“Lumpability”的版本间的差异
第357行: | 第357行: | ||
===Quasi Lumpability=== | ===Quasi Lumpability=== | ||
− | 我们可以看到,strong lumpability这种对于马尔科夫矩阵的分块性是一个很严格的要求。而我们在现实情况中,很难会找到strongly lumpable的矩阵,稍微加了一点扰动就不行了。如果我们考虑了这种扰动的情况,我们就可以定义不是lumpable但非常接近lumpable的情况:quasi | + | 我们可以看到,strong lumpability这种对于马尔科夫矩阵的分块性是一个很严格的要求。而我们在现实情况中,很难会找到strongly lumpable的矩阵,稍微加了一点扰动就不行了。如果我们考虑了这种扰动的情况,我们就可以定义不是lumpable但非常接近lumpable的情况:quasi<ref name=":5">Franceschinis, Giuliana, and Richard R. Muntz. "Bounds for quasi-lumpable Markov chains." Performance Evaluation 20.1-3 (1994): 223-243.</ref> |
− | |||
− | <ref name=": | ||
如果一个 <math>P</math>可以被分解为<math>P = P^- + P^{\epsilon}</math>,其中<math>P^-</math>是一个lumpable的矩阵,而<math>P^{\epsilon}</math>中的每个元素都小于<math>\epsilon</math>,<math>P</math>就是一个<math>\epsilon</math>-quasi-lumpable的矩阵。 | 如果一个 <math>P</math>可以被分解为<math>P = P^- + P^{\epsilon}</math>,其中<math>P^-</math>是一个lumpable的矩阵,而<math>P^{\epsilon}</math>中的每个元素都小于<math>\epsilon</math>,<math>P</math>就是一个<math>\epsilon</math>-quasi-lumpable的矩阵。 | ||
第401行: | 第399行: | ||
===Proportional Lumpability=== | ===Proportional Lumpability=== | ||
+ | |||
+ | Proportional lumpability<ref name=":6">Piazza, Carla, and Sabina Rossi. "Reasoning about proportional lumpability." International Conference on Quantitative Evaluation of Systems. Cham: Springer International Publishing, 2021.</ref>是基于连续时间马尔科夫链CTMC(Continuoous-time markov chain)的设定提出来的一个放松lumpability限制的概念。 | ||
==Lumpability和粗粒化== | ==Lumpability和粗粒化== |
2024年12月22日 (日) 17:06的版本
Lumpability是一种描述马尔科夫链的状态是否可聚类的性质,笔者暂时还没找到一个正式的中文翻译。
这个概念最早出现在Kemeny, Snell在1969年的Finite Markov Chains[1]中。
最初定义
为了严谨的介绍这一定义,我们会详细翻译文章中的部分。不感兴趣的读者可以跳到更简洁直观的‘lumpable partition的充分必要条件'部分。
首先定义[math]\displaystyle{ s^{(t)} }[/math]表示系统在[math]\displaystyle{ t }[/math]时刻处于各微观状态的概率,微观状态空间为[math]\displaystyle{ S=\{s_1, s_2, ... ,s_n\} }[/math]。
给定一个任意状态划分(state partition) [math]\displaystyle{ A=\{A_1, A_2, ... ,A_r\} }[/math],也可以把其理解为宏观的状态空间。[math]\displaystyle{ S }[/math] 和 [math]\displaystyle{ A }[/math] 之间的硬性划分映射关系[math]\displaystyle{ \Phi:S \rightarrow A }[/math]为:[math]\displaystyle{ A_i \in S, A_i \neq \empty, A_i \cap A_j = \empty , \forall i, j, \cup_i A_i = S }[/math]。这种映射关系是指:[math]\displaystyle{ A }[/math]中的每个元素[math]\displaystyle{ A_i }[/math]都包括了若干个[math]\displaystyle{ s_i }[/math];[math]\displaystyle{ A_i }[/math]和[math]\displaystyle{ A_j }[/math]之间没有交集,即每个[math]\displaystyle{ s_i }[/math]不会同时属于[math]\displaystyle{ A_i }[/math]和[math]\displaystyle{ A_j }[/math];[math]\displaystyle{ S }[/math]中的每个元素必须属于某个[math]\displaystyle{ A }[/math]的元素,即[math]\displaystyle{ A }[/math]覆盖了[math]\displaystyle{ S }[/math]。
在这里,我们考虑的是线性的马尔科夫过程的投影,所以[math]\displaystyle{ \Phi }[/math]是一个[math]\displaystyle{ n \times n }[/math]的投影矩阵。因此,我们可以定义宏观状态[math]\displaystyle{ A^{(t)} = s^{(t)} \Phi }[/math]为微观状态[math]\displaystyle{ s^{(t)} }[/math]在宏观空间上的投影。
对于任意状态划分 [math]\displaystyle{ A }[/math],我们能够定义一个lumped process,即把微观的动力学轨迹[math]\displaystyle{ s^{(t)} }[/math]通过投影矩阵[math]\displaystyle{ \Phi }[/math]投影到[math]\displaystyle{ A }[/math]的空间上。
比如,我们有某条微观状态序列[math]\displaystyle{ \{ s^{(1)}, ..., s^{(6)} \} }[/math] 是 [math]\displaystyle{ \{ s_1, s_3, s_4, s_2, s_2, s_3 \} }[/math],对于投影矩阵[math]\displaystyle{ \Phi = \left ( \begin{array}{cc} 1 & 0 \\ 1 & 0 \\ 0 & 1 \\ 0 & 1 \end{array} \right ) }[/math],我们得到的投影后的宏观状态序列为[math]\displaystyle{ \{ A_1, A_2, A_2, A_1, A_1, A_2 \} }[/math]
定义1:Lumped process
Lumped process,是微观轨迹在宏观空间上的投影。这种投影可以写作下列公式:
-
[math]\displaystyle{ Pr_{\pi}[s^{(0)} \in A_i] }[/math]
(3.1)
-
[math]\displaystyle{ Pr_{\pi}[s^{(1)} \in A_j | s^{(0)} \in A_i] }[/math]
(3.2)
-
[math]\displaystyle{ Pr_{\pi}[s^{(t)} \in A_m | s^{(t-1)} \in A_k, ... , s^{(1)} \in A_j, s^{(0)} \in A_i] }[/math]
(3.3)
其中,[math]\displaystyle{ \pi }[/math]为初始微观状态。
这些公式描述了:
- 式子3.1:系统在[math]\displaystyle{ t=0 }[/math]的微观状态[math]\displaystyle{ s^{(0)} }[/math]属于某个宏观状态[math]\displaystyle{ A_i }[/math]的概率;
- 式子3.2:已知在[math]\displaystyle{ t=0 }[/math]的微观状态[math]\displaystyle{ s^{(0)} }[/math]属于某个宏观状态[math]\displaystyle{ A_i }[/math],那么在时间[math]\displaystyle{ t=1 }[/math]的微观状态[math]\displaystyle{ s^{(1)} }[/math]属于某个宏观状态[math]\displaystyle{ A_j }[/math]的概率;
- 式子3.3:已知历史微观状态[math]\displaystyle{ \{s^{(0)}, ... , s^{(t-1)} \} }[/math]对应的宏观状态,那么在时间[math]\displaystyle{ t }[/math]的微观状态[math]\displaystyle{ s^{(t)} }[/math]属于某个宏观状态[math]\displaystyle{ A_m }[/math]的概率;
回想一下图(1)中 微观状态->微观动力学->粗粒化->宏观状态 的这一条路径。我们现在有了微观动力学[math]\displaystyle{ \{s^{(0)}, ... , s^{(t-1)} \} }[/math],也有了微观到宏观的粗粒化过程[math]\displaystyle{ A }[/math]。所以式子(3)描述的lumped process正是先走微观动力学再聚合的这条路径的[math]\displaystyle{ A^{(t)} }[/math]。它描述了我们从任意一个初始状态[math]\displaystyle{ s^{(0)} }[/math](或状态分布[math]\displaystyle{ \pi }[/math]),一直走微观动力学,然后在[math]\displaystyle{ t }[/math]时刻做粗粒化得到[math]\displaystyle{ A^{(t)} }[/math]的过程。
需要注意的是,虽然任意一个状态划分 [math]\displaystyle{ A }[/math]都能走这样的路径,并形成lumped process,但不是所有的这些lumped process都是有意义且满足交换律的。他们不一定能够得出一个宏观动力学来描述式子(3)中微观状态的宏观投影,也不一定会拥有马尔科夫性。
我们暂且先不考虑‘失败’的情况,先关注‘成功’的部分。书中接下来就定义了什么叫做lumpable 划分:
定义2:lumpable partition 划分
对于一个给定的状态 [math]\displaystyle{ A }[/math],当下列两个条件都满足时,[math]\displaystyle{ A }[/math]是一个lumpable partition 划分。
- 定义1中式子(3)描述的lumped process具有马尔科夫性,即式子(3.3)可写成[math]\displaystyle{ Pr_{\pi} \left [s^{(t)} \in A_m | s^{(t-1)} \in A_k, ... , s^{(1)} \in A_j, s^{(0)} \in A_i \right ] = Pr_{\pi} \left [s^{(t)} \in A_m | s^{(t-1)} \in A_k \right ] }[/math]
- 转移概率(Transition probability),即式子(3.3),对任何微观初始状态(starting vector) [math]\displaystyle{ \pi }[/math] 都保持一致
不满足上述两个条件的,都不被定义为lumpable partition。也就是说,不是所有的lumped process都是lumpable的,即使他们的命名方式相似。
从图3的lumpable例子中,我们看到对于分组矩阵[math]\displaystyle{ \Phi = \left ( \begin{array}{cc} 1 & 0 \\ 1 & 0 \\ 0 & 1 \\ 0 & 1 \end{array} \right ) }[/math],lumpable的动力学TPM可以找到一个lumped process,而这个lumped process是满足马尔可夫性,且对所有初始状态都保持一致的。也就是说,无论[math]\displaystyle{ s^{(t)} }[/math]是什么,[math]\displaystyle{ \{ s^{(t)}, s^{(t+1)} \} }[/math]投影到宏观空间上的[math]\displaystyle{ \{ A^{(t)}, A^{(t+1)} \} }[/math]的[math]\displaystyle{ P( A^{(t+1)} | A^{(t)} ) }[/math]都保持一致。
对于例子中的non-lumpable矩阵,我们或许可以给它强制定义一个lumped process的TPM,比如[math]\displaystyle{ P_1' = \left ( \begin{array}{cc} 0.6 & 0.4 \\ 0.2 & 0.8 \\ \end{array} \right ) }[/math],但这个lumped process并不能对任何微观初始状态保持一致,只能对[math]\displaystyle{ s^{(t)} = \pi = \left [ \begin{array}{cc}1 & 0 & 0 & 0 \end{array} \right ] }[/math]适用。
而[math]\displaystyle{ s^{(t)} = \pi = \left [ \begin{array}{cc}0 & 1 & 0 & 0 \end{array} \right ] }[/math]不适用这个lumped process。它适用的是[math]\displaystyle{ P_2' = \left ( \begin{array}{cc} 0.5 & 0.5 \\ 0.2 & 0.8 \\ \end{array} \right ) }[/math]。这里的不适用是指,这个lumped process不能描述这个[math]\displaystyle{ \pi }[/math]为初始状态出发的微观状态序列投影的宏观状态序列。
我们看到,对于这个分组矩阵[math]\displaystyle{ \Phi = \left ( \begin{array}{cc} 1 & 0 \\ 1 & 0 \\ 0 & 1 \\ 0 & 1 \end{array} \right ) }[/math],马尔科夫矩阵有两个lumped process [math]\displaystyle{ P_1' }[/math]和[math]\displaystyle{ P_2' }[/math],而它们各自只适用于一些微观初始状态(starting vector) [math]\displaystyle{ \pi }[/math],并不满足定义2中的条件,所以[math]\displaystyle{ A }[/math]对于这个TPM来说不是一个lumpable partition。事实上,这个TPM只存在一个lumpable partition,就是不做任何的分组,所以它无法按照lumpability的定义进行粗粒化。
lumpable partition的充分必要条件
通过上面的例子,我们可以对lumpable partition有一些直观的理解。我们可以留意到,在lumpable partition中,有两个分组,所以有四个框。每一个框里,每一列相加的和都是一样的,比如左上角的框中,[math]\displaystyle{ 0.3 + 0.3 = 0.2 + 0.4 }[/math]。这就是lumpable partition更通俗的解释,在某些文献中,并不会使用上面提及的定义,而是直接使用这个通俗版的解释。[1]中作者指出,这两者之间是存在充分必要条件关系的。
设[math]\displaystyle{ p_{s_k \rightarrow s_m} = p(s^{(t)} = s_m | s^{(t-1)} = s_k) }[/math],[math]\displaystyle{ p_{s_k \rightarrow A_i} = p(s^{(t)} \in A_i | s^{(t-1)} = s_k) = \sum_{s_m \in A_i} p_{s_k \rightarrow s_m} }[/math],[math]\displaystyle{ p_{A_i \rightarrow A_j} = p(A^{(t)} = A_j | A^{(t-1)} = A_i) }[/math]
作者提出了判断一个马尔科夫链对给定partition [math]\displaystyle{ A }[/math] 是否lumpable的充分必要条件为:
定理1:
给定partition [math]\displaystyle{ A }[/math]是lumpable的充分必要条件为:
对于任意一对[math]\displaystyle{ A_i, A_j }[/math],每一个属于[math]\displaystyle{ A_i }[/math]的状态[math]\displaystyle{ s_k }[/math]的[math]\displaystyle{ p_{s_k \rightarrow A_j} }[/math]都是一样的。
顺着这个条件,我们也能对lumpable partition,定义宏观动力学[math]\displaystyle{ \{A^{(0)}, ... , A^{(t-1)} \} }[/math]了:
定义3:lumpability的宏观动力学
对于给定的微观状态[math]\displaystyle{ S }[/math],微观动力学[math]\displaystyle{ p_{s_k \rightarrow s_m} }[/math]和lumpable partition [math]\displaystyle{ A }[/math],宏观动力学[math]\displaystyle{ p_{A_i \rightarrow A_j} }[/math]等于[math]\displaystyle{ A_i }[/math]中任意状态[math]\displaystyle{ s_k }[/math]到[math]\displaystyle{ A_j }[/math]的概率:
-
[math]\displaystyle{ \begin{aligned} p_{A_i \rightarrow A_j} = p_{s_k \rightarrow A_j} = \sum_{s_m \in A_j} p_{s_k \rightarrow s_m}, k \in A_i \end{aligned} }[/math]
(4)
这个公式表达的是,群组[math]\displaystyle{ A_i }[/math]到群组[math]\displaystyle{ A_j }[/math]的转移概率 = 群组[math]\displaystyle{ A_i }[/math]中任意状态[math]\displaystyle{ s_k }[/math]到群组[math]\displaystyle{ A_j }[/math]的转移概率 = 群组[math]\displaystyle{ A_i }[/math]中任意状态[math]\displaystyle{ s_k }[/math]到群组[math]\displaystyle{ A_j }[/math]中的所有状态的转移概率的和。
对于lumpable partition [math]\displaystyle{ A }[/math]定义宏观动力学后,我们就能走另外一条路径了:微观状态->粗粒化->宏观动力学->宏观状态。
必要性部分的证明:
必要性是指,如果一个马尔可夫链的partition [math]\displaystyle{ A }[/math]是lumpable,那么就肯定会满足上述的这个特定条件。
从lumpable partition 的定义可知,[math]\displaystyle{ A }[/math]的转移概率满足宏观马尔可夫性,且这个转移概率[math]\displaystyle{ Pr_{\pi} [ s^{(1)} \in A_j | s^{(0)} \in A_i ] }[/math]对每个[math]\displaystyle{ \pi }[/math]保持一致,即在[math]\displaystyle{ t=0 }[/math]时无论从哪个具体的初始状态[math]\displaystyle{ \pi }[/math]开始,只要它属于[math]\displaystyle{ A_i }[/math]这个群组里,在[math]\displaystyle{ t=1 }[/math]时刻转移到[math]\displaystyle{ A_j }[/math]的概率都相同。
我们设这个([math]\displaystyle{ A_i }[/math]中任意微观状态[math]\displaystyle{ s_k }[/math]的)共同的转移概率为[math]\displaystyle{ \hat{p}_{A_i \rightarrow A_j} }[/math]。当初始状态[math]\displaystyle{ \pi }[/math]属于某个[math]\displaystyle{ A_i }[/math]时([math]\displaystyle{ \pi \in A_i }[/math]),[math]\displaystyle{ p_{s_k \rightarrow A_j} = Pr_{\pi \in A_i} [ s^{(1)} \in A_j ] = \hat{p}_{A_i \rightarrow A_j} }[/math]。
所以,这说明了,如果一个马尔可夫链的lumped process满足lumpability,那么从任一集合到另一集合的转移概率必须是集合间的固定值,而与集合内的具体状态(不管是[math]\displaystyle{ \pi }[/math]还是[math]\displaystyle{ s_k }[/math])无关。这个固定值就是式子(4)定义的宏观状态转移概率,所以满足了该条件。
充分性部分的证明:
充分性是指,如果一个马尔可夫链的某个partition [math]\displaystyle{ A }[/math]满足这个条件,那么它就是lumpable。我们要证明的是,如果上述条件满足,那么马尔可夫性成立,即对于任何给定的[math]\displaystyle{ t }[/math],转移概率[math]\displaystyle{ Pr \left [ s^{(t)} \in A_j | s^{(t-1)} \in A_i, \ldots, s^{(1)} \in A_k, s^{(0)} \in A_m \right ] }[/math]只依赖于[math]\displaystyle{ A_i }[/math]和[math]\displaystyle{ A_j }[/math],而不依赖于[math]\displaystyle{ A_i }[/math]中的具体状态或是[math]\displaystyle{ t-1 }[/math]前的宏观状态。
条件要求所有[math]\displaystyle{ s_k \in A_i }[/math],转移概率[math]\displaystyle{ p_{s_k \rightarrow A_j} = p_{A_i \rightarrow A_j} }[/math],这意味着无论我们从[math]\displaystyle{ A_i }[/math]中的哪个微观状态转移过来,转移到[math]\displaystyle{ A_j }[/math]的概率都是相同的。
因此,当我们知道[math]\displaystyle{ t-1 }[/math]的宏观状态是[math]\displaystyle{ A_i }[/math]时,我们计算[math]\displaystyle{ t }[/math]时刻转移到[math]\displaystyle{ A_j }[/math]的概率时,只需要用[math]\displaystyle{ p_{A_i \rightarrow A_j} }[/math],而不需要关心[math]\displaystyle{ A_i }[/math]中的具体状态。
由于转移概率只依赖于群组[math]\displaystyle{ A_i }[/math]和[math]\displaystyle{ A_j }[/math],而不依赖于[math]\displaystyle{ A_i }[/math]中的具体状态,因此我们可以将[math]\displaystyle{ A_i }[/math]中的所有状态合并为一个状态,并且这个合并后的链仍满足马尔可夫性。所以,上述条件为lumpability的充分条件。
从这两个证明里,我们也能看到微观动力学的路径和宏观动力学的路径的一致性。无论我们从[math]\displaystyle{ A_i }[/math]中的哪个具体状态[math]\displaystyle{ s^{(t-1)} }[/math]开始,只要我们知道当前的宏观状态是[math]\displaystyle{ A_i }[/math],我们就可以计算下一步转移到[math]\displaystyle{ A_j }[/math]的概率,而不依赖于[math]\displaystyle{ s^{(t-1)} }[/math]。这表明,微观[math]\displaystyle{ s^{(t-1)} }[/math]->宏观[math]\displaystyle{ A^{(t-1)} }[/math]->宏观动力学->[math]\displaystyle{ A^{(t)} }[/math]不会影响未来状态的预测,因此从[math]\displaystyle{ s^{(t-1)} }[/math]出发的两个路径得出的[math]\displaystyle{ A^{(t)} }[/math]的概率是一致的。
请注意:lumpability有一个非常重要的前提,即我们要给定一个对马尔科夫链的state的partition,然后根据马尔科夫状态转移矩阵来决定这个partition对这个马尔科夫链是否lumpable。
所以,lumpability并不是一个马尔科夫链的特质,而是对马尔科夫链的某个state partition的特质。我们不能直接说某个马尔科夫链是lumpable或non-lumpable,但是我们可以说,对于某个马尔科夫链的某几个状态partition是lumpable或non-lumpable。
Lumpability正例和反例
对于一个马尔科夫链,有些分组partition是lumpable,有些分组是non-lumpable。这里用一个简单的例子提供正例和反例。
我们用到的是这样一个转移矩阵:
[math]\displaystyle{ \begin{aligned} P = P_{s_i \rightarrow s_j} = \left [ \begin{array}{ccc:c} 0.3 & 0.3 & 0.3 & 0.1 \\ 0.3 & 0.3 & 0.3 & 0.1 \\ 0.3 & 0.3 & 0.3 & 0.1 \\ \hdashline0.1 & 0.1 & 0.1 & 0.7 \end{array} \right ] \end{aligned} }[/math]
正例
我们可以看到,123行都是一样的,所以把他们[math]\displaystyle{ \{s_1, s_2, s_3\} }[/math]分在一组[math]\displaystyle{ A_1 }[/math],并把状态[math]\displaystyle{ s_4 }[/math]分在另外一组[math]\displaystyle{ A_2 }[/math],看起来像是一个合理的正例。
接下来我们来计算一下。
设[math]\displaystyle{ p_{s_1 \rightarrow s_2} = p(s^{(t)} = s_2 | s^{(t-1)} = s_1) }[/math],[math]\displaystyle{ p_{s_1 \rightarrow A_2} = p(s^{(t)} \in A_2 | s^{(t-1)} = s_1) }[/math]
第1组[math]\displaystyle{ A_1 }[/math]的状态[math]\displaystyle{ s_1 }[/math]到第1组[math]\displaystyle{ A_1 }[/math],[math]\displaystyle{ p_{s_1 \rightarrow A_1} = p_{A_1 \rightarrow A_1} = \sum_{s_m \in A_1} p_{s_1 \rightarrow s_m} = p_{s_1 \rightarrow s_1} + p_{s_1 \rightarrow s_2} + p_{s_1 \rightarrow s_3} = 0.3 \times 3 = 0.9 }[/math]
第1组[math]\displaystyle{ A_1 }[/math]的状态[math]\displaystyle{ s_1 }[/math]到第2组[math]\displaystyle{ A_2 }[/math],[math]\displaystyle{ p_{s_1 \rightarrow A_2} = p_{A_1 \rightarrow A_2} = \sum_{s_m \in A_2} p_{s_1 \rightarrow s_m} = p_{s_1 \rightarrow s_4} = 0.1 }[/math]
第2组[math]\displaystyle{ A_2 }[/math]的状态[math]\displaystyle{ s_4 }[/math]到第1组[math]\displaystyle{ A_1 }[/math],[math]\displaystyle{ p_{s_4 \rightarrow A_1} = p_{A_2 \rightarrow A_1} = \sum_{s_m \in A_1} p_{s_4 \rightarrow s_m} = p_{s_4 \rightarrow s_1} + p_{s_4 \rightarrow s_2} + p_{s_4 \rightarrow s_3} = 0.1 \times 3 = 0.3 }[/math]
第2组[math]\displaystyle{ A_2 }[/math]的状态[math]\displaystyle{ s_4 }[/math]到第2组[math]\displaystyle{ A_2 }[/math],[math]\displaystyle{ p_{s_4 \rightarrow A_2} = p_{A_2 \rightarrow A_2} = \sum_{s_m \in A_2} p_{s_4 \rightarrow s_m} = p_{s_4 \rightarrow s_4} = 0.7 }[/math]
[math]\displaystyle{ \begin{aligned} P = P_{A_k \rightarrow A_l} = \left [ \begin{array}{c:c} 0.9 & 0.1 \\ \hdashline0.3 & 0.7 \end{array} \right ] \end{aligned} }[/math]
反例
现在我们来看一个反例:把状态12分为一组,状态34分为另一组,
同样的,我们来计算一下。这次我们需要对3和4分开计算了。
第1组[math]\displaystyle{ A_1 }[/math]的状态[math]\displaystyle{ s_1 }[/math]到第1组[math]\displaystyle{ A_1 }[/math],[math]\displaystyle{ p_{s_1 \rightarrow A_1} = p_{A_1 \rightarrow A_1} = \sum_{s_m \in A_1} p_{s_1 \rightarrow s_m} = p_{s_1 \rightarrow s_1} + p_{s_1 \rightarrow s_2} = 0.3 \times 2 = 0.6 }[/math]
第1组[math]\displaystyle{ A_1 }[/math]的状态[math]\displaystyle{ s_1 }[/math]到第2组[math]\displaystyle{ A_2 }[/math],[math]\displaystyle{ p_{s_1 \rightarrow A_2} = p_{A_1 \rightarrow A_2} = \sum_{s_m \in A_2} p_{s_1 \rightarrow s_m} = p_{s_1 \rightarrow s_3} + p_{s_1 \rightarrow s_4} = 0.4 }[/math]
第2组[math]\displaystyle{ A_2 }[/math]的状态[math]\displaystyle{ s_3 }[/math]到第1组[math]\displaystyle{ A_1 }[/math],[math]\displaystyle{ p_{s_3 \rightarrow A_1} = \sum_{s_m \in A_1} p_{s_3 \rightarrow s_m} = p_{s_3 \rightarrow s_1} + p_{s_3 \rightarrow s_2} = 0.3 \times 2 = 0.6 }[/math]
第2组[math]\displaystyle{ A_2 }[/math]的状态[math]\displaystyle{ s_4 }[/math]到第1组[math]\displaystyle{ A_1 }[/math],[math]\displaystyle{ p_{s_4 \rightarrow A_1} = \sum_{s_m \in A_1} p_{s_4 \rightarrow s_m} = p_{s_4 \rightarrow s_1} + p_{s_4 \rightarrow s_2} = 0.1 \times 2 = 0.2 }[/math]
这里我们就能看到,[math]\displaystyle{ p_{s_3 \rightarrow A_1} \neq p_{s_4 \rightarrow A_1} }[/math],当同一组的两个状态[math]\displaystyle{ s_3 }[/math]和[math]\displaystyle{ s_4 }[/math]对其他组的转移概率不一样的话,如果我们强行按照这样来分组(也没办法强行,因为我们不知道[math]\displaystyle{ p_{A_2 \rightarrow A_1} = p_{s_3 \rightarrow A_1} }[/math]还是[math]\displaystyle{ p_{A_2 \rightarrow A_1} = p_{s_4 \rightarrow A_1} }[/math]),假设[math]\displaystyle{ p_{A_2 \rightarrow A_1} = 0.6 }[/math],我们会发现这样的粗粒化结果违背了Lumpability一开始的定义[1](公式(3)),即粗粒化后的转移概率对所有的初始微观状态[math]\displaystyle{ \pi }[/math]都适用。因为当[math]\displaystyle{ \pi = s_4 }[/math]的时候,[math]\displaystyle{ p_{A_2 \rightarrow A_1} = 0.6 }[/math]这个转移概率就是错的,即使[math]\displaystyle{ \pi \neq s_4 }[/math],任何初始微观状态[math]\displaystyle{ \pi }[/math]都会在某时刻[math]\displaystyle{ n }[/math]达到[math]\displaystyle{ s_4 }[/math]并导致转移概率出错。
一个更复杂的正例
三个相同状态的例子可能稍微有点简单,接下来我们来看一个稍微复杂一点的例子:
[math]\displaystyle{ \begin{aligned} P = P_{s_i \rightarrow s_j} = \left [ \begin{array}{cc:cc} 0.2 & 0.4 & 0.3 & 0.1 \\ 0.4 & 0.2 & 0.1 & 0.3 \\ \hdashline0.2 & 0.1 & 0.2 & 0.5 \\ 0.1 & 0.2 & 0 & 0.7 \end{array} \right ] \end{aligned} }[/math]
对于这个马尔科夫矩阵,[math]\displaystyle{ \{\{1,2\}, \{3,4\}\} }[/math]是一个lumpable的分组。分组后的转移矩阵为: [math]\displaystyle{ \begin{aligned} P = P_{A_k \rightarrow A_l} = \left [ \begin{array}{c:c} 0.6 & 0.4 \\ \hdashline0.3 & 0.7 \end{array} \right ] \end{aligned} }[/math]
Lumpability概念的变种
Strong/Ordinary Lumpability
Strong lumpability 或 ordinary lumpability 是上面所述的lumpability的原本概念,是这六个变种中约束最强的一种。它要求矩阵具有严格的分块性。
这里的'Strong'是与下面的'weak'相对的概念,指上文的lumped process满足对任意初始状态分布都保持一致的条件。
简单的例子:
[math]\displaystyle{ \begin{aligned} P = P_{s_i \rightarrow s_j} = \left [ \begin{array}{cc:cc} 0.2 & 0.4 & 0.3 & 0.1 \\ 0.4 & 0.2 & 0.1 & 0.3 \\ \hdashline0.2 & 0.1 & 0.2 & 0.5 \\ 0.1 & 0.2 & 0 & 0.7 \end{array} \right ] \end{aligned} }[/math]
此例子中,[math]\displaystyle{ [[1, 2], [3, 4]] }[/math]是一个strongly lumpable分组。
Weak Lumpability
Weak lumpability[2]是指lumped process不对所有的初始状态满足,而仅对某些初始状态满足。
一个简单的例子是,某个状态[math]\displaystyle{ s_i }[/math]到[math]\displaystyle{ s_j }[/math]不联通的,而lumped process适用于[math]\displaystyle{ s_i }[/math]但不适用于[math]\displaystyle{ s_j }[/math]。所以,当初始状态等于[math]\displaystyle{ s_i }[/math]时,因为永远不会到达[math]\displaystyle{ s_j }[/math],所以lumped process会一直适用。
简单的例子:
[math]\displaystyle{ \begin{aligned} P = P_{s_i \rightarrow s_j} = \left [ \begin{array}{cc:cc} 0.6 & 0.4 & 0.0 & 0.0 \\ 0.3 & 0.7 & 0.0 & 0.0 \\ \hdashline 0.2 & 0.1 & 0.2 & 0.5 \\ 0.6 & 0.1 & 0.1 & 0.1 \end{array} \right ] \end{aligned} }[/math]
此例子中,[math]\displaystyle{ [[1, 2], [3], [4]] }[/math]是一个strongly lumpable分组,而[math]\displaystyle{ [[1, 2], [3, 4]] }[/math]是一个weakly lumpable分组,
Exact Lumpability
在strong lumpability中,[math]\displaystyle{ P }[/math]分组内每一行的加总是一样的,也就是说,同一组中的每个状态到另一个分组的转出概率是相同的。
而在exact lumpability中,[math]\displaystyle{ P }[/math]分组内的每一列的加总是一样的,也就是说,同一组中的每个状态从另一个分组的转入概率是相同的。
在[3]中给出的正式定义是,[math]\displaystyle{ P }[/math]的转置经过行归一化是strong lumpable的。
另外,[3]说明了exactly lumpable的[math]\displaystyle{ P }[/math]也是weakly lunpable的。
在Strong lumpability例子中,[math]\displaystyle{ [[1, 2], [3, 4]] }[/math]是一个strongly lumpable分组,但不是一个exactly lumpable分组。
我们再举一个例子:
[math]\displaystyle{ \begin{aligned} P = P_{s_i \rightarrow s_j} = \left [ \begin{array}{cc:cc} 0.1 & 0.1 & 0.4 & 0.4 \\ 0.4 & 0.4 & 0.1 & 0.1 \\ \hdashline 0.3 & 0.3 & 0.2 & 0.2 \\ 0.2 & 0.2 & 0.3 & 0.3 \end{array} \right ] \end{aligned} }[/math]
此例子中,[math]\displaystyle{ [[1, 2], [3, 4]] }[/math]不是一个strongly lumpable分组,但是一个exactly lumpable分组,
Strict Lumpability
Strict lumpability是指一个马尔可夫链同时满足strong和exact lumpability,也就是说,同一组中的每个状态不仅从另一个分组的转入概率相等,而且到另一个分组的转出概率也相等。
简单的例子:
[math]\displaystyle{ \begin{aligned} P = P_{s_i \rightarrow s_j} = \left [ \begin{array}{cc:cc} 0.4 & 0.2 & 0.1 & 0.3 \\ 0.2 & 0.4 & 0.3 & 0.1 \\ \hdashline 0.1 & 0.3 & 0.4 & 0.2 \\ 0.3 & 0.1 & 0.2 & 0.4 \end{array} \right ] \end{aligned} }[/math]
此例子中,[math]\displaystyle{ [[1, 2], [3, 4]] }[/math]既是一个strongly lumpable分组,又是一个exactly lumpable分组。
Quasi Lumpability
我们可以看到,strong lumpability这种对于马尔科夫矩阵的分块性是一个很严格的要求。而我们在现实情况中,很难会找到strongly lumpable的矩阵,稍微加了一点扰动就不行了。如果我们考虑了这种扰动的情况,我们就可以定义不是lumpable但非常接近lumpable的情况:quasi[4]
如果一个 [math]\displaystyle{ P }[/math]可以被分解为[math]\displaystyle{ P = P^- + P^{\epsilon} }[/math],其中[math]\displaystyle{ P^- }[/math]是一个lumpable的矩阵,而[math]\displaystyle{ P^{\epsilon} }[/math]中的每个元素都小于[math]\displaystyle{ \epsilon }[/math],[math]\displaystyle{ P }[/math]就是一个[math]\displaystyle{ \epsilon }[/math]-quasi-lumpable的矩阵。
比如说,我们对上面的strict lumpability例子加上一点扰动:
[math]\displaystyle{ \begin{aligned} P = P_{s_i \rightarrow s_j} = \left [ \begin{array}{cc:cc} 0.40 & 0.18 & 0.11 & 0.31 \\ 0.21 & 0.42 & 0.29 & 0.08 \\ \hdashline 0.12 & 0.30 & 0.38 & 0.20 \\ 0.30 & 0.08 & 0.22 & 0.40 \end{array} \right ] = \left [ \begin{array}{cc:cc} 0.4 & 0.2 & 0.1 & 0.3 \\ 0.2 & 0.4 & 0.3 & 0.1 \\ \hdashline 0.1 & 0.3 & 0.4 & 0.2 \\ 0.3 & 0.1 & 0.2 & 0.4 \end{array} \right ] + \left [ \begin{array}{cccc} 0.00 & -0.02 & 0.01 & 0.01 \\ 0.01 & 0.02 & -0.01 & -0.02 \\ 0.02 & 0.00 & -0.02 & 0.00 \\ 0.00 & -0.02 & 0.02 & 0.00 \end{array} \right ] \end{aligned} }[/math]
就能获得一个[math]\displaystyle{ \epsilon }[/math]-quasi-lumpable,[math]\displaystyle{ \epsilon=0.02 }[/math]的矩阵。
Proportional Lumpability
Proportional lumpability[5]是基于连续时间马尔科夫链CTMC(Continuoous-time markov chain)的设定提出来的一个放松lumpability限制的概念。
Lumpability和粗粒化
马尔科夫链的粗粒化不仅要对状态空间做,也要对转移矩阵和概率空间做。这三个部分可以同时做,也可以看作为:先对状态空间做,再对转移矩阵和概率空间做;即先对状态做分组,然后再获取对应的粗粒化后的转移矩阵。
对状态空间做粗粒化有硬划分和软划分两种。软划分可以看作把微观状态打散重构成了一些宏观状态,而硬划分则是更严格的,把若干个微观状态分成一个组。
而Lumpability就是一个指标,用来评价‘对于某一种硬划分的微观状态分组分案,是否对微观状态转移矩阵lumpable’。
我们把状态空间按照任意一个硬划分方案做分类,它都有对应后续的对转移矩阵和概率空间的粗粒化方案(公式(1)和(2))[3],其中有些分组满足交换律,有些则不满足。
其中的某些分组方案lumpable,也有某些分组方案non-lumpable。
所以,整个链条应该是这样的:
所以,一个满足lumpability的良定义的粗粒化方案应该有四个条件:
- 粗粒化后的[math]\displaystyle{ S' }[/math]和[math]\displaystyle{ T' }[/math]要符合马尔科夫链定义;
- 满足交换律;
- Hard Partitioning,即存在分组矩阵[math]\displaystyle{ V_{i, j} = 1\ if\ s_i \in s_j'\ and\ 0\ otherwise }[/math];
- [math]\displaystyle{ V }[/math]是一个lumpable分组。
Lumpable Partition粗粒化的一致性Consistency
这一小节里我们讨论一下,对一个马尔科夫链的lumpable partition 与 HOM(Higher-order Macronodes,详情请参考复杂网络中的因果涌现),和Consistency之间的关联。
Higher-order Macronodes,是由Jian Xu[2]提出,根据给定的微观马尔科夫链和节点的聚类方案,得出一个和微观转移矩阵保持一致的宏观转移矩阵的方法。
我们这里使用一个简单的lumpable partition例子,来显示lumpability的粗粒化跟HON是对应的。
[math]\displaystyle{ \begin{aligned} P = P_{s_i \rightarrow s_j} = \left [ \begin{array}{c:c:cc:c:c} 0 & 0 & 0.3 & 0.1 & 0.6 & 0\\ \hdashline 0 & 0 & 0.2 & 0.3 & 0 & 0.5\\ \hdashline 0 & 0 & 0 & 0.7 & 0.2 & 0.1\\ 0 & 0 & 0.7 & 0 & 0.2 & 0.1\\ \hdashline 1.0 & 0 & 0 & 0 & 0 & 0\\ \hdashline 0 & 1.0 & 0 & 0 & 0 & 0\\ \end{array} \right ] \end{aligned} }[/math]
对于这个转移概率矩阵,[math]\displaystyle{ \{1, 2, \{34\}, 5, 6\} }[/math]是一个lumpable partition。
首先,我们先按照lumpability的粗粒化定义,计算对应的宏观转移概率: [math]\displaystyle{ \begin{aligned} \left [ \begin{array}{c:c:c:c:c} 0 & 0 & 0.4 & 0.6 & 0\\ \hdashline 0 & 0 & 0.5 & 0 & 0.5\\ \hdashline 0 & 0 & 0.7 & 0.2 & 0.1\\ \hdashline 1.0 & 0 & 0 & 0 & 0\\ \hdashline 0 & 1.0 & 0 & 0 & 0\\ \end{array} \right ] \end{aligned} }[/math]
然后,我们来简单介绍一下的构建宏观HOM转移概率矩阵的计算方法。
在图4中,我们看到3和4为待合并节点[math]\displaystyle{ A }[/math]。
1. 对于其他节点到待合并节点[math]\displaystyle{ A }[/math]的连边,即待合并节点的入流(图4中绿色的连边),我们直接加和即可,即[math]\displaystyle{ p_{s_1 \rightarrow A} = 0.3+0.1=0.4 }[/math],[math]\displaystyle{ p_{ s_2 \rightarrow A} = 0.2+0.3=0.5 }[/math]
2. 对于待合并节点[math]\displaystyle{ A }[/math]到其他节点的连边,即待合并节点的出流(图4中棕色的连边),我们需要考虑三种情况:
- 当待合并节点[math]\displaystyle{ A }[/math]间存在连边时;
- 当待合并节点[math]\displaystyle{ A }[/math]指向同一个输出节点时;
- 当待合并节点[math]\displaystyle{ A }[/math]指向不同输出节点时;
待合并节点[math]\displaystyle{ A }[/math]的出流的计算方式都不一样,如图5所示。
但是,我们可以注意到,这三种计算方式都是待合并节点[math]\displaystyle{ A }[/math]中的各节点的出流的[math]\displaystyle{ W_i^{out} }[/math]加权,而我们知道,lumpability的特性决定了,群组里的各节点到其他群组的出流[math]\displaystyle{ W_i^{out} }[/math]都是一样的,对相同的[math]\displaystyle{ W_i^{out} }[/math]做任意加权平均的结果都等于[math]\displaystyle{ W_i^{out} }[/math]。也就是说,任意的加权方法对于lumpable partition来说都能得出相同的结果。
由此,我们总结,lumpable partition的聚合方式和HOM的计算方式,无论入流还是出流都完全一致。
我们得出这个结论的目标是,借助HOM方法的consistency的性质,说明lumpable partition的聚合方式,也有consistency的性质。
以下关于网络动力学的一致性的描述节选自复杂网络中的因果涌现:
定义微观网络[math]\displaystyle{ G }[/math]与宏观网络[math]\displaystyle{ G_M }[/math],在两个网络上随机游走,在未来某个时间[math]\displaystyle{ t }[/math] , [math]\displaystyle{ G }[/math]上的预期分布为 [math]\displaystyle{ P_m(t) }[/math], [math]\displaystyle{ G_M }[/math]上的预期分布为 [math]\displaystyle{ P_M(t) }[/math]。
将[math]\displaystyle{ P_m(t) }[/math]分布叠加到宏观上[math]\displaystyle{ G_M }[/math]的相同节点上,得到[math]\displaystyle{ P_{M|m}(t) }[/math]分布。用[math]\displaystyle{ P_M(t) }[/math]和[math]\displaystyle{ P_{M|m}(t) }[/math]之间的KL散度来衡量其不一致性(inconsistency),若结果为零则动力学一致。公式为:
[math]\displaystyle{ inconsistency=\sum_{t=0}^T D_{KL}[P_M(t)||P_{M|m}(t)] }[/math]
也就是说,Inconsistency 等于 宏观和微观网络节点在不同时刻的概率分布的KL散度之和。
实验发现,对不同节点规模以及参数下的微观网络,使用HOM粗粒化后的宏观网络的不一致性随着迭代步数的增加都会收敛到0。这说明,HOM构建的宏观网络和微观网络是保持一致的。
回到上面lumpable partition 等于HOM的推断,我们就能得出,根据lumpability而做的粗粒化,构建的宏观网络和微观网络也是保持一致的。
基于Lumpability的粗粒化方法(未给定lumpable partition的情况)
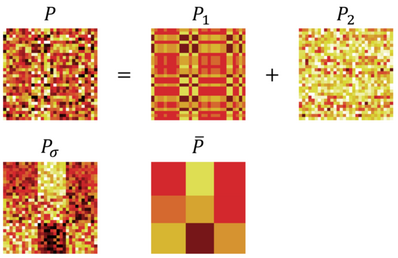
由上面的lumpability公式(4)和例子中我们能获得一个直观上的说法:当马尔科夫矩阵存在block结构,或者状态明显可被分成几类的时候,根据这样的partition,该矩阵就会lumpable,如图6中的[math]\displaystyle{ \bar{P} }[/math]所示,把相同的状态(行向量)分成一类的partition显然lumpable。
但是,有时候有些lumpable的矩阵的状态排序被打乱了(如图6中的[math]\displaystyle{ P_1 }[/math]),或者矩阵包含了如[math]\displaystyle{ P_2 }[/math]的噪声(如图6中的[math]\displaystyle{ P }[/math],[math]\displaystyle{ P = P_1 + P_2 }[/math],[math]\displaystyle{ P_1^TP_2 = 0 }[/math])。
我们在实际问题中很多时候要面对的是像[math]\displaystyle{ P }[/math]这样的矩阵,我们既无法确定它是否lumpable,也无法决定它的partition,我们甚至不知道它的马尔科夫秩。
在这种情况下,Anru Zhang[6][6]的文章中提供了一种寻找最优partition的方法。通过此方法我们能找到最优的partition,代入这个partition我们就能通过上面的充分必要条件来决定一个马尔科夫矩阵是否lumpable。具体步骤如下:
- 先获取一个n*n维的马尔科夫矩阵P,或者是马尔科夫链的频率采样;
- 获取r<n 的马尔科夫秩,或指定一个r;
- 对P进行SVD分解,[math]\displaystyle{ P = U \Sigma V^T }[/math],其中U为左奇异向量,V为右奇异向量。
- 通过下列公式得到最优partition [math]\displaystyle{ \Omega_1, \Omega_2, ... , \Omega_r }[/math]
[math]\displaystyle{ \Omega_1, \Omega_2, ... , \Omega_r = argmin_{\Omega_1, \Omega_2, ... , \Omega_r} min_{v_1, v_2, ... , v_r} \sum_{s=1}^r \sum_{i \in \Omega_s} || V_{[i,:r]} - v_s ||_2^2 }[/math]
其中,[math]\displaystyle{ v_s }[/math]是第[math]\displaystyle{ s }[/math]类[math]\displaystyle{ \Omega_s }[/math]的特征向量。
这个算法的意思是在最优的partition中,[math]\displaystyle{ \Omega_s }[/math]中的状态[math]\displaystyle{ i }[/math]的右奇异向量会和[math]\displaystyle{ v_s }[/math]距离最小,也就是说,让每个微观态的右特征向量和它对应的宏观态的右特征向量尽可能接近。
这个看起来非常眼熟,其实它就是在做kMeans聚类算法。让[math]\displaystyle{ v_s }[/math]和组里的[math]\displaystyle{ V[i:r] }[/math]距离最小的方法,就是让[math]\displaystyle{ v_s }[/math]成为[math]\displaystyle{ V[i:r] }[/math]这若干个点的中心。
回顾一下kMeans算法把n个点聚成r类的目标函数,其中第[math]\displaystyle{ i }[/math]个点被归到第[math]\displaystyle{ c^i }[/math]类,而[math]\displaystyle{ \mu_j }[/math]为第[math]\displaystyle{ j }[/math]类点的中心点:
[math]\displaystyle{ J(c^1, ... , c^n, \mu_1, ... , \mu_r) = \sum_{i=1}^n || x_i - \mu_{c^i} ||^2 }[/math]
我们看到这两个目标函数是非常相似的。所以,我们就可以通过上述算法或者kMeans来获取最优partition,然后根据这个partition来判断马尔科夫矩阵的lumpability。
- ↑ 1.0 1.1 1.2 Kemeny, John G., and J. Laurie Snell. Finite markov chains. Vol. 26. Princeton, NJ: van Nostrand, 1969. https://www.math.pku.edu.cn/teachers/yaoy/Fall2011/Kemeny-Snell_Chapter6.3-4.pdf
- ↑ 2.0 2.1 J. Xu, T. L. Wickramarathne, and N. V. Chawla, 'Representing higher-order dependencies in networks,' Science Advances, vol. 2, Article ID el600028, 2016.a
- ↑ 3.0 3.1 3.2 Buchholz, Peter. "Exact and ordinary lumpability in finite Markov chains." Journal of applied probability 31.1 (1994): 59-75.
- ↑ Franceschinis, Giuliana, and Richard R. Muntz. "Bounds for quasi-lumpable Markov chains." Performance Evaluation 20.1-3 (1994): 223-243.
- ↑ Piazza, Carla, and Sabina Rossi. "Reasoning about proportional lumpability." International Conference on Quantitative Evaluation of Systems. Cham: Springer International Publishing, 2021.
- ↑ 6.0 6.1 6.2 Zhang, Anru, and Mengdi Wang. "Spectral state compression of markov processes." IEEE transactions on information theory 66.5 (2019): 3202-3231.