyo (23 October 2015). "Data scientists predict Springbok defeat". itweb.co.za. Retrieved 12 December 2015.
- ↑ Regina Pazvakavambwa (17 November 2015). "Predictive analytics, big data transform sports". itweb.co.za. Retrieved 12 December 2015.
- ↑ Dave Ryan (13 November 2015). "Sports: Where Big Data Finally Makes Sense". huffingtonpost.com. Retrieved 12 December 2015.
- ↑ Frank Bi. "How Formula One Teams Are Using Big Data To Get The Inside Edge". Forbes. Retrieved 12 December 2015.
- ↑ Tay, Liz. "Inside eBay's 90PB data warehouse". ITNews. Retrieved 12 February 2016.
- ↑ Layton, Julia (25 January 2006). "Amazon Technology". Money.howstuffworks.com. Retrieved 5 March 2013.
- ↑ "Scaling Facebook to 500 Million Users and Beyond". Facebook.com. Retrieved 21 July 2013.
- ↑ Constine, Josh (27 June 2017). "Facebook now has 2 billion monthly users… and responsibility". TechCrunch. Retrieved 3 September 2018.
- ↑ "Google Still Doing at Least 1 Trillion Searches Per Year". Search Engine Land. 16 January 2015. Retrieved 15 April 2015.
- ↑ Haleem, Abid; Javaid, Mohd; Khan, Ibrahim; Vaishya, Raju (2020). "Significant Applications of Big Data in COVID-19 Pandemic". Indian Journal of Orthopaedics. 54 (4): 526–528. doi:10.1007/s43465-020-00129-z. PMC 7204193. PMID 32382166.
- ↑ Manancourt, Vincent (10 March 2020). "Coronavirus tests Europe's resolve on privacy". Politico. Retrieved 30 October 2020.
- ↑ Choudhury, Amit Roy (27 March 2020). "Gov in the Time of Corona". Gov Insider. Retrieved 30 October 2020.
- ↑ Cellan-Jones, Rory (11 February 2020). "China launches coronavirus 'close contact detector' app". BBC. Archived from the original on 28 February 2020. Retrieved 30 October 2020.
- ↑ Siwach, Gautam; Esmailpour, Amir (March 2014). Encrypted Search & Cluster Formation in Big Data (PDF). ASEE 2014 Zone I Conference. University of Bridgeport, Bridgeport, Connecticut, US. Archived from the original (PDF) on 9 August 2014. Retrieved 26 July 2014.
- ↑ "Obama Administration Unveils "Big Data" Initiative:Announces $200 Million in New R&D Investments" (PDF). Office of Science and Technology Policy. Archived (PDF) from the original on 21 January 2017 – via National Archives.
- ↑ "AMPLab at the University of California, Berkeley". Amplab.cs.berkeley.edu. Retrieved 5 March 2013.
- ↑ "NSF Leads Federal Efforts in Big Data". National Science Foundation (NSF). 29 March 2012.
- ↑ Timothy Hunter; Teodor Moldovan; Matei Zaharia; Justin Ma; Michael Franklin; Pieter Abbeel; Alexandre Bayen (October 2011). Scaling the Mobile Millennium System in the Cloud.
- ↑ David Patterson (5 December 2011). "Computer Scientists May Have What It Takes to Help Cure Cancer". The New York Times.
- ↑ "Secretary Chu Announces New Institute to Help Scientists Improve Massive Data Set Research on DOE Supercomputers". energy.gov.
- ↑ Young, Shannon (2012-05-30). "Mass. governor, MIT announce big data initiative". Boston.com. Retrieved 2021-07-29.
- ↑ "Big Data @ CSAIL". Bigdata.csail.mit.edu. 22 February 2013. Retrieved 5 March 2013.
- ↑ "Big Data Public Private Forum". cordis.europa.eu. 1 September 2012. Retrieved 16 March 2020.
- ↑ "Alan Turing Institute to be set up to research big data". BBC News. 19 March 2014. Retrieved 19 March 2014.
- ↑ "Inspiration day at University of Waterloo, Stratford Campus". betakit.com/. Retrieved 28 February 2014.
- ↑ 153.0 153.1 153.2 Reips, Ulf-Dietrich; Matzat, Uwe (2014). "Mining "Big Data" using Big Data Services". International Journal of Internet Science. 1 (1): 1–8.
- ↑ Preis T, Moat HS, Stanley HE, Bishop SR (2012). "Quantifying the advantage of looking forward". Scientific Reports. 2: 350. Bibcode:2012NatSR...2E.350P. doi:10.1038/srep00350. PMC 3320057. PMID 22482034.
- ↑ Marks, Paul (5 April 2012). "Online searches for future linked to economic success". New Scientist. Retrieved 9 April 2012.
- ↑ Johnston, Casey (6 April 2012). "Google Trends reveals clues about the mentality of richer nations". Ars Technica. Retrieved 9 April 2012.
- ↑ Tobias Preis (24 May 2012). "Supplementary Information: The Future Orientation Index is available for download" (PDF). Retrieved 24 May 2012.
- ↑ Philip Ball (26 April 2013). "Counting Google searches predicts market movements". Nature. doi:10.1038/nature.2013.12879. S2CID 167357427. Retrieved 9 August 2013.
- ↑ Preis T, Moat HS, Stanley HE (2013). "Quantifying trading behavior in financial markets using Google Trends". Scientific Reports. 3: 1684. Bibcode:2013NatSR...3E1684P. doi:10.1038/srep01684. PMC 3635219. PMID 23619126.
- ↑ Nick Bilton (26 April 2013). "Google Search Terms Can Predict Stock Market, Study Finds". The New York Times. Retrieved 9 August 2013.
- ↑ Christopher Matthews (26 April 2013). "Trouble With Your Investment Portfolio? Google It!". Time. Retrieved 9 August 2013.
- ↑ Philip Ball (26 April 2013). "Counting Google searches predicts market movements". Nature. doi:10.1038/nature.2013.12879. S2CID 167357427. Retrieved 9 August 2013.
- ↑ Bernhard Warner (25 April 2013). "'Big Data' Researchers Turn to Google to Beat the Markets". Bloomberg Businessweek. Retrieved 9 August 2013.
- ↑ Hamish McRae (28 April 2013). "Hamish McRae: Need a valuable handle on investor sentiment? Google it". The Independent. London. Retrieved 9 August 2013.
- ↑ Richard Waters (25 April 2013). "Google search proves to be new word in stock market prediction". Financial Times. Retrieved 9 August 2013.
- ↑ Jason Palmer (25 April 2013). "Google searches predict market moves". BBC. Retrieved 9 August 2013.
- ↑ E. Sejdić (March 2014). "Adapt current tools for use with big data". Nature. 507 (7492): 306.
- ↑ Stanford. "MMDS. Workshop on Algorithms for Modern Massive Data Sets".
- ↑ Deepan Palguna; Vikas Joshi; Venkatesan Chakravarthy; Ravi Kothari & L. V. Subramaniam (2015). Analysis of Sampling Algorithms for Twitter. International Joint Conference on Artificial Intelligence.
- ↑ 引用错误:无效
<ref>
标签;未给name属性为Kimble and Milolidakis (2015)
的引用提供文字 - ↑ Chris Anderson (23 June 2008). "The End of Theory: The Data Deluge Makes the Scientific Method Obsolete". Wired.
- ↑ Graham M. (9 March 2012). "Big data and the end of theory?". The Guardian. London.
- ↑ Shah, Shvetank; Horne, Andrew; Capellá, Jaime (April 2012). "Good Data Won't Guarantee Good Decisions". Harvard Business Review. Retrieved 8 September 2012.
- ↑ 174.0 174.1 Big Data requires Big Visions for Big Change., Hilbert, M. (2014). London: TEDx UCL, x=independently organized TED talks
- ↑ Alemany Oliver, Mathieu; Vayre, Jean-Sebastien (2015). "Big Data and the Future of Knowledge Production in Marketing Research: Ethics, Digital Traces, and Abductive Reasoning". Journal of Marketing Analytics. 3 (1): 5–13. doi:10.1057/jma.2015.1. S2CID 111360835.
- ↑ Jonathan Rauch (1 April 2002). "Seeing Around Corners". The Atlantic.
- ↑ Epstein, J. M., & Axtell, R. L. (1996). Growing Artificial Societies: Social Science from the Bottom Up. A Bradford Book.
- ↑ "Delort P., Big data in Biosciences, Big Data Paris, 2012" (PDF). Bigdataparis.com. Retrieved 8 October 2017.
- ↑ "Next-generation genomics: an integrative approach" (PDF). nature. July 2010. Retrieved 18 October 2016.
- ↑ "Big Data in Biosciences". October 2015. Retrieved 18 October 2016.
- ↑ "Big data: are we making a big mistake?". Financial Times. 28 March 2014. Retrieved 20 October 2016.
- ↑ Ohm, Paul (23 August 2012). "Don't Build a Database of Ruin". Harvard Business Review.
- ↑ Bond-Graham, Darwin (2018). "The Perspective on Big Data". The Perspective.
- ↑ Al-Rodhan, Nayef (16 September 2014). "The Social Contract 2.0: Big Data and the Need to Guarantee Privacy and Civil Liberties – Harvard International Review". Harvard International Review. Archived from the original on 13 April 2017. Retrieved 3 April 2017.
- ↑ Barocas, Solon; Nissenbaum, Helen; Lane, Julia; Stodden, Victoria; Bender, Stefan; Nissenbaum, Helen (June 2014). Big Data's End Run around Anonymity and Consent. Cambridge University Press. pp. 44–75. doi:10.1017/cbo9781107590205.004. ISBN 9781107067356.
- ↑ Lugmayr, Artur; Stockleben, Bjoern; Scheib, Christoph; Mailaparampil, Mathew; Mesia, Noora; Ranta, Hannu; Lab, Emmi (1 June 2016). "A Comprehensive Survey On Big-Data Research and Its Implications – What is Really 'New' in Big Data? – It's Cognitive Big Data!".
{{cite journal}}
: Cite journal requires|journal=
(help) - ↑ danah boyd (29 April 2010). "Privacy and Publicity in the Context of Big Data". WWW 2010 conference. Retrieved 18 April 2011.
- ↑ Katyal, Sonia K. (2019). "Artificial Intelligence, Advertising, and Disinformation". Advertising & Society Quarterly (in English). 20 (4). doi:10.1353/asr.2019.0026. ISSN 2475-1790. S2CID 213397212.
- ↑ Jones, MB; Schildhauer, MP; Reichman, OJ; Bowers, S (2006). "The New Bioinformatics: Integrating Ecological Data from the Gene to the Biosphere" (PDF). Annual Review of Ecology, Evolution, and Systematics. 37 (1): 519–544. doi:10.1146/annurev.ecolsys.37.091305.110031.
- ↑ 190.0 190.1 Boyd, D.; Crawford, K. (2012). "Critical Questions for Big Data". Information, Communication & Society. 15 (5): 662–679. doi:10.1080/1369118X.2012.678878. hdl:10983/1320. S2CID 51843165.
- ↑ Failure to Launch: From Big Data to Big Decisions Forte Wares.
- ↑ "15 Insane Things That Correlate with Each Other".
- ↑ Random structures & algorithms
- ↑ Cristian S. Calude, Giuseppe Longo, (2016), The Deluge of Spurious Correlations in Big Data, Foundations of Science
- ↑ 195.0 195.1 Gregory Piatetsky (12 August 2014). "Interview: Michael Berthold, KNIME Founder, on Research, Creativity, Big Data, and Privacy, Part 2". KDnuggets. Retrieved 13 August 2014.
- ↑ Pelt, Mason (26 October 2015). ""Big Data" is an over used buzzword and this Twitter bot proves it". Siliconangle. Retrieved 4 November 2015.
- ↑ 197.0 197.1 Harford, Tim (28 March 2014). "Big data: are we making a big mistake?". Financial Times. Retrieved 7 April 2014.
- ↑ Ioannidis JP (August 2005). "Why most published research findings are false". PLOS Medicine. 2 (8): e124. doi:10.1371/journal.pmed.0020124. PMC 1182327. PMID 16060722.
- ↑ Lohr, Steve; Singer, Natasha (10 November 2016). "How Data Failed Us in Calling an Election". The New York Times. ISSN 0362-4331. Retrieved 27 November 2016.
- ↑ "How data-driven policing threatens human freedom". The Economist. 4 June 2018. ISSN 0013-0613. Retrieved 27 October 2019.
- ↑ Brayne, Sarah (29 August 2017). "Big Data Surveillance: The Case of Policing". American Sociological Review (in English). 82 (5): 977–1008. doi:10.1177/0003122417725865. S2CID 3609838.
拓展材料
- Peter Kinnaird; Inbal Talgam-Cohen, eds. (2012). "Big Data". XRDS: Crossroads, The ACM Magazine for Students. Vol. 19, no. 1. Association for Computing Machinery. ISSN 1528-4980. OCLC 779657714.
- Jure Leskovec; Anand Rajaraman; Jeffrey D. Ullman (2014). Mining of massive datasets. Cambridge University Press. ISBN 9781107077232. OCLC 888463433. http://mmds.org/.
- Viktor Mayer-Schönberger; Kenneth Cukier (2013). Big Data: A Revolution that Will Transform how We Live, Work, and Think. Houghton Mifflin Harcourt. ISBN 9781299903029. OCLC 828620988.
- Press, Gil (9 May 2013). "A Very Short History of Big Data". forbes.com. Jersey City, NJ. Retrieved 17 September 2016.
- Stephens-Davidowitz, Seth (2017). Everybody Lies: Big Data, New Data, and What the Internet Can Tell Us About Who We Really Are. Dey Street Books. ISBN 978-0062390851.
- "Big Data: The Management Revolution". Harvard Business Review. October 2012.
- O'Neil, Cathy (2017). Weapons of Math Destruction: How Big Data Increases Inequality and Threatens Democracy. Broadway Books. ISBN 978-0553418835.