“大规模脑网络”的版本间的差异
第19行: | 第19行: | ||
Large-scale brain networks are identified by their function and provide a coherent framework for understanding cognition by offering a neural model of how different cognitive functions emerge when different sets of brain regions join together as self-organized coalitions. The number and composition of the coalitions will vary with the algorithm and parameters used to identify them. In one model, there is only the default mode network and the task-positive network, but most current analyses show several networks, from a small handful to 17. The most common and stable networks are enumerated below. The regions participating in a functional network may be dynamically reconfigured. | Large-scale brain networks are identified by their function and provide a coherent framework for understanding cognition by offering a neural model of how different cognitive functions emerge when different sets of brain regions join together as self-organized coalitions. The number and composition of the coalitions will vary with the algorithm and parameters used to identify them. In one model, there is only the default mode network and the task-positive network, but most current analyses show several networks, from a small handful to 17. The most common and stable networks are enumerated below. The regions participating in a functional network may be dynamically reconfigured. | ||
− | 大规模脑网络是通过其功能来进行识别的。通过研究大规模脑网络建立神经模型,对不同脑区组合所形成的自组织联合体如何实现不同的'''<font color="#ff8000">认知Cognition</font>''' | + | 大规模脑网络是通过其功能来进行识别的。通过研究大规模脑网络建立神经模型,对不同脑区组合所形成的自组织联合体如何实现不同的'''<font color="#ff8000">认知Cognition</font>'''功能进行解释,就能够为认知理解提供一个连贯的框架。识别算法和参数的不同会导致所识别出的上述联合体的数量和组成有所不同。一个模型理论认为,符合上述条件的神经模型只包含'''<font color="#ff8000">默认模式网络Default mode network</font>'''和'''<font color="#ff8000">任务激活网络Task-positive network</font>''',但目前'''<font color="#32CD32">大多数分析理论都包括从几个到17个不等的网络。</font>'''下面列举了最常见且稳定的网络。'''<font color="#32CD32"> 人脑</font>'''可以动态地重新配置参与功能网络的脑区。 |
Disruptions in activity in various networks have been implicated in neuropsychiatric disorders such as [[Depression (mood)|depression]], [[Alzheimer's disease|Alzheimer's]], [[Autism-spectrum disorder|autism spectrum disorder]], [[schizophrenia]], [[ADHD]]<ref>{{cite journal |last1=Griffiths |first1=Kristi R. |last2=Braund |first2=Taylor A. |last3=Kohn |first3=Michael R. |last4=Clarke |first4=Simon |last5=Williams |first5=Leanne M. |last6=Korgaonkar |first6=Mayuresh S. |title=Structural brain network topology underpinning ADHD and response to methylphenidate treatment |journal=Translational Psychiatry |date=2 March 2021 |volume=11 |issue=1 |pages=1–9 |doi=10.1038/s41398-021-01278-x | pmc=7925571 |pmid=33654073 |url=https://www.nature.com/articles/s41398-021-01278-x#citeas |access-date=16 November 2021}}</ref> and [[bipolar disorder]].<ref>{{Cite journal|url=https://www.researchgate.net/publication/51639686|title=Large-scale brain networks and psychopathology: A unifying triple network model|last=Menon|first=Vinod|s2cid=26653572|journal=Trends in Cognitive Sciences|date=2011-09-09|volume=15|issue=10|pages=483–506|doi=10.1016/j.tics.2011.08.003|pmid=21908230}}</ref> | Disruptions in activity in various networks have been implicated in neuropsychiatric disorders such as [[Depression (mood)|depression]], [[Alzheimer's disease|Alzheimer's]], [[Autism-spectrum disorder|autism spectrum disorder]], [[schizophrenia]], [[ADHD]]<ref>{{cite journal |last1=Griffiths |first1=Kristi R. |last2=Braund |first2=Taylor A. |last3=Kohn |first3=Michael R. |last4=Clarke |first4=Simon |last5=Williams |first5=Leanne M. |last6=Korgaonkar |first6=Mayuresh S. |title=Structural brain network topology underpinning ADHD and response to methylphenidate treatment |journal=Translational Psychiatry |date=2 March 2021 |volume=11 |issue=1 |pages=1–9 |doi=10.1038/s41398-021-01278-x | pmc=7925571 |pmid=33654073 |url=https://www.nature.com/articles/s41398-021-01278-x#citeas |access-date=16 November 2021}}</ref> and [[bipolar disorder]].<ref>{{Cite journal|url=https://www.researchgate.net/publication/51639686|title=Large-scale brain networks and psychopathology: A unifying triple network model|last=Menon|first=Vinod|s2cid=26653572|journal=Trends in Cognitive Sciences|date=2011-09-09|volume=15|issue=10|pages=483–506|doi=10.1016/j.tics.2011.08.003|pmid=21908230}}</ref> | ||
第25行: | 第25行: | ||
Disruptions in activity in various networks have been implicated in neuropsychiatric disorders such as depression, Alzheimer's, autism spectrum disorder, schizophrenia, ADHD and bipolar disorder. | Disruptions in activity in various networks have been implicated in neuropsychiatric disorders such as depression, Alzheimer's, autism spectrum disorder, schizophrenia, ADHD and bipolar disorder. | ||
− | + | 脑网络活动的中断与诸多神经精神疾病密切相关,如'''<font color="#ff8000">抑郁症Depression</font>'''、'''<font color="#ff8000">老年痴呆症Alzheimer's</font>'''、'''<font color="#ff8000">自闭症谱系障碍Autism spectrum disorder</font>'''、'''<font color="#ff8000">精神分裂症Schizophrenia</font>'''、'''<font color="#ff8000">多动症ADHD</font>'''和'''<font color="#ff8000">躁郁症Bipolar disorder</font>'''。 | |
− | ==Core networks== | + | == Core networks== |
[[File:Heine2012x3010.png|thumb|An example that identified 10 large-scale brain networks from [[resting state fMRI]] activity through [[independent component analysis]].<ref name="Heine" />|链接=Special:FilePath/Heine2012x3010.png]] | [[File:Heine2012x3010.png|thumb|An example that identified 10 large-scale brain networks from [[resting state fMRI]] activity through [[independent component analysis]].<ref name="Heine" />|链接=Special:FilePath/Heine2012x3010.png]] | ||
Because brain networks can be identified at various different resolutions and with various different neurobiological properties, there is no such thing as a universal atlas of brain networks that fits all circumstances.<ref>{{cite journal|last1=Eickhoff|first1=SB|last2=Yeo|first2=BTT|last3=Genon|first3=S|title=Imaging-based parcellations of the human brain.|journal=Nature Reviews. Neuroscience|date=November 2018|volume=19|issue=11|pages=672–686|doi=10.1038/s41583-018-0071-7|pmid=30305712|s2cid=52954265|url=http://juser.fz-juelich.de/record/856633/files/Eickhoff_Yeo_Genon_NRN_MainManuscriptInclFigures.pdf}}</ref> While acknowledging this problem, Uddin, Yeo, and Spreng proposed in 2019<ref name="Uddin2019">{{cite journal|last1=Uddin|first1=LQ|last2=Yeo|first2=BTT|last3=Spreng|first3=RN|title=Towards a Universal Taxonomy of Macro-scale Functional Human Brain Networks.|journal=Brain Topography|date=November 2019|volume=32|issue=6|pages=926–942|doi=10.1007/s10548-019-00744-6|pmid=31707621|pmc=7325607}}</ref> that the following six networks should be defined as core networks based on converging evidences from multiple studies<ref>{{cite journal|last1=Doucet|first1=GE|last2=Lee|first2=WH|last3=Frangou|first3=S|title=Evaluation of the spatial variability in the major resting-state networks across human brain functional atlases.|journal=Human Brain Mapping|date=2019-10-15|volume=40|issue=15|pages=4577–4587|doi=10.1002/hbm.24722|pmid=31322303|pmc=6771873}}</ref><ref name="Yeo" /><ref>{{cite journal|last1=Smith|first1=SM|last2=Fox|first2=PT|last3=Miller|first3=KL|last4=Glahn|first4=DC|last5=Fox|first5=PM|last6=Mackay|first6=CE|last7=Filippini|first7=N|last8=Watkins|first8=KE|last9=Toro|first9=R|last10=Laird|first10=AR|last11=Beckmann|first11=CF|title=Correspondence of the brain's functional architecture during activation and rest.|journal=Proceedings of the National Academy of Sciences of the United States of America|date=2009-08-04|volume=106|issue=31|pages=13040–5|doi=10.1073/pnas.0905267106|pmid=19620724|pmc=2722273|bibcode=2009PNAS..10613040S|doi-access=free}}</ref> to facilitate communication between researchers. | Because brain networks can be identified at various different resolutions and with various different neurobiological properties, there is no such thing as a universal atlas of brain networks that fits all circumstances.<ref>{{cite journal|last1=Eickhoff|first1=SB|last2=Yeo|first2=BTT|last3=Genon|first3=S|title=Imaging-based parcellations of the human brain.|journal=Nature Reviews. Neuroscience|date=November 2018|volume=19|issue=11|pages=672–686|doi=10.1038/s41583-018-0071-7|pmid=30305712|s2cid=52954265|url=http://juser.fz-juelich.de/record/856633/files/Eickhoff_Yeo_Genon_NRN_MainManuscriptInclFigures.pdf}}</ref> While acknowledging this problem, Uddin, Yeo, and Spreng proposed in 2019<ref name="Uddin2019">{{cite journal|last1=Uddin|first1=LQ|last2=Yeo|first2=BTT|last3=Spreng|first3=RN|title=Towards a Universal Taxonomy of Macro-scale Functional Human Brain Networks.|journal=Brain Topography|date=November 2019|volume=32|issue=6|pages=926–942|doi=10.1007/s10548-019-00744-6|pmid=31707621|pmc=7325607}}</ref> that the following six networks should be defined as core networks based on converging evidences from multiple studies<ref>{{cite journal|last1=Doucet|first1=GE|last2=Lee|first2=WH|last3=Frangou|first3=S|title=Evaluation of the spatial variability in the major resting-state networks across human brain functional atlases.|journal=Human Brain Mapping|date=2019-10-15|volume=40|issue=15|pages=4577–4587|doi=10.1002/hbm.24722|pmid=31322303|pmc=6771873}}</ref><ref name="Yeo" /><ref>{{cite journal|last1=Smith|first1=SM|last2=Fox|first2=PT|last3=Miller|first3=KL|last4=Glahn|first4=DC|last5=Fox|first5=PM|last6=Mackay|first6=CE|last7=Filippini|first7=N|last8=Watkins|first8=KE|last9=Toro|first9=R|last10=Laird|first10=AR|last11=Beckmann|first11=CF|title=Correspondence of the brain's functional architecture during activation and rest.|journal=Proceedings of the National Academy of Sciences of the United States of America|date=2009-08-04|volume=106|issue=31|pages=13040–5|doi=10.1073/pnas.0905267106|pmid=19620724|pmc=2722273|bibcode=2009PNAS..10613040S|doi-access=free}}</ref> to facilitate communication between researchers. | ||
第42行: | 第42行: | ||
− | * The default mode network is active when an individual is awake and at rest. It preferentially activates when individuals focus on internally-oriented tasks such as daydreaming, envisioning the future, retrieving memories, and theory of mind. It is negatively correlated with brain systems that focus on external visual signals. It is the most widely researched network. | + | *The default mode network is active when an individual is awake and at rest. It preferentially activates when individuals focus on internally-oriented tasks such as daydreaming, envisioning the future, retrieving memories, and theory of mind. It is negatively correlated with brain systems that focus on external visual signals. It is the most widely researched network. |
第54行: | 第54行: | ||
− | * The salience network consists of several structures, including the anterior (bilateral) insula, dorsal anterior cingulate cortex, and three subcortical structures which are the ventral striatum, substantia nigra/ventral tegmental region. It plays the key role of monitoring the salience of external inputs and internal brain events. Specifically, it aids in directing attention by identifying important biological and cognitive events. | + | *The salience network consists of several structures, including the anterior (bilateral) insula, dorsal anterior cingulate cortex, and three subcortical structures which are the ventral striatum, substantia nigra/ventral tegmental region. It plays the key role of monitoring the salience of external inputs and internal brain events. Specifically, it aids in directing attention by identifying important biological and cognitive events. |
*This network includes the ventral attention network, which primarily includes the temporoparietal junction and the ventral frontal cortex of the right hemisphere. These areas respond when behaviorally relevant stimuli occur unexpectedly. The ventral attention network is inhibited during focused attention in which top-down processing is being used, such as when visually searching for something. This response may prevent goal-driven attention from being distracted by non-relevant stimuli. It becomes active again when the target or relevant information about the target is found. | *This network includes the ventral attention network, which primarily includes the temporoparietal junction and the ventral frontal cortex of the right hemisphere. These areas respond when behaviorally relevant stimuli occur unexpectedly. The ventral attention network is inhibited during focused attention in which top-down processing is being used, such as when visually searching for something. This response may prevent goal-driven attention from being distracted by non-relevant stimuli. It becomes active again when the target or relevant information about the target is found. | ||
第60行: | 第60行: | ||
*这个网络包括腹侧注意网络,主要包括右半球的颞顶联合区和腹侧额叶皮层。当行为相关的刺激意外发生时,这些区域会做出反应。腹侧注意网络在使用自上而下加工的集中注意过程中被抑制,例如在视觉搜索某物时。这种反应可以防止目标驱动的注意力被非相关的刺激分散。当找到目标或关于目标的相关信息时,它再次激活。 | *这个网络包括腹侧注意网络,主要包括右半球的颞顶联合区和腹侧额叶皮层。当行为相关的刺激意外发生时,这些区域会做出反应。腹侧注意网络在使用自上而下加工的集中注意过程中被抑制,例如在视觉搜索某物时。这种反应可以防止目标驱动的注意力被非相关的刺激分散。当找到目标或关于目标的相关信息时,它再次激活。 | ||
− | ===Attention (Dorsal frontoparietal)=== | + | ===Attention (Dorsal frontoparietal) === |
{{Main|Dorsal attention network}} | {{Main|Dorsal attention network}} | ||
*This network is involved in the voluntary, top-down deployment of attention.<ref name="Riedl" /><ref name="Yuan" /><ref name="Bell" /><ref name="Yeo" /><ref name="Shafiei" /><ref name="Vossel">{{cite journal|last1=Vossel|first1=Simone|last2=Geng|first2=Joy J.|last3=Fink|first3=Gereon R.|title=Dorsal and Ventral Attention Systems: Distinct Neural Circuits but Collaborative Roles|journal=The Neuroscientist|date=2014|volume=20|issue=2|pages=150–159|doi=10.1177/1073858413494269|pmid=23835449|pmc=4107817}}</ref><ref name="Hutton">{{cite journal|last1=Hutton|first1=John S.|last2=Dudley|first2=Jonathan|last3=Horowitz-Kraus|first3=Tzipi|last4=DeWitt|first4=Tom|last5=Holland|first5=Scott K.|title=Functional Connectivity of Attention, Visual, and Language Networks During Audio, Illustrated, and Animated Stories in Preschool-Age Children|journal=Brain Connectivity|date=1 September 2019|volume=9|issue=7|pages=580–592|doi=10.1089/brain.2019.0679|pmid=31144523|pmc=6775495|ref=Hutton}}</ref> Within the dorsal attention network, the intraparietal sulcus and frontal eye fields influence the visual areas of the brain. These influencing factors allow for the orientation of attention.<ref>{{Cite journal|last1=Fox|first1=Michael D.|last2=Corbetta|first2=Maurizio|last3=Snyder|first3=Abraham Z.|last4=Vincent|first4=Justin L.|last5=Raichle|first5=Marcus E.|date=2006-06-27|title=Spontaneous neuronal activity distinguishes human dorsal and ventral attention systems|journal=Proceedings of the National Academy of Sciences|language=en|volume=103|issue=26|pages=10046–10051|doi=10.1073/pnas.0604187103|issn=0027-8424|pmid=16788060|pmc=1480402|bibcode=2006PNAS..10310046F|doi-access=free}}</ref><ref name="Vossel" /><ref name="Bailey" /> | *This network is involved in the voluntary, top-down deployment of attention.<ref name="Riedl" /><ref name="Yuan" /><ref name="Bell" /><ref name="Yeo" /><ref name="Shafiei" /><ref name="Vossel">{{cite journal|last1=Vossel|first1=Simone|last2=Geng|first2=Joy J.|last3=Fink|first3=Gereon R.|title=Dorsal and Ventral Attention Systems: Distinct Neural Circuits but Collaborative Roles|journal=The Neuroscientist|date=2014|volume=20|issue=2|pages=150–159|doi=10.1177/1073858413494269|pmid=23835449|pmc=4107817}}</ref><ref name="Hutton">{{cite journal|last1=Hutton|first1=John S.|last2=Dudley|first2=Jonathan|last3=Horowitz-Kraus|first3=Tzipi|last4=DeWitt|first4=Tom|last5=Holland|first5=Scott K.|title=Functional Connectivity of Attention, Visual, and Language Networks During Audio, Illustrated, and Animated Stories in Preschool-Age Children|journal=Brain Connectivity|date=1 September 2019|volume=9|issue=7|pages=580–592|doi=10.1089/brain.2019.0679|pmid=31144523|pmc=6775495|ref=Hutton}}</ref> Within the dorsal attention network, the intraparietal sulcus and frontal eye fields influence the visual areas of the brain. These influencing factors allow for the orientation of attention.<ref>{{Cite journal|last1=Fox|first1=Michael D.|last2=Corbetta|first2=Maurizio|last3=Snyder|first3=Abraham Z.|last4=Vincent|first4=Justin L.|last5=Raichle|first5=Marcus E.|date=2006-06-27|title=Spontaneous neuronal activity distinguishes human dorsal and ventral attention systems|journal=Proceedings of the National Academy of Sciences|language=en|volume=103|issue=26|pages=10046–10051|doi=10.1073/pnas.0604187103|issn=0027-8424|pmid=16788060|pmc=1480402|bibcode=2006PNAS..10310046F|doi-access=free}}</ref><ref name="Vossel" /><ref name="Bailey" /> | ||
第82行: | 第82行: | ||
*Versions of this network have also been called the central executive (or executive control) network and the cognitive control network.<ref name="Uddin2019" /> | *Versions of this network have also been called the central executive (or executive control) network and the cognitive control network.<ref name="Uddin2019" /> | ||
− | *Versions of this network have also been called the central executive (or executive control) network and the cognitive control network. | + | * Versions of this network have also been called the central executive (or executive control) network and the cognitive control network. |
− | * 这种网络的版本也被称为中央执行(或执行控制)网络和认知控制网络。 | + | *这种网络的版本也被称为中央执行(或执行控制)网络和认知控制网络。 |
===Sensorimotor or Somatomotor (Pericentral)=== | ===Sensorimotor or Somatomotor (Pericentral)=== | ||
{{Main|Sensorimotor network}} | {{Main|Sensorimotor network}} | ||
− | *This network processes somatosensory information and coordinates motion.<ref name="Heine" /><ref name="Yeo" /><ref name="Shafiei" /><ref name="Bassett" /><ref name="Yuan" /> The [[auditory cortex]] may be included.<ref name="Uddin2019" /><ref name="Yeo" /> | + | * This network processes somatosensory information and coordinates motion.<ref name="Heine" /><ref name="Yeo" /><ref name="Shafiei" /><ref name="Bassett" /><ref name="Yuan" /> The [[auditory cortex]] may be included.<ref name="Uddin2019" /><ref name="Yeo" /> |
第101行: | 第101行: | ||
===Visual (Occipital)=== | ===Visual (Occipital)=== | ||
{{See|Visual cortex}} | {{See|Visual cortex}} | ||
− | * This network handles visual information processing.<ref name="Yang">{{cite journal|last1=Yang|first1=Yan-li|last2=Deng|first2=Hong-xia|last3=Xing|first3=Gui-yang|last4=Xia|first4=Xiao-luan|last5=Li|first5=Hai-fang|title=Brain functional network connectivity based on a visual task: visual information processing-related brain regions are significantly activated in the task state|journal=Neural Regeneration Research|date=2015|volume=10|issue=2|pages=298–307|doi=10.4103/1673-5374.152386|pmid=25883631|pmc=4392680 }}</ref> | + | *This network handles visual information processing.<ref name="Yang">{{cite journal|last1=Yang|first1=Yan-li|last2=Deng|first2=Hong-xia|last3=Xing|first3=Gui-yang|last4=Xia|first4=Xiao-luan|last5=Li|first5=Hai-fang|title=Brain functional network connectivity based on a visual task: visual information processing-related brain regions are significantly activated in the task state|journal=Neural Regeneration Research|date=2015|volume=10|issue=2|pages=298–307|doi=10.4103/1673-5374.152386|pmid=25883631|pmc=4392680 }}</ref> |
第126行: | 第126行: | ||
*Cerebellar | *Cerebellar | ||
*Spatial attention | *Spatial attention | ||
− | * Language | + | *Language |
*Lateral visual | *Lateral visual | ||
*Temporal | *Temporal | ||
第132行: | 第132行: | ||
其他网络不同的方法和数据已经确定了其他几个大脑网络,其中许多网络极大地重叠或者是更具特色的核心网络的子集。 | 其他网络不同的方法和数据已经确定了其他几个大脑网络,其中许多网络极大地重叠或者是更具特色的核心网络的子集。 | ||
− | * 边缘 | + | *边缘 |
*听觉 | *听觉 | ||
*右/左执行 | *右/左执行 | ||
第149行: | 第149行: | ||
=<nowiki>= = =</nowiki>= | =<nowiki>= = =</nowiki>= | ||
− | *复杂网络 | + | * 复杂网络 |
* | * | ||
*神经网络 | *神经网络 |
2022年4月4日 (一) 15:55的版本
此词条由神经动力学模型读书会词条梳理志愿者Shenky20翻译审校,翻译字数共800,未经专家审核,带来阅读不便,请见谅。
Large-scale brain networks (also known as intrinsic brain networks) are collections of widespread brain regions showing functional connectivity by statistical analysis of the fMRI BOLD signal[1] or other recording methods such as EEG,[2] PET[3] and MEG.[4] An emerging paradigm in neuroscience is that cognitive tasks are performed not by individual brain regions working in isolation but by networks consisting of several discrete brain regions that are said to be "functionally connected". Functional connectivity networks may be found using algorithms such as cluster analysis, spatial independent component analysis (ICA), seed based, and others.[5] Synchronized brain regions may also be identified using long-range synchronization of the EEG, MEG, or other dynamic brain signals.[6]
Large-scale brain networks (also known as intrinsic brain networks) are collections of widespread brain regions showing functional connectivity by statistical analysis of the fMRI BOLD signal or other recording methods such as EEG, PET and MEG. An emerging paradigm in neuroscience is that cognitive tasks are performed not by individual brain regions working in isolation but by networks consisting of several discrete brain regions that are said to be "functionally connected". Functional connectivity networks may be found using algorithms such as cluster analysis, spatial independent component analysis (ICA), seed based, and others. Synchronized brain regions may also be identified using long-range synchronization of the EEG, MEG, or other dynamic brain signals.
大规模脑网络Large-scale brain networks(也称为内在大脑网络Intrinsic brain networks)是在对基于血氧水平依赖效应BOLD的功能性磁共振成像fMRI信号的统计分析或其他记录方法(如脑电图EEG、正电子发射断层扫描技术PET和脑磁图MEG)中,表现出功能连接Functional connectivity的脑区Brain regions的集合。根据神经科学中一个新出现的范式,认知任务不是由单个脑区独立执行的,而是由几个互不相连的脑区“功能连接”组成的网络执行的。功能连接网络可以通过数据聚类Cluster analysis、空间独立元素分析ICA、种子点方法等算法来发现。同步的脑区也可以用脑电图、脑磁图或其他动态脑信号的远程同步来识别。
The set of identified brain areas that are linked together in a large-scale network varies with cognitive function.[7] When the cognitive state is not explicit (i.e., the subject is at "rest"), the large-scale brain network is a resting state network (RSN). As a physical system with graph-like properties,[6] a large-scale brain network has both nodes and edges and cannot be identified simply by the co-activation of brain areas. In recent decades, the analysis of brain networks was made feasible by advances in imaging techniques as well as new tools from graph theory and dynamical systems.
The set of identified brain areas that are linked together in a large-scale network varies with cognitive function. When the cognitive state is not explicit (i.e., the subject is at "rest"), the large-scale brain network is a resting state network (RSN). As a physical system with graph-like properties, a large-scale brain network has both nodes and edges and cannot be identified simply by the co-activation of brain areas. In recent decades, the analysis of brain networks was made feasible by advances in imaging techniques as well as new tools from graph theory and dynamical systems.
大规模脑网络中,连接在一起的脑区集合因认知功能的不同而不同。当认知状态不明确(即主体处于“静止”状态)时,大规模脑网络是一个静息状态Resting state网络(RSN)。作为一个具有图形特征的物理系统,大规模脑网络既有节点又有边,不能简单地通过脑区的共同激活来识别。近几十年来,成像技术不断进步,同时图论Graph theory、动力学系统Dynamical systems领域出现了新的技术手段,这使得脑网络分析变得可行。
Large-scale brain networks are identified by their function and provide a coherent framework for understanding cognition by offering a neural model of how different cognitive functions emerge when different sets of brain regions join together as self-organized coalitions. The number and composition of the coalitions will vary with the algorithm and parameters used to identify them.[8][9] In one model, there is only the default mode network and the task-positive network, but most current analyses show several networks, from a small handful to 17.[8] The most common and stable networks are enumerated below. The regions participating in a functional network may be dynamically reconfigured.[5][10]
Large-scale brain networks are identified by their function and provide a coherent framework for understanding cognition by offering a neural model of how different cognitive functions emerge when different sets of brain regions join together as self-organized coalitions. The number and composition of the coalitions will vary with the algorithm and parameters used to identify them. In one model, there is only the default mode network and the task-positive network, but most current analyses show several networks, from a small handful to 17. The most common and stable networks are enumerated below. The regions participating in a functional network may be dynamically reconfigured.
大规模脑网络是通过其功能来进行识别的。通过研究大规模脑网络建立神经模型,对不同脑区组合所形成的自组织联合体如何实现不同的认知Cognition功能进行解释,就能够为认知理解提供一个连贯的框架。识别算法和参数的不同会导致所识别出的上述联合体的数量和组成有所不同。一个模型理论认为,符合上述条件的神经模型只包含默认模式网络Default mode network和任务激活网络Task-positive network,但目前大多数分析理论都包括从几个到17个不等的网络。下面列举了最常见且稳定的网络。 人脑可以动态地重新配置参与功能网络的脑区。
Disruptions in activity in various networks have been implicated in neuropsychiatric disorders such as depression, Alzheimer's, autism spectrum disorder, schizophrenia, ADHD[11] and bipolar disorder.[12]
Disruptions in activity in various networks have been implicated in neuropsychiatric disorders such as depression, Alzheimer's, autism spectrum disorder, schizophrenia, ADHD and bipolar disorder.
脑网络活动的中断与诸多神经精神疾病密切相关,如抑郁症Depression、老年痴呆症Alzheimer's、自闭症谱系障碍Autism spectrum disorder、精神分裂症Schizophrenia、多动症ADHD和躁郁症Bipolar disorder。
Core networks
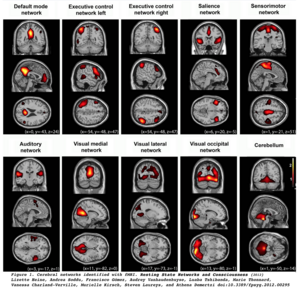
Because brain networks can be identified at various different resolutions and with various different neurobiological properties, there is no such thing as a universal atlas of brain networks that fits all circumstances.[14] While acknowledging this problem, Uddin, Yeo, and Spreng proposed in 2019[15] that the following six networks should be defined as core networks based on converging evidences from multiple studies[16][8][17] to facilitate communication between researchers.
Because brain networks can be identified at various different resolutions and with various different neurobiological properties, there is no such thing as a universal atlas of brain networks that fits all circumstances. While acknowledging this problem, Uddin, Yeo, and Spreng proposed in 2019 that the following six networks should be defined as core networks based on converging evidences from multiple studies to facilitate communication between researchers.
因为大脑网络可以用不同的分辨率和不同的神经生物学特性来识别,所以没有适合所有情况的通用大脑网络图谱。在承认这个问题的同时,Uddin,Yeo,和 Spreng 在2019年提出,以下六个网络应该被定义为核心网络,基于来自多个研究的聚合证据,以促进研究人员之间的交流。
Default Mode (Medial frontoparietal)
- The default mode network is active when an individual is awake and at rest. It preferentially activates when individuals focus on internally-oriented tasks such as daydreaming, envisioning the future, retrieving memories, and theory of mind. It is negatively correlated with brain systems that focus on external visual signals. It is the most widely researched network.[6][10][18][1][19][20][13][8][21][22]
- The default mode network is active when an individual is awake and at rest. It preferentially activates when individuals focus on internally-oriented tasks such as daydreaming, envisioning the future, retrieving memories, and theory of mind. It is negatively correlated with brain systems that focus on external visual signals. It is the most widely researched network.
= = 默认模式(Medial frontoparietal) = =
- 默认模式网络在个人清醒和休息时处于活动状态。当个体专注于面向内部的任务时,比如做白日梦、展望未来、回忆和心理理论,它就会优先激活。它与专注于外部视觉信号的大脑系统负相关。它是研究最广泛的网络。
Salience (Midcingulo-Insular)
- The salience network consists of several structures, including the anterior (bilateral) insula, dorsal anterior cingulate cortex, and three subcortical structures which are the ventral striatum, substantia nigra/ventral tegmental region.[23][24] It plays the key role of monitoring the salience of external inputs and internal brain events.[1][6][10][19][13][8][21] Specifically, it aids in directing attention by identifying important biological and cognitive events.[24][22]
- This network includes the ventral attention network, which primarily includes the temporoparietal junction and the ventral frontal cortex of the right hemisphere.[15][25] These areas respond when behaviorally relevant stimuli occur unexpectedly.[25] The ventral attention network is inhibited during focused attention in which top-down processing is being used, such as when visually searching for something. This response may prevent goal-driven attention from being distracted by non-relevant stimuli. It becomes active again when the target or relevant information about the target is found.[25][26]
- The salience network consists of several structures, including the anterior (bilateral) insula, dorsal anterior cingulate cortex, and three subcortical structures which are the ventral striatum, substantia nigra/ventral tegmental region. It plays the key role of monitoring the salience of external inputs and internal brain events. Specifically, it aids in directing attention by identifying important biological and cognitive events.
- This network includes the ventral attention network, which primarily includes the temporoparietal junction and the ventral frontal cortex of the right hemisphere. These areas respond when behaviorally relevant stimuli occur unexpectedly. The ventral attention network is inhibited during focused attention in which top-down processing is being used, such as when visually searching for something. This response may prevent goal-driven attention from being distracted by non-relevant stimuli. It becomes active again when the target or relevant information about the target is found.
突显网络由前(双)岛、前扣带回皮层和腹侧纹状体、黑质/腹侧被盖区3个皮层下结构组成。它起着监测外部输入和内部脑事件的显著性的关键作用。具体来说,它通过识别重要的生物学和认知事件来帮助引导注意力。
- 这个网络包括腹侧注意网络,主要包括右半球的颞顶联合区和腹侧额叶皮层。当行为相关的刺激意外发生时,这些区域会做出反应。腹侧注意网络在使用自上而下加工的集中注意过程中被抑制,例如在视觉搜索某物时。这种反应可以防止目标驱动的注意力被非相关的刺激分散。当找到目标或关于目标的相关信息时,它再次激活。
Attention (Dorsal frontoparietal)
- This network is involved in the voluntary, top-down deployment of attention.[1][19][20][8][21][25][27] Within the dorsal attention network, the intraparietal sulcus and frontal eye fields influence the visual areas of the brain. These influencing factors allow for the orientation of attention.[28][25][22]
- This network is involved in the voluntary, top-down deployment of attention. Within the dorsal attention network, the intraparietal sulcus and frontal eye fields influence the visual areas of the brain. These influencing factors allow for the orientation of attention.
= = 注意力(背侧额顶骨) = =
- 这个网络参与了自发的、自上而下的注意力分配。在背侧注意网络中,顶内沟和额眼影响大脑的视觉区域。这些影响因素决定了注意力的方向。
Control (Lateral frontoparietal)
- This network initiates and modulates cognitive control and comprises 18 sub-regions of the brain.[29] There is a strong correlation between fluid intelligence and the involvement of the fronto-parietal network with other networks.[30]
- This network initiates and modulates cognitive control and comprises 18 sub-regions of the brain. There is a strong correlation between fluid intelligence and the involvement of the fronto-parietal network with other networks.
= = 控制(侧额顶骨) = =
- 这个网络启动和调节认知控制,包括大脑的18个亚区。在流体智力和额顶网络与其他网络的参与之间有很强的相关性。
- Versions of this network have also been called the central executive (or executive control) network and the cognitive control network.[15]
- Versions of this network have also been called the central executive (or executive control) network and the cognitive control network.
- 这种网络的版本也被称为中央执行(或执行控制)网络和认知控制网络。
Sensorimotor or Somatomotor (Pericentral)
- This network processes somatosensory information and coordinates motion.[13][8][21][10][19] The auditory cortex may be included.[15][8]
- This network processes somatosensory information and coordinates motion. The auditory cortex may be included.
Sensorimotor or Somatomotor (Pericentral)
- This network processes somatosensory information and coordinates motion.听觉皮层可能也包括在内。
Visual (Occipital)
- This network handles visual information processing.[31]
- This network handles visual information processing.
= = = = = = = = = = = = = 这个网络处理视觉信息。
Other networks
Different methods and data have identified several other brain networks, many of which greatly overlap or are subsets of more well-characterized core networks.[15]
- Limbic[10][8][22]
- Auditory[19][13]
- Right/left executive[19][13]
- Cerebellar[20][13]
- Spatial attention[1][6]
- Language[6][27]
- Lateral visual[19][20][13]
- Temporal[8][21]
- Visual perception/imagery[27]
Different methods and data have identified several other brain networks, many of which greatly overlap or are subsets of more well-characterized core networks.
- Limbic
- Auditory
- Right/left executive
- Cerebellar
- Spatial attention
- Language
- Lateral visual
- Temporal
- Visual perception/imagery
其他网络不同的方法和数据已经确定了其他几个大脑网络,其中许多网络极大地重叠或者是更具特色的核心网络的子集。
- 边缘
- 听觉
- 右/左执行
- 小脑
- 空间注意
- 语言
- 外侧视觉
- 颞视知觉/图像
See also
- Complex network
- Neural network
= = =
- 复杂网络
- 神经网络
References
- ↑ 1.0 1.1 1.2 1.3 1.4 Riedl, Valentin; Utz, Lukas; Castrillón, Gabriel; Grimmer, Timo; Rauschecker, Josef P.; Ploner, Markus; Friston, Karl J.; Drzezga, Alexander; Sorg, Christian (January 12, 2016). "Metabolic connectivity mapping reveals effective connectivity in the resting human brain". PNAS. 113 (2): 428–433. Bibcode:2016PNAS..113..428R. doi:10.1073/pnas.1513752113. PMC 4720331. PMID 26712010.
- ↑ Foster, Brett L.; Parvizi, Josef (2012-03-01). "Resting oscillations and cross-frequency coupling in the human posteromedial cortex". NeuroImage. 60 (1): 384–391. doi:10.1016/j.neuroimage.2011.12.019. ISSN 1053-8119. PMC 3596417. PMID 22227048.
- ↑ Buckner, Randy L.; Andrews‐Hanna, Jessica R.; Schacter, Daniel L. (2008). "The Brain's Default Network". Annals of the New York Academy of Sciences (in English). 1124 (1): 1–38. Bibcode:2008NYASA1124....1B. doi:10.1196/annals.1440.011. ISSN 1749-6632. PMID 18400922. S2CID 3167595.
- ↑ Morris, Peter G.; Smith, Stephen M.; Barnes, Gareth R.; Stephenson, Mary C.; Hale, Joanne R.; Price, Darren; Luckhoo, Henry; Woolrich, Mark; Brookes, Matthew J. (2011-10-04). "Investigating the electrophysiological basis of resting state networks using magnetoencephalography". Proceedings of the National Academy of Sciences (in English). 108 (40): 16783–16788. Bibcode:2011PNAS..10816783B. doi:10.1073/pnas.1112685108. ISSN 0027-8424. PMC 3189080. PMID 21930901.
- ↑ 5.0 5.1 Petersen, Steven; Sporns, Olaf (October 2015). "Brain Networks and Cognitive Architectures". Neuron. 88 (1): 207–219. doi:10.1016/j.neuron.2015.09.027. PMC 4598639. PMID 26447582.
- ↑ 6.0 6.1 6.2 6.3 6.4 6.5 Bressler, Steven L.; Menon, Vinod (June 2010). "Large scale brain networks in cognition: emerging methods and principles". Trends in Cognitive Sciences. 14 (6): 233–290. doi:10.1016/j.tics.2010.04.004. PMID 20493761. S2CID 5967761. Retrieved 24 January 2016.
- ↑ Bressler, Steven L. (2008). "Neurocognitive networks". Scholarpedia. 3 (2): 1567. Bibcode:2008SchpJ...3.1567B. doi:10.4249/scholarpedia.1567.
- ↑ 8.0 8.1 8.2 8.3 8.4 8.5 8.6 8.7 8.8 8.9 Yeo, B. T. Thomas; Krienen, Fenna M.; Sepulcre, Jorge; Sabuncu, Mert R.; Lashkari, Danial; Hollinshead, Marisa; Roffman, Joshua L.; Smoller, Jordan W.; Zöllei, Lilla; Polimeni, Jonathan R.; Fischl, Bruce; Liu, Hesheng; Buckner, Randy L. (2011-09-01). "The organization of the human cerebral cortex estimated by intrinsic functional connectivity". Journal of Neurophysiology. 106 (3): 1125–1165. Bibcode:2011NatSD...2E0031H. doi:10.1152/jn.00338.2011. PMC 3174820. PMID 21653723.
- ↑ Abou Elseoud, Ahmed; Littow, Harri; Remes, Jukka; Starck, Tuomo; Nikkinen, Juha; Nissilä, Juuso; Timonen, Markku; Tervonen, Osmo; Kiviniemi1, Vesa (2011-06-03). "Group-ICA Model Order Highlights Patterns of Functional Brain Connectivity". Frontiers in Systems Neuroscience. 5: 37. doi:10.3389/fnsys.2011.00037. PMC 3109774. PMID 21687724.
- ↑ 10.0 10.1 10.2 10.3 10.4 Bassett, Daniella; Bertolero, Max (July 2019). "How Matter Becomes Mind". Scientific American. 321 (1): 32. Retrieved 23 June 2019.
- ↑ Griffiths, Kristi R.; Braund, Taylor A.; Kohn, Michael R.; Clarke, Simon; Williams, Leanne M.; Korgaonkar, Mayuresh S. (2 March 2021). "Structural brain network topology underpinning ADHD and response to methylphenidate treatment". Translational Psychiatry. 11 (1): 1–9. doi:10.1038/s41398-021-01278-x. PMC 7925571. PMID 33654073. Retrieved 16 November 2021.
- ↑ Menon, Vinod (2011-09-09). "Large-scale brain networks and psychopathology: A unifying triple network model". Trends in Cognitive Sciences. 15 (10): 483–506. doi:10.1016/j.tics.2011.08.003. PMID 21908230. S2CID 26653572.
- ↑ 13.0 13.1 13.2 13.3 13.4 13.5 13.6 13.7 Heine, Lizette; Soddu, Andrea; Gomez, Francisco; Vanhaudenhuyse, Audrey; Tshibanda, Luaba; Thonnard, Marie; Charland-Verville, Vanessa; Kirsch, Murielle; Laureys, Steven; Demertzi, Athena (2012). "Resting state networks and consciousness. Alterations of multiple resting state network connectivity in physiological, pharmacological and pathological consciousness states". Frontiers in Psychology. 3: 295. doi:10.3389/fpsyg.2012.00295. PMC 3427917. PMID 22969735.
- ↑ Eickhoff, SB; Yeo, BTT; Genon, S (November 2018). "Imaging-based parcellations of the human brain" (PDF). Nature Reviews. Neuroscience. 19 (11): 672–686. doi:10.1038/s41583-018-0071-7. PMID 30305712. S2CID 52954265.
- ↑ 15.0 15.1 15.2 15.3 15.4 Uddin, LQ; Yeo, BTT; Spreng, RN (November 2019). "Towards a Universal Taxonomy of Macro-scale Functional Human Brain Networks". Brain Topography. 32 (6): 926–942. doi:10.1007/s10548-019-00744-6. PMC 7325607. PMID 31707621.
- ↑ Doucet, GE; Lee, WH; Frangou, S (2019-10-15). "Evaluation of the spatial variability in the major resting-state networks across human brain functional atlases". Human Brain Mapping. 40 (15): 4577–4587. doi:10.1002/hbm.24722. PMC 6771873. PMID 31322303.
- ↑ Smith, SM; Fox, PT; Miller, KL; Glahn, DC; Fox, PM; Mackay, CE; Filippini, N; Watkins, KE; Toro, R; Laird, AR; Beckmann, CF (2009-08-04). "Correspondence of the brain's functional architecture during activation and rest". Proceedings of the National Academy of Sciences of the United States of America. 106 (31): 13040–5. Bibcode:2009PNAS..10613040S. doi:10.1073/pnas.0905267106. PMC 2722273. PMID 19620724.
- ↑ Buckner, Randy L. (2012-08-15). "The serendipitous discovery of the brain's default network". NeuroImage (in English). 62 (2): 1137–1145. doi:10.1016/j.neuroimage.2011.10.035. ISSN 1053-8119. PMID 22037421. S2CID 9880586.
- ↑ 19.0 19.1 19.2 19.3 19.4 19.5 19.6 Yuan, Rui; Di, Xin; Taylor, Paul A.; Gohel, Suril; Tsai, Yuan-Hsiung; Biswal, Bharat B. (30 April 2015). "Functional topography of the thalamocortical system in human". Brain Structure and Function. 221 (4): 1971–1984. doi:10.1007/s00429-015-1018-7. PMC 6363530. PMID 25924563.
- ↑ 20.0 20.1 20.2 20.3 Bell, Peter T.; Shine, James M. (2015-11-09). "Estimating Large-Scale Network Convergence in the Human Functional Connectome". Brain Connectivity. 5 (9): 565–74. doi:10.1089/brain.2015.0348. PMID 26005099.
- ↑ 21.0 21.1 21.2 21.3 21.4 Shafiei, Golia; Zeighami, Yashar; Clark, Crystal A.; Coull, Jennifer T.; Nagano-Saito, Atsuko; Leyton, Marco; Dagher, Alain; Mišić, Bratislav (2018-10-01). "Dopamine Signaling Modulates the Stability and Integration of Intrinsic Brain Networks". Cerebral Cortex. 29 (1): 397–409. doi:10.1093/cercor/bhy264. PMC 6294404. PMID 30357316.
- ↑ 22.0 22.1 22.2 22.3 Bailey, Stephen K.; Aboud, Katherine S.; Nguyen, Tin Q.; Cutting, Laurie E. (13 December 2018). "Applying a network framework to the neurobiology of reading and dyslexia". Journal of Neurodevelopmental Disorders. 10 (1): 37. doi:10.1186/s11689-018-9251-z. PMC 6291929. PMID 30541433.
- ↑ Steimke, Rosa; Nomi, Jason S.; Calhoun, Vince D.; Stelzel, Christine; Paschke, Lena M.; Gaschler, Robert; Goschke, Thomas; Walter, Henrik; Uddin, Lucina Q. (2017-12-01). "Salience network dynamics underlying successful resistance of temptation". Social Cognitive and Affective Neuroscience (in English). 12 (12): 1928–1939. doi:10.1093/scan/nsx123. ISSN 1749-5016. PMC 5716209. PMID 29048582.
- ↑ 24.0 24.1 Menon, V. (2015-01-01), "Salience Network", in Toga, Arthur W. (ed.), Brain Mapping, Academic Press, pp. 597–611, doi:10.1016/B978-0-12-397025-1.00052-X, ISBN 978-0-12-397316-0, retrieved 2019-12-08
- ↑ 25.0 25.1 25.2 25.3 25.4 Vossel, Simone; Geng, Joy J.; Fink, Gereon R. (2014). "Dorsal and Ventral Attention Systems: Distinct Neural Circuits but Collaborative Roles". The Neuroscientist. 20 (2): 150–159. doi:10.1177/1073858413494269. PMC 4107817. PMID 23835449.
- ↑ Shulman, Gordon L.; McAvoy, Mark P.; Cowan, Melanie C.; Astafiev, Serguei V.; Tansy, Aaron P.; d'Avossa, Giovanni; Corbetta, Maurizio (2003-11-01). "Quantitative Analysis of Attention and Detection Signals During Visual Search". Journal of Neurophysiology. 90 (5): 3384–3397. doi:10.1152/jn.00343.2003. ISSN 0022-3077. PMID 12917383.
- ↑ 27.0 27.1 27.2 Hutton, John S.; Dudley, Jonathan; Horowitz-Kraus, Tzipi; DeWitt, Tom; Holland, Scott K. (1 September 2019). "Functional Connectivity of Attention, Visual, and Language Networks During Audio, Illustrated, and Animated Stories in Preschool-Age Children". Brain Connectivity. 9 (7): 580–592. doi:10.1089/brain.2019.0679. PMC 6775495. PMID 31144523.
- ↑ Fox, Michael D.; Corbetta, Maurizio; Snyder, Abraham Z.; Vincent, Justin L.; Raichle, Marcus E. (2006-06-27). "Spontaneous neuronal activity distinguishes human dorsal and ventral attention systems". Proceedings of the National Academy of Sciences (in English). 103 (26): 10046–10051. Bibcode:2006PNAS..10310046F. doi:10.1073/pnas.0604187103. ISSN 0027-8424. PMC 1480402. PMID 16788060.
- ↑ Scolari, Miranda; Seidl-Rathkopf, Katharina N; Kastner, Sabine (2015-02-01). "Functions of the human frontoparietal attention network: Evidence from neuroimaging". Current Opinion in Behavioral Sciences. Cognitive control. 1: 32–39. doi:10.1016/j.cobeha.2014.08.003. ISSN 2352-1546. PMC 4936532. PMID 27398396.
- ↑ Marek, Scott; Dosenbach, Nico U. F. (June 2018). "The frontoparietal network: function, electrophysiology, and importance of individual precision mapping". Dialogues in Clinical Neuroscience. 20 (2): 133–140. doi:10.31887/DCNS.2018.20.2/smarek. ISSN 1294-8322. PMC 6136121. PMID 30250390.
- ↑ Yang, Yan-li; Deng, Hong-xia; Xing, Gui-yang; Xia, Xiao-luan; Li, Hai-fang (2015). "Brain functional network connectivity based on a visual task: visual information processing-related brain regions are significantly activated in the task state". Neural Regeneration Research. 10 (2): 298–307. doi:10.4103/1673-5374.152386. PMC 4392680. PMID 25883631.
Category:Neuroscience Category:Neural coding Category:Neural circuits Category:Neurophysiology
类别: 神经科学类别: 神经编码类别: 神经回路类别: 神经生理学
This page was moved from wikipedia:en:Large-scale brain network. Its edit history can be viewed at 大规模脑网络/edithistory