“神经场”的版本间的差异
(→生理学动机) |
|||
第18行: | 第18行: | ||
具有化学受体对单个传入脉冲和传入瞬时脉冲序列 P(t) 的突触响应函数 h(t)。 单个突触的实验研究表明某些函数 h(t) 可以很好地近似突触反应行为。 在神经领域,人们考虑一个有效的平均突触响应函数,它描述了许多突触对神经群体网络中许多传入脉冲序列的平均响应。 当然,一组突触的平均时间响应可能与单个突触受体的响应函数不同,但形状可能相同。因此,Eq.\ref{eqn_1} 也适用于群体树突电流 I(t),其中涉及有效参数和与突触群体响应函数 h(t)相关的群体放电率 P(t)。 | 具有化学受体对单个传入脉冲和传入瞬时脉冲序列 P(t) 的突触响应函数 h(t)。 单个突触的实验研究表明某些函数 h(t) 可以很好地近似突触反应行为。 在神经领域,人们考虑一个有效的平均突触响应函数,它描述了许多突触对神经群体网络中许多传入脉冲序列的平均响应。 当然,一组突触的平均时间响应可能与单个突触受体的响应函数不同,但形状可能相同。因此,Eq.\ref{eqn_1} 也适用于群体树突电流 I(t),其中涉及有效参数和与突触群体响应函数 h(t)相关的群体放电率 P(t)。 | ||
− | + | 在数学上,从 P(t) 到 I(t) 的转换是具有核 h(t) 的 Volterra 积分方程。 考虑微分方程通常比考虑积分方程更方便,因此可以尝试计算积分算子的逆。 这对于某些积分核函数 h(t) 是可能的。 一个简单且应用广泛的模型是 <math>h(t)=\exp(-t/\tau)/\tau</math> 衰减时间常数为 <math>\tau</math>。然后 | |
<math> | <math> | ||
第27行: | 第27行: | ||
</math> | </math> | ||
− | + | 应用链式法则和方程式 (\ref{eqn_1}) 可以写成微分方程 | |
<math> | <math> | ||
第33行: | 第33行: | ||
</math> | </math> | ||
− | + | 更详细的响应函数及其对应的微分算子是 | |
<math> | <math> | ||
第40行: | 第40行: | ||
</math> | </math> | ||
− | + | 具有较短的上升时间 <math>\tau_2</math>和较长的衰减时间 <math>\tau_1</math>。 | |
− | + | 对于 <math>\tau_1=\tau_2=\tau</math>, 响应函数就是所谓的阿尔法函数 | |
<math> | <math> |
2022年6月11日 (六) 11:23的版本
神经场方程是组织层面的模型,描述了粗粒度变量的时空演变,例如神经元群体中的突触或放电率活动。
简介
即使是一小块皮层中的神经元和突触的数量也是巨大的。因此,一种流行的建模方法是采用连续极限并研究神经网络(神经网络中,空间是连续的,并且宏观状态变量等于平均发放率)。首次尝试开发神经活动的连续近似,或可追溯到 1950 年代的 Beurle (1956) 和 1960 年代的 Griffith (1963, 1965)。通过关注给定体积的模型大脑中每单位时间被激活的神经元比例组织,Beurle 能够分析大规模大脑活动的触发和传导。然而,这项工作只处理没有难治或恢复变量的兴奋性神经元网络。Wilson和 Cowan(1972,1973) 在 1970 年代将 Beurle 的工作扩展到包括抑制性和兴奋性神经元以及不应期。Amari (1975, 1977) 在对连通性和放电率函数的自然假设下,在对经活动的连续模型中进行了进一步工作,特别是关于模式形成。Amari 考虑了局部激发和远端抑制对于具有典型皮质连接(通常称为“墨西哥帽连接”)的相互作用的抑制性和兴奋性神经元的混合群体,这是一个有效的模型。
由于这些对动态神经场理论的开创性贡献,类似的模型已被用于研究EEG节律、视觉幻觉、短期记忆和运动感知机制。
生理学动机
神经领域考虑嵌入在粗粒度空间区域中的神经元群体(Wilson & Cowan 1973)。这种区域的颗粒反映了在初级感觉区域中观察到的微观或宏观柱状体,例如啮齿动物的桶状皮层 (Petersen 2007) 或哺乳动物的视觉皮层 (Hubel & Wiesel 1962, Saez et al. 1998)。此外,神经领域考虑瞬时种群放电率(种群编码),即在几毫秒的某个短时间间隔内放电神经元的数量。神经场模型中的时间变量是这个时间间隔的倍数。因此,神经场在时间和空间上是粗粒度的,并表示平均场模型。重要的是要提到与神经质量模型的相似性,后者忽略了空间扩展,但涉及到关于人口编码和时间粒度的相同基本假设。
Beurle (1956) 和 Wilson & Cowan (1972, 1973) 是最早从数学上推导出神经场模型方程的人之一。最近 Faugeras 等人。 (2009) 和 Bressloff (2009) 考虑到单个神经元的统计特性和随机动力学,给出了不同的推导。有趣的是,他们提出了一个扩展模型,同时考虑了平均活动及其方差的动态,而以前的模型只考虑了平均活动。以下段落给出了神经场模型的更具启发性的推导,其中考虑了神经作用的三个主要要素:输入脉冲在化学突触处引起的树突电流、受到树突电流影响的神经元的放电以及沿轴突分支的动作电位传输。诱发的树枝状电流 I(t) 服从
[math]\displaystyle{ I(t) = \int_{-\infty}^t {\rm d}\tau^\prime h(t-t^\prime) P(t^\prime)\label{eqn_1} }[/math]
具有化学受体对单个传入脉冲和传入瞬时脉冲序列 P(t) 的突触响应函数 h(t)。 单个突触的实验研究表明某些函数 h(t) 可以很好地近似突触反应行为。 在神经领域,人们考虑一个有效的平均突触响应函数,它描述了许多突触对神经群体网络中许多传入脉冲序列的平均响应。 当然,一组突触的平均时间响应可能与单个突触受体的响应函数不同,但形状可能相同。因此,Eq.\ref{eqn_1} 也适用于群体树突电流 I(t),其中涉及有效参数和与突触群体响应函数 h(t)相关的群体放电率 P(t)。
在数学上,从 P(t) 到 I(t) 的转换是具有核 h(t) 的 Volterra 积分方程。 考虑微分方程通常比考虑积分方程更方便,因此可以尝试计算积分算子的逆。 这对于某些积分核函数 h(t) 是可能的。 一个简单且应用广泛的模型是 [math]\displaystyle{ h(t)=\exp(-t/\tau)/\tau }[/math] 衰减时间常数为 [math]\displaystyle{ \tau }[/math]。然后
[math]\displaystyle{ \begin{array}{lcl} \frac{dI(t)}{dt}&=&-\frac{1}{\tau}\int_{-\infty}^t {\rm d}t^\prime \exp\left(-(t-t^\prime)/\tau\right)/\tau P(t^\prime) + \int_{-\infty}^t \left({\rm d}t^\prime/dt\right)|_{\infty}^t \exp\left(-(t-t^\prime)/\tau\right)/\tau P(t^\prime)\\ &=&-\frac{1}{\tau}I(t) + \frac{1}{\tau} P(t) \end{array} }[/math]
应用链式法则和方程式 (\ref{eqn_1}) 可以写成微分方程
[math]\displaystyle{ \hat{L} I(t)=P(t)\quad,\quad \hat{L}=\tau\frac{d}{dt}+1 . }[/math]
更详细的响应函数及其对应的微分算子是
[math]\displaystyle{ h(t)=\frac{1}{\tau_1-\tau_2}\left(e^{-t/\tau_1}-e^{t/\tau_2}\right) \quad , \quad \hat{L}=\tau_1\tau_2\frac{d^2}{dt^2}+\left(\tau_1+\tau_2\right)\frac{d}{dt}+1 }[/math]
具有较短的上升时间 [math]\displaystyle{ \tau_2 }[/math]和较长的衰减时间 [math]\displaystyle{ \tau_1 }[/math]。 对于 [math]\displaystyle{ \tau_1=\tau_2=\tau }[/math], 响应函数就是所谓的阿尔法函数
[math]\displaystyle{ h(t)=te^{-t/\tau}/\tau\quad , \quad \hat{L}=\tau^2\frac{d^2}{dt^2}+2\tau\frac{d}{dt}+1~. }[/math]
Mathematical Framework
In many continuum models for the propagation of electrical activity in neural tissue it is assumed that the synaptic input current is a function of the pre-synaptic firing rate function (Wilson & Cowan 1973). These infinite dimensional dynamical systems are typically variations on the form
[math]\displaystyle{ \frac{1}{\alpha} \frac{\partial u(x,t)}{\partial t} = -u + \int_{-\infty}^\infty {\rm d} y w(y) f(u(x-y,t - |y|/v)) . }[/math]
Here, u(x,t) is interpreted as a neural field representing the local activity of a population of neurons at position x and time t. The second term on the right represents the synaptic input, with f interpreted as the firing rate function of a single neuron. The strength of connections between neurons separated by a distance y is denoted w(y), and the function w is often referred to as the synaptic footprint. This formulation assumes that the system is spatially homogeneous and isotropic. Typically w reflects global excitation (w>0), global inhibition (w<0), local excitation - lateral inhibition (Mexican hat) describing, e.g. orientation tuning in the visual cortex (Somers et al. 1995, Ben-Yishai et al. 1995), or local inhibition - lateral excitation (inverse Mexican hat) reflecting short-range interactions of inhibitory interneurons and long-range interactions of excitatory pyramidal cells. Periodic w have also attracted some attention (Ben-Yishai et al. 1995, Laing and Troy 2003). The parameter [math]\displaystyle{ \alpha }[/math] is the temporal decay rate of the synapse. The delayed argument to u under the spatial integral represents the axonal conduction delay arising from the finite speed of signals travelling over a distance y (Wilson & Cowan 1972; Nunez 1974; Jirsa & Haken 1997); namely |y|/v where v is the velocity of an action potential along axonal fibres. Recent extensions involve distributions of axonal transmission speeds v (Atay and Hutt 2006).
There are several natural choices for the firing rate function, the simplest being a Heaviside step function. In this case a neuron fires maximally (at a rate set by its absolute refractory period) or not at all, depending on whether or not synaptic activity is above or below some threshold. In a statistical mechanics approach to formulating mean-field neural equations this all or nothing response is replaced by a smooth sigmoidal form (Wilson & Cowan 1972; Amari 1972).
The simple mathematical model above can be naturally extended to describe multiple populations, cortical sheets, spike frequency adaptation, neuromodulation, slow ionic currents and more sophisticated forms of synaptic and dendritic processing as described in the review articles below.
Dynamic behaviour
The sorts of dynamic behaviour that are typically observed in neural field models include spatially and temporally periodic patterns beyond a Turing instability (Ermentrout 1979; Tass 1995), localised regions of activity such as bumps (Kishimoto 1979) and travelling waves (Ermentrout 1993; Pinto & Ermentrout 2001). In the latter case corresponding phenomena may be observed experimentally using multi-electrode recordings and imaging methods. In particular it is possible to electrically stimulate slices of pharmacologically treated tissue taken from the cortex (Chervin et al. 1988; Golomb & Amitai 1997,Wu et al 1999}, hippocampus (Miles et al. 1995) and thalamus (Kim et al. 1995). In brain slices these waves can take the form of synchronous discharge seen during an epileptic seizure (Connors & Amitai 1993) and spreading excitation associated with sensory processing (Ermentrout & Kleinfeld 2001). Interestingly, spatially localised bumps of activity have been linked to working memory (the temporary storage of information within the brain) in prefrontal cortex (Colby et al. 1995, Goldman-Rakic 1995), representations in the head-direction system (Zhang 1996), and feature selectivity in the visual cortex, where bump formation is related to the tuning of a particular neuron's response (Ben-Yishai et al. 1995).
Pattern formation
Neural field models are nonlinear spatially extended systems and thus have all the necessary ingredients to support pattern formation. The analysis of such behaviour is typically performed with a mixture of linear Turing instability theory, weakly nonlinear perturbative analysis and numerical simulations. In one dimension single population models with Mexican-hat connectivity can support global periodic stationary patterns. With more than one population non-stationary (travelling) patterns are also possible. In two dimensions many other interesting patterns can occur such as spiral waves (Laing 2005), target waves and doubly periodic patterns. These latter patterns take the form of stripes and checkerboard like patterns, and have been linked by Ermentrout & Cowan (1979) and Bressloff et al (2001) to drug-induced visual hallucinations.
Neural field models with short-range excitation and long-range inhibition are also able to support spatially localised solutions, commonly referred to as bumps or multi-bumps. For the case that the firing rate function is a Heaviside step function with threshold h Amari (1977) was able to construct an explicit one-bump solution of the form
[math]\displaystyle{ u(x) = \int_0^\Delta w(x-y) {\rm d y}, \qquad u(0)=h=u(\Delta) , }[/math]
such that below some critical threshold there co-exists both a wide and a narrow solution. Of the two, it is the wider solution that is stable. For smooth sigmoidal firing rates no closed-form spatially localised solutions are known, though much insight into the form of multi-bump solutions has been obtained using techniques first developed for the study of fourth order pattern forming systems (Laing & Troy 2003). A stationary activity bump can exhibit a variety of dynamical instabilities including a Hopf bifurcation to a spatially localized oscillatory solution or breather (Folias and Bressloff 2004, Coombes and Owen 2005).
One possible computational role for an activity bump is to encode a set of stimulus features in terms of the peak location of the bump within a spatially-structured network. In the case of a homogeneous network, the set of allowed features will form a continuous manifold (attractor) that reflects the underlying topology of the network. Thus, a periodic stimulus feature can be encoded by an activity bump in a homogeneous ring network (Ben-Yishai et al 1995, Zhang 1995). However, one of the consequences of a homogeneous network is that the bump will be marginally stable with respect to spatial translations tangential to the continuous manifold. This means that the activity bump will slowly drift over time in the presence of arbitrarily small levels of noise (Laing and Chow 2001). One way to construct neural field models that are robust to noise is to introduce some form of cellular bistability (Camperi and Wang 1998, Fall et al 2004).
Travelling Waves
For one-dimensional models with sigmoidal firing rate functions and excitatory coupling it is possible to find wave fronts joining an excited state to a resting state (Ermentrout & McLeod 1993). Moreover, in systems with mixed (excitatory and inhibitory) coupling or excitatory systems with adaptive currents, solitary travelling pulses are also possible. For a Heaviside firing rate function with threshold h many exact results about travelling waves have been obtained. For example the speed of a stable travelling front in a purely excitatory network with w(x)=exp(-|x|)/2 takes the explicit form
[math]\displaystyle{ c = \frac{v(2h-1)}{2h-1 - 2 h v/\alpha} }[/math]
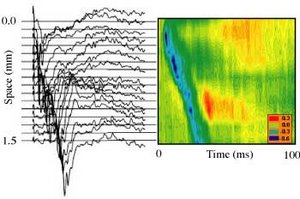
The strong dependence of the wave speed on the threshold h has now been indirectly established in real neural tissue (rat cortical slices bathed in the GABA_A blocker picrotoxin) by Richardson et al. 2005. These experiments exploit the fact that cortical neurons have long apical dendrites and are easily polarisable by an electric field and that epileptiform bursts can be initiated by a stimulation electrode. An applied positive (negative) electric field across the slice increased (decreased) the speed of wave propagation, consistent with the theoretical predictions of neural field theory assuming that a positive (negative) electric field reduces (increases) the threshold h.
The bifurcation structure of travelling waves can be analysed using a so-called Evans function. This was originally formulated
by Evans (1975) in the context of a stability theorem about
excitable nerve axon equations of Hodgkin–Huxley type. The zeros of this complex analytic function determine the normal spectrum of the operator obtained by linearising a system about its travelling wave solution.
The extension to neural field models is more recent and, for the special case of a Heaviside firing rate function, several models have now been studied (Coombes & Owen 2004, 2005).
One of the common assumptions in most neural field models is that the network is homogeneous and isotropic, that is, the weight distribution depends on the distance between interacting populations within the network. The real cortex, however, is more realistically modeled as an anisotropic and inhomogeneous two-dimensional medium due to the patchy nature of long-range horizontal connections found in superficial layers of cortex (Bosking et al 1997). Anisotropies in the weight distribution could lead to variations in wave speed in different directions, whereas inhomogeneities could lead to time-varying wave profiles and possibly wave propagation failure (Bressloff 2001).
References
- S Amari. Dynamics of pattern formation in lateral inhibition type neural fields. Biological Cybernetics, 27:77–87, 1977.
- S Amari. Homogeneous nets of neuron-like elements. Biological Cybernetics, 17:211–220, 1975.
- F.M. Atay and A.Hutt, Neural fields with distributed transmission speeds and long-range feedback delays. SIAM Journal Applied Dynamical Systems 5(4), 670-698, 2006
- R Ben-Yishai, L Bar-Or, and H Sompolinsky. Theory of orientation tuning in visual cortex. Proceedings of the National Academy of Sciences USA, 92:3844–3848, 1995.
- R L Beurle. Properties of a mass of cells capable of regenerating pulses. Philosophical Transactions of the Royal Society London B, 240:55–94, 1956.
- W H Bosking, Y Zhang, B Schofield and D Fitzpatrick. Orientation selectivity and the arrangement of horizontal connections in tree shrew striate cortex. J. Neurosci., 17:2112-2127, 1997.
- P C Bressloff. Traveling fronts and wave propagation failure in an inhomogeneous neural network. Physica D, 155:83-100, 2001.
- P C Bressloff, J D Cowan, M Golubitsky, P J Thomas and M Wiener. Geometric visual hallucinations, Euclidean symmetry and the functional architecture of striate cortex. Phil. Trans. Roy. Soc. B, 356: 299-330.
- P C Bressloff. Stochastic neural field theory and the system-size expansion. SIAM J. Applied Mathematics, 70:1488-1521, 2009
- M Camperi and X-J Wang. A model of visuospatial short-term memory in prefrontal cortex: recurrent network and cellular bistability. J. Comp. Neurosci., 5:383-405, 1998.
- R D Chervin, P A Pierce, and BWConnors. Periodicity and directionality in the propagation of epileptiform discharges across neortex. Journal of Neurophysiology, 60:1695–1713, 1988.
- B W Connors and Y Amitai. Generation of epileptiform discharges by local circuits in neocortex. In P A Schwartzkroin, editor, Epilepsy: Models, Mechanisms and Concepts, pages 388–424. Cambridge University Press, 1993.
- C L Colby, J R Duhamel, and M E Goldberg. Oculocentric spatial representation in parietal cortex. Cerebral Cortex, 5:470–481, 1995.
- S Coombes and M R Owen. Evans functions for integral neural field equations with Heaviside firing rate function. SIAM Journal on Applied Dynamical Systems, 34:574–600, 2004.
- S Coombes and M R Owen. Bumps, breathers, and waves in a neural network with spike frequency adaptation, Physical Review Letters, 94, 148102, 2005.
- G B Ermentrout and J D Cowan. A mathematical theory of visual hallucination patterns. Biological Cybernetics, 34:137–150, 1979.
- G B Ermentrout and J B McLeod. Existence and uniqueness of travelling waves for a neural network. Proceedings of the Royal Society of Edinburgh, 123A:461–478, 1993.
- G B Ermentrout and D Kleinfeld. Traveling electrical waves in cortex: Insights from phase dynamics and speculation on a computational role. Neuron, 29:33–44, 2001.
- J Evans. Nerve axon equations: IV The stable and unstable impulse. Indiana University Mathematics Journal, 24:1169–1190, 1975.
- C P Fall, T J Lewis and J Rinzel. Background-activity-dependent properties of a network model for working memory that incorporates cellular bistability. Biological Cybernetics, 93:109-18, 2004.
- O Faugeras, J Touboul and B Cessac. A constructive mean field analysis of multi population neural networks with random synaptic weights and stochastic inputs. Frontiers of Computational Neuroscience, 3:1, 2009
- S E Folias and P C Bressloff. Breathing pulses in an excitatory neural network. SIAM J. Appl. Dyn. Syst., 3:378-407, 2004.
- J S Griffith. A field theory of neural nets: I: Derivation of field equations. Bulletin of Mathematical Biophysics, 25:111–120, 1963.
- J S Griffith. A field theory of neural nets: II: Properties of field equations. Bulletin of Mathematical Biophysics, 27:187–195, 1965.
- P S Goldman-Rakic. Cellular basis of working memory. Neuron, 14:477–485, 1995.
- D Golomb and Y Amitai. Propagating neuronal discharges in neocortical slices: Computational and experimental study. Journal of Neurophysiology, 78:1199–1211, 1997.
- D H Hubel and T N Wiesel. Receptive Fields, Binocular Interaction And Functional Architecture In The Cat's Visual Cortex. Journal of Physiology 160:106–154, 1962
- V K Jirsa and H Haken. A derivation of a macroscopic field theory of the brain from the quasi-microscopic neural dynamics. Physica D, 99:503–526, 1997.
- U Kim, T Bal, and D A McCormick. Spindle waves are propagating synchronized oscillations in the ferret LGNd in vitro. Journal of Neurophysiology, 74:1301–1323, 1995.
- K Kishimoto and S Amari. Existence and stability of local excitations in homogeneous neural fields. Journal of Mathematical Biology, 7:303–318, 1979.
- C R Laing. Spiral waves in nonlocal equations. SIAM Journal on Applied Dynamical Systems, 4:588-606, 2005.
- C R laing and C C Chow. Stationary bumps in networks of spiking neurons. Neural Comput., 13:1473–1494, 2001.
- C R Laing and W C Troy. PDE methods for nonlocal models. SIAM Journal on Applied Dynamical Systems, 2:487–516, 2003.
- P Tass. Cortical pattern formation during visual hallucinations. Journal of Biological Physics, 21:177–210, 1995.
- R Miles, R D Traub, and R K S Wong. Spread of synchronous firing in longitudinal slices from the CA3 region of Hippocampus. Journal of Neurophysiology, 60:1481–1496, 1995.
- P L Nunez. The brain wave equation: a model for the EEG. Mathematical Biosciences, 21:279–297, 1974.
- C C Petersen. The functional organization of the barrel cortex. Neuron 56(2):339-55, 2007.
- D J Pinto and G B Ermentrout. Spatially structured activity in synaptically coupled neuronal networks: I. Travelling fronts and pulses. SIAM Journal on Applied Mathematics, 62:206–225, 2001.
- K A Richardson, S J Schiff, and B J Gluckman. Control of traveling waves in the mammalian cortex. Physical Review Letters, 94:028103, 2005.
- J A Saez, J Paniagua, I Dominguez & J M Ferrer. Image processing in the primary visual cortex. Review Neurology 26(151):439-44, 1998.
- D C Somers, S B Nelson and M Sur. An emergent model of orientation selectivity in cat visual cortical simple cells. Journal of Neuroscience 15(8), 5448-5465, 1995
- H R Wilson and J D Cowan. Excitatory and inhibitory interactions in localized populations of model neurons. Biophysical Journal, 12:1–24, 1972.
- H R Wilson and J D Cowan. A mathematical theory of the functional dynamics of cortical and thalamic nervous tissue. Kybernetik, 13:55–80, 1973.
- J Y Wu, L Guan, and Y Tsau. Propagating activation during oscillations and evoked responses in neocortical slices. Journal of Neuroscience, 19:5005–5015, 1999.
- K Zhang. Representation of spatial orientation by the intrinsic dynamics of the head-direction cell ensemble: a theory. Journal of Neuroscience, 16:2112–2126, 1996.
Internal references
- Yuri A. Kuznetsov (2006) Andronov-Hopf bifurcation. Scholarpedia, 1(10):1858.
- John W. Milnor (2006) Attractor. Scholarpedia, 1(11):1815.
- Eugene M. Izhikevich (2006) Bursting. Scholarpedia, 1(3):1300.
- Philip Holmes and Eric T. Shea-Brown (2006) Stability. Scholarpedia, 1(10):1838.
- S. Murray Sherman (2006) Thalamus. Scholarpedia, 1(9):1583.
External links
Recent review articles
Recommended reading
- Electric Fields of the Brain: The Neurophysics of EEG, by Paul L. Nunez and Ramesh Srinivasan, Oxford University Press, 2006.