蚁群优化算法
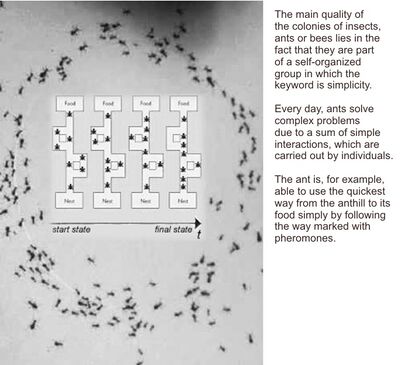
在计算机科学和运筹学中, 蚁群优化算法 ant colony optimization algorithm(ACO)是一种解决计算问题的概率化方法,它可以简化为通过图 Graph (discrete mathematics)来寻找最优路径。人工蚁群代表了受真实蚂蚁行为启发的多主体方法。
基于信息素的蚂蚁通信方法常被作为一种典型范式[2]。人工蚁群和局部搜索算法的组合已经是许多优化任务的一种求解方法,这些优化任务往往涉及某种,例如车辆路径 vehicle routing和互联网路由 internet routing的问题。这一领域的蓬勃发展催生了专门讨论人工蚂蚁的学术会议,以及诸如 AntOptima 等专业公司的大量商业应用。
以蚁群算法[3]为例,它是一种模拟蚁群行为的优化算法。人造”蚂蚁“(例如:仿真模拟的主体)通过在代表所有可能解的参数空间移动来求取最优解。真实蚂蚁们在探索环境时,会产生信息素 pheromone来指引彼此寻找资源。模拟的“蚂蚁”会类似地记录它们的位置和求解结果的好坏,以便在随后的仿真迭代计算中有更多的蚂蚁找到更好的解。[4]蚁群算法的一个变种是蜜蜂算法,它更类似于另一种社会性昆虫——蜜蜂的觅食模式。
在群智能 swarm intelligence方法中,这个算法是蚁群算法家族的一员。它构成了某些元启发式 metaheuristic的优化。第一个蚁群算法最初由 Marco Dorigo 在1992年的博士论文中提出[5][6],目的是基于蚂蚁在它们的聚居地和食物之间寻找一条路径的行为,在一张图中寻找最佳路径。借鉴了蚂蚁行为的各个方面,最初的蚁群算法已经变得多样化以解决更多类型的数值问题,并且也出现了一些问题。从更高的角度来看,蚁群算法执行基于模型的搜索[7] ,并与分布估计算法 estimation of distribution algorithm具有一定的相似性。
概览
在自然世界中,某些种类的蚂蚁(最初)随机徘徊,一旦发现食物便回到群落,同时留下信息素的轨迹。如果其他蚂蚁找到了这条路径,它们可能不会继续随机行走,而是沿着信息素轨迹前进。如果它们最终找到了食物,就会返回并加强这一信息素的路径[8]。
然而,随着时间的推移,信息素的轨迹开始蒸发,对蚂蚁的吸引力减小。蚂蚁沿着路径往返需要的时间越长,信息素蒸发所需的时间就越长。相比之下,蚂蚁更频繁地穿越短路径,因此短路径上的信息素密度比长路径上更高。在算法中,信息素蒸发还具有避免收敛到局部最优解的优点。如果根本没有蒸发,那么第一批蚂蚁选择的路径对后面的蚂蚁来说过度有吸引力。在这种情况下,算法对解空间的探索范围将受到限制。信息素蒸发对实际蚂蚁系统的影响尚不清楚,但在人工系统中非常关键[9]。
总的结果是,当一只蚂蚁找到一条从蚁群到食物来源的好的(即短的)路径时,其他蚂蚁更有可能沿着这条路径,正反馈最终导致许多蚂蚁沿着同一条路径。蚁群算法的思想是通过“仿真蚂蚁”在代表待解决问题的图上移动来模仿蚂蚁的行为。
智能物体的周围网络 Ambient networks of intelligent objects
因为“智能”不再是集中的而可以在所有微小的物体中找到,所以需要引入一些新的概念。以人为中心的概念被认为引导了 IT 系统的产生,其中数据处理、控制单元和算力是集中的。这些集中的单元功能不断地提高,并可以与人类大脑相比较。大脑模型已经成为计算机的终极愿景。由智能物体构成的环境网络,以及基于纳米技术 nanotechnology的新一代信息系统,迟早会深刻地改变这种概念。可以与昆虫相比的小型设备本身并不具备高智能。事实上,他们的智能程度相当有限。例如,不可能把一个能解决任何数学问题的高性能计算器集成到可以植入人体的生物芯片中,或者集成到一个用于跟踪商品的智能标签中。然而,一旦这些物体互相连接起来,它们就拥有了可以与一群蚂蚁或蜜蜂相提并论的智慧。在某些问题下,这种类型的智能可能优于类似于大脑的集中系统的推理[10]。
大自然中存在很多例子,说明了微小的生物在遵循同样的基本规则时,是如何创造出一种宏观层面的集体智慧 collective intelligence的。社会性昆虫的群落完美地说明了这个与人类社会有很大不同的模型。该模型基于具有简单和不可预测行为的独立单元之间的合作。[11] 他们穿过周围地区执行某些任务,但只掌握了非常有限的信息。例如,一群蚂蚁具有许多可以应用到环境对象的网络中的特性。蚂蚁群体具有很高的适应环境变化的能力,并且有很强的能力处理某项个体无法完成的任务。这种灵活性对于不断发展的移动网络也是非常有用的,从一台计算机到一个数字化对象的信息包和蚂蚁的行为一样。它们穿过网络并从一个节点传到下一个节点,目的是尽快到达终点。[12]
人工信息素系统 Artificial pheromone system
基于信息素的通信是自然界中普遍存在的、最有效的通信方式之一。信息素被社会性的昆虫所利用,如
蜜蜂、蚂蚁和白蚁; 既用于主体之间的通信也用于主体与群体之间的通信。由于其可行性,人工信息素已被应用于多机器人和群机器人系统中。基于信息素的通信是通过化学[13][14][15]或物理(RFID标签[16]、光[17][18][19][20]、声[21])方式实现的。然而,这些方式并不能复制信息素在自然中呈现的所有方面。
2007年,Garnier,Simon 等人在一篇IEEE论文[22] 中提出了使用投射光作为研究基于信息素的微型自主机器人通信的实验装置。另一项针对群机器人系统的研究提出了一种新颖的信息素通信方法 cosφ[23],该方法基于精确快速的视觉定位。[24]
该系统可以模拟几乎无限数量的不同信息素,并在机器人移动的水平 LCD 屏幕上以灰度图像的形式提供它们相互作用的结果。为了演示信息素通信方法,自主微型机器人Colias被作为群体机器人平台。
算法和公式 Algorithm and formulae
在蚁群算法中,人工蚂蚁是一种简单的计算主体,可以为给定优化问题寻找最优解。为了应用蚁群算法,需要将优化问题转化为在加权图上寻找最短路径的问题。在每次迭代的第一步,每个蚂蚁随机构造一个解,即图中的边应遵循的顺序。在第二步中,比较不同蚂蚁发现的路径。最后一步是更新每个边上的信息素水平。
procedure ACO_MetaHeuristic is while not_termination do generateSolutions() daemonActions() pheromoneUpdate() repeat end procedure
边的选择 Edge selection
每个蚂蚁都需要构造一个解来遍历图。为了在遍历过程中选择下一条边,蚂蚁将考虑从其当前位置可以获得的每条边的长度,以及相应的信息素水平。在算法的每一步,每一个蚂蚁都从状态[math]\displaystyle{ x }[/math]移动到状态[math]\displaystyle{ y }[/math],状态[math]\displaystyle{ y }[/math]对应一个更完整的中间解。因此,每个蚂蚁[math]\displaystyle{ k }[/math] 在每次迭代中计算一组可行展开式集合[math]\displaystyle{ A_k(x) }[/math],并以一定概率移动到其中一个。对于蚂蚁[math]\displaystyle{ k }[/math],从状态[math]\displaystyle{ x }[/math]移动到状态[math]\displaystyle{ y }[/math]的概率[math]\displaystyle{ p_{xy}^k }[/math]取决于两个值的组合,即移动的吸引力[math]\displaystyle{ \eta_{xy} }[/math],这是由某种启发式计算得出的,表示移动的先验期望值和本次移动的信息素踪迹浓度等级[math]\displaystyle{ \tau_{xy} }[/math],这表明它在过去进行该特定移动的熟练程度。踪迹浓度等级代表了本次移动期望的后验指标。
一般来说,蚂蚁[math]\displaystyle{ k }[/math]从状态[math]\displaystyle{ x }[/math]移动到状态[math]\displaystyle{ y }[/math]的概率
- [math]\displaystyle{ p_{xy}^k =\frac{ (\tau_{xy}^{\alpha}) (\eta_{xy}^{\beta}) } { \sum_{z\in \mathrm{allowed}_x} (\tau_{xz}^{\alpha}) (\eta_{xz}^{\beta}) } }[/math]
其中,[math]\displaystyle{ \tau_{xy} }[/math] 是从状态[math]\displaystyle{ x }[/math] 到[math]\displaystyle{ y }[/math] 转移的信息素积累量,0 ≤ [math]\displaystyle{ \alpha }[/math]是控制[math]\displaystyle{ \tau_{xy} }[/math] 影响的参数,[math]\displaystyle{ \eta_{xy} }[/math] 是状态转换[math]\displaystyle{ xy }[/math] 的期望值(一种先验信息,通常为[math]\displaystyle{ 1/d_{xy} }[/math],其中d是距离),[math]\displaystyle{ \beta }[/math]≥1是控制[math]\displaystyle{ \eta_{xy} }[/math]影响的参数。 [math]\displaystyle{ \tau_{xz} }[/math]和[math]\displaystyle{ \eta_{xz} }[/math]代表了其他可能的状态转移的轨迹浓度等级和吸引力
信息素更新 Pheromone update
当所有的蚂蚁都完成了求解过程,路径通常都被更新,通过分别增加或减少路径浓度水平对应的“好”或者“坏”的移动。全局信息素更新规则的一个例子是
- [math]\displaystyle{ \tau_{xy} \leftarrow (1-\rho)\tau_{xy} + \sum_{k}\Delta \tau^{k}_{xy} }[/math]
式中,[math]\displaystyle{ \tau_{xy} }[/math]是状态转换[math]\displaystyle{ xy }[/math]的信息素沉积量,[math]\displaystyle{ \rho }[/math]是信息素蒸发系数,[math]\displaystyle{ \Delta \tau^{k}_{xy} }[/math]是第[math]\displaystyle{ k }[/math]只蚂蚁沉积的信息素量,通常针对TSP问题(移动对应于图的弧)给出:
- [math]\displaystyle{ \Delta \tau^{k}_{xy} =\begin{cases} Q/L_k & \mbox{if ant }k\mbox{ uses curve }xy\mbox{ in its tour} \\ 0 & \mbox{otherwise} \end{cases} }[/math]
其[math]\displaystyle{ L_k }[/math]是蚂蚁[math]\displaystyle{ k }[/math]移动的代价(通常是长度),[math]\displaystyle{ Q }[/math]是一个常数。
常见延展Common extensions
下面是一些常见的 ACO 算法变体。
蚂蚁系统 Ant System (AS)
蚂蚁系统是第一种蚁群算法。此算法与前述算法相对应。它是由 Dorigo 开发的。[25]
蚁群系统 Ant Colony System (ACS)
在蚁群系统算法中,对原来的蚁群算法在三个方面进行了改进:
- (i)边的选择偏向于开发 exploitation(即偏向选择具有大量信息素的最短边的概率);
- (ii)在求解过程中,蚂蚁通过应用局部信息素更新规则来改变所选边的信息素水平;
- (iii)在每次迭代结束时,只允许最优的蚂蚁通过改进的全局信息素更新规则来更新路径。[26]
精英蚂蚁系统 Elitist Ant System
这种算法中,在每次迭代之后,全局最优解的蚂蚁与其他所有蚂蚁将信息素沉积在该路径上(即使这条路径没有被重访问过)。
最大-最小蚂蚁系统 Max-Min Ant System (MMAS)
该算法控制每条路线上信息素的最大和最小数量。只有全局最优或迭代最优才允许在其路线中添加信息素。为了避免搜索算法的停滞不前,每条路径上可能的信息素数量范围被限制在一个区间[τmax,τmin]。所有边都被初始化为τmax,以进行最多的求解。当算法接近停滞状态时,路线被重新初始化为τmax。[27]
基于排序的蚂蚁系统 Rank-based Ant System (ASrank)
所有解都是根据长度排序的。只有固定个数的最佳蚂蚁可以在迭代中更新他们的轨迹。信息素沉积量对每个解进行加权,使得路径较短的解比路径较长的解沉积更多的信息素。
连续正交蚁群 Continuous Orthogonal Ant Colony (COAC)
COAC的信息素沉积机制使蚂蚁能够协同有效地寻找解决方案。利用正交化设计法,可行域中的蚂蚁可以快速有效地搜索所选区域,提高了全局搜索能力和准确性。正交设计法和自适应半径调整法也可以推广到其他优化算法中,在解决实际问题时具有更广泛的优势。[28]
递归蚁群优化 Recursive Ant Colony Optimization
它是蚂蚁系统的一种递归 recursive形式,将整个搜索域划分为若干子域,并在这些子域上求解。[29]对所有子域的结果进行比较,并将其中最好的几个子域提升到下一个等级。与选定结果相对应的子区域被进一步细分,并重复这个过程,直到获得所需精度的输出。该方法在不适定地球物理反演问题中得到了验证,效果良好。[30]
收敛 Convergence
对于某些版本的算法,可以证明它是收敛的(也就是说,它能在有限时间内找到全局最优解)。蚁群算法的收敛性在2000年首次得到证实,然后是基于图的蚂蚁系统算法,以及后来的 ACS 和 MMAS 算法。和大多数启发式算法一样,很难估计理论上的收敛速度。对连续蚁群算法相关各参数(边的选择策略、距离测度方法和信息素蒸发率)的性能分析表明,蚁群算法的性能和收敛速度对参数值,特别是信息素蒸发率的参数选择非常敏感。[31] 在2004年,Zlochin 和他的同事[32]们展示了COAC类型的算法在交叉熵 cross-entropy和分布估计算法 estimation of distribution algorithm中可以同化为随机梯度下降方法。他们提出这些元启发式算法作为一个“基于研究的模型”。
应用
蚁群算法已经被应用于许多组合优化问题,从二次分配 quadratic assignment到蛋白质折叠 protein folding或路径选择 routing vehicles问题,以及许多派生方法已经被应用于实变量的动态问题、随机问题、多目标和并行方法。
它也被用来产生旅行商问题 travelling salesman problem的近似最优解。当图形可能发生动态变化时,它们比模拟退火算法 simulated annealing和遗传算法 genetic algorithm具有优势;蚁群算法可以连续运行并实时适应变化。这是网络路由和城市交通系统中很感兴趣的内容。
第一个蚁群算法被称为蚂蚁系统[25],它的目标是解决旅行商问题。旅行商问题的目标是找到最短的往返路线连接一系列城市。通常的算法相对简单,可以基于一组蚂蚁,每个蚂蚁沿着城市进行一次可能的往返旅行。在每个阶段,蚂蚁都会根据一些规则从一个城市迁移到另一个城市:
- 1.每个城市必须只到达一次;
- 2.较远的城市被选中的机会较少(可见度);
- 3.在两个城市之间的边上留下的信息素轨迹越强烈,这条边被选择的概率就越大;
- 4.旅程结束后,如果旅程很短,蚂蚁会在所经过的所有边上释放更多的信息素;
- 5.每次迭代后,信息素踪迹会蒸发。
规划问题
- 顺序排序问题 Sequential Ordering Problem (SOP) [33]
- 工序车间调度问题 Job-shop scheduling problem (JSP)[34]
- 开放车间调度问题 Open-shop scheduling problem (OSP)[35][36]
- 排列流车间问题 Permutation flow shop problem (PFSP)[37]
- 单机总延迟问题 Single machine total tardiness problem (SMTTP)[38]
- 单机总加权延迟调度问题 Single machine total weighted tardiness problem (SMTWTP)[39][40][41]
- 资源受限的项目调度问题 Resource-constrained project scheduling problem (RCPSP)[42]
- 组车间调度问题 Group-shop scheduling problem (GSP)[43]
- 具有序列依赖设置时间的单机总延迟问题 Single-machine total tardiness problem with sequence dependent setup times (SMTTPDST)[44]
- 具有序列依赖设置/转换时间的多级流车间调度问题 Multistage flowshop scheduling problem (MFSP) with sequence dependent setup/changeover times[45]
车辆路线问题 Vehicle routing problem
- 多基地车辆路径问题 Multi-depot vehicle routing problem (MDVRP)[49]
- 周期车辆路径问题 Period vehicle routing problem (PVRP)[50]
- 分批配送车辆路径问题 Split delivery vehicle routing problem (SDVRP)[51]
- 随机车辆路径问题Stochastic vehicle routing problem (SVRP)[52]
- 带时间窗的时间相关的车辆路径问题 Time dependent vehicle routing problem with time windows (TDVRPTW)[59]
- 带时间窗和多个服务工人的车辆路径问题 Vehicle routing problem with time windows and multiple service workers (VRPTWMS)
分配问题 Assignment problem
集合问题 Set problem
- 权约束的图树划分问题 Weight constrained graph tree partition problem (WCGTPP)[68]
- 弧加权的l-基数树问题 Arc-weighted l-cardinality tree problem (AWlCTP)[69]
- 多重背包问题 Multiple knapsack problem (MKP)[70]
- 最大独立集问题 Maximum independent set problem (MIS)[71]
纳米电子学物理设计中的器件尺寸问题 Device sizing problem in nanoelectronics physical design
- 基于蚁群优化(ACO)的45 nm CMOS感放电路优化可以在非常短的时间内收敛到最优解。[72]
- 基于蚁群优化(ACO)的可逆电路合成可以显著提高效率 [73]
天线优化与综合 Antennas optimization and synthesis


为了优化天线的结构,可以使用蚁群算法。例如,可以考虑基于蚁群算法(ACO)的天线RFID标签, [75]10×10的回送和卸载振动器[74]
图像处理 Image processing
在图像处理中,蚁群算法可以用于进行边缘检测与与边缘连接。[76][77]
- 边缘检测 Edge detection:
这里的图是二维图像,并且蚂蚁从一个像素的沉积信息素遍历。蚂蚁从一个像素移动到另一个是由图像灰度值的局部变化引导的。这样的移动导致最高密度的信息素沉积在边缘上。
以下是使用 ACO 进行边缘检测的步骤:[78][79][80]
Step1: 初始化:
在图像[math]\displaystyle{ I_{M_1 M_2} }[/math]上随机放置蚂蚁[math]\displaystyle{ K }[/math],其中[math]\displaystyle{ K= (M_1*M_2)^\tfrac{1}{2} }[/math] 。信息素矩阵[math]\displaystyle{ tau_{(i,j)} }[/math]由一个随机值初始化。在初始化过程中的主要困难是确定启发式矩阵。
确定启发式矩阵的方法有多种。对于下面的例子,启发式矩阵是根据局部统计数据计算出来的:
像素(i,j)的局部统计数据。
- [math]\displaystyle{ \eta_{(i,j)}= \tfrac{1}{Z}*Vc*I_{(i,j)} }[/math]
其中 [math]\displaystyle{ I }[/math] 是尺寸为 [math]\displaystyle{ M_1*M_2 }[/math]的图像
- [math]\displaystyle{ Z =\sum_{i=1:M_1} \sum_{j=1:M_2} Vc(I_{i,j}) }[/math], 是一个归一化因子
- [math]\displaystyle{ \begin{align}Vc(I_{i,j}) = &f \left( \left\vert I_{(i-2,j-1)} - I_{(i+2,j+1)} \right\vert + \left\vert I_{(i-2,j+1)} - I_{(i+2,j-1)} \right\vert \right. \\ & +\left\vert I_{(i-1,j-2)} - I_{(i+1,j+2)} \right\vert + \left\vert I_{(i-1,j-1)} - I_{(i+1,j+1)} \right\vert\\ & +\left\vert I_{(i-1,j)} - I_{(i+1,j)} \right\vert + \left\vert I_{(i-1,j+1)} - I_{(i-1,j-1)} \right\vert\\ & + \left. \left\vert I_{(i-1,j+2)} - I_{(i-1,j-2)} \right\vert + \left\vert I_{(i,j-1)} - I_{(i,j+1)} \right\vert \right) \end{align} }[/math]
[math]\displaystyle{ f(\cdot) }[/math]可以用以下函数计算:
- [math]\displaystyle{ f(x) = \lambda x, \quad \text{for x ≥ 0; (1)} }[/math]
- [math]\displaystyle{ f(x) = \lambda x^2, \quad \text{for x ≥ 0; (2)} }[/math]
- [math]\displaystyle{ f(x) =\begin{cases}
\sin(\frac{\pi x}{2 \lambda}), & \text{for 0 ≤ x ≤} \lambda \text{; (3)} \\
0, & \text{else}
\end{cases} }[/math]
- [math]\displaystyle{ f(x) =\begin{cases}\pi x \sin(\frac{\pi x}{2 \lambda}), & \text{for 0 ≤ x ≤} \lambda \text{; (4)} \\ 0, & \text{else}\end{cases} }[/math]
上面每个函数中的参数[math]\displaystyle{ \lambda }[/math]用来调整函数各自的形状。
Step 2 构建过程:
蚂蚁的移动是在4个连接的像素或8个连接的像素进行的。根据概率方程[math]\displaystyle{ P_{x,y} }[/math]给出蚂蚁移动的概率
Step 3与Step 5更新过程
信息素矩阵更新两次。在步骤3中,蚂蚁(由[math]\displaystyle{ \tau_{(x,y)} }[/math]给出)的踪迹被更新,就像在Step 5中,蚂蚁的踪迹蒸发率由下面的方程进行更新。
- [math]\displaystyle{ \tau_{new} \leftarrow (1-\psi)\tau_{old} + \psi \tau_{0} }[/math], where [math]\displaystyle{ \psi }[/math] is the pheromone decay coefficient [math]\displaystyle{ 0\lt \tau \lt 1 }[/math]
- [math]\displaystyle{ \tau_{new} \leftarrow (1-\psi)\tau_{old} + \psi \tau_{0} }[/math], 其中 [math]\displaystyle{ \psi }[/math] 是信息素衰减系数 [math]\displaystyle{ 0\lt \tau \lt 1 }[/math]
Step 7 决策过程:
一旦 k 只蚂蚁在 n 次迭代中移动了一个固定的距离 l,可以基于信息素矩阵 τ 上的阈值 T判断它是否是一个边缘。下面例子的阈值是根据 Otsu 的方法计算的。
使用 ACO 检测图像边缘: < br/> 下面的图像是使用方程(1)至(4)给出的不同函数生成的。[81]
- 边缘连接 Edge linking:[82] ACO算法在边缘连接算法中也是证明有效的。
其他应用 Other applications
- 面向连接的网络路由 Connection-oriented network routing[85]
- 项目调度中的折现现金流 Discounted cash flows in project scheduling[91]
- 能源与电力网络设计 [94]
- 网格工作流调度问题 Grid workflow scheduling problem[95]
- 蛋白质-蛋白质相互作用的抑制肽设计 Inhibitory peptide design for protein protein interactions[96]
- 智能测试系统 Intelligent testing system[97]
- 电源电子电路设计 Power electronic circuit design[98]
[99]
发明者是 Frans Moyson 和 Bernard Manderick。这一领域的先驱包括 Marco Dorigo, Luca Maria Gambardell。
定义困难 Definition difficulty
采用蚁群优化算法,图中两点 a 和 b 之间的最短路径是由多条路径组合建立的。[105]要准确定义算法是不是蚁群算法并不容易,因为其定义可能因作者和用途而有所不同。广义地说,蚁群算法被认为是一种填充的元启发式算法,每个解由一个在搜索空间中移动的蚂蚁表示。[106]蚂蚁标记最优解,并考虑到以前的标记来优化搜索。它们可以被看作是概率化多智能体算法,使用概率分布进行每次迭代之间的转换。[107] 在用于解决组合问题的蚁群算法版本中,使用了一种解的迭代构造方法。[108] 根据一些作者的观点,蚁群算法区别于其他相关算法(比如估计分布的算法或粒子群优化算法)的是蚁群算法的建设性方面。在组合问题中,即使没有蚂蚁被证明是有效的,最终可能会找到最好的解。因此,在旅行商问题的例子中,蚂蚁实际上并不需要走最短的路线: 最短的路线可以从最优解中最强的部分建立起来。然而,在实变量中没有“相邻”这样的结构存在,所以这一定义在实变量问题的情况下可能是有问题的。群居昆虫的集体行为仍然是研究人员的灵感来源。各种算法(无论是优化还是非优化)寻找生物系统中的自组织促进了“群体智能”的概念。
Stigmergy算法 Stigmergy algorithms
在实践中,有大量的算法声称是“蚁群”,但并不总是拥有典型蚁群优化的一般框架。[109]
在实践中,蚂蚁之间通过环境交换信息(称为“stigmergy”的原理)被认为足以使算法属于蚁群算法。基于这种“合作性”的分类原则,作者提出了一些“合作式”的分类和运输方式。[110]
实际上,通过蚂蚁之间通过环境交换信息的行为(被称为“stigmergy”原理)足以使一种算法属于蚁群算法的一类。这一原则促使一些作者创造“价值”这个词来组织基于寻找食物,幼虫分类,分工和合作运输的方法和行为。[111]
相关方法 Related methods
- 基因算法Genetic algorithm (GA) 维护不是一个而是一组解。寻找更好的解的过程模仿了进化的过程,解被组合或变异以改变解空间,较差的解被丢弃。
- 分布估计算法 estimation of distribution algorithm(EDA)是一种进化算法,它用模型引导的算子代替传统的复制算子。这类模型通过机器学习技术从群体中学习,并以概率图形模型表示,从中可以通过抽样[112][113]或引导交叉[114][115]生成新的解。
- 模拟退火 Simulated annealing (SA) 是一种相关的全局优化技术,它通过生成当前解的相邻解来遍历搜索空间。更好的的相邻解总是被接受的。根据质量和温度参数的差异概率地接受较差的相邻解。随着算法的进行,温度参数会被修改,以改变搜索的性质。
- 反应式搜索优化是将机器学习与优化相结合,通过添加一个内部反馈回路,根据问题、实例和当前解周围的局部情况,对算法的自由参数进行自调整。
- 塔布搜索 Tabu search (TS) 类似于模拟退火,两者都通过测试单个解的突变来遍历解空间。模拟退火只产生一个变异解,塔布搜索则产生许多变异解,并转移到适应度最低的解。为了防止循环并鼓励在解空间中进行更大的移动,塔布列表保留了部分或完整的解。禁止移动到包含塔布列表元素的解,该列表会随着解遍历解空间而更新
- 人工免疫系统 Artificial immune system (AIS) 算法是以脊椎动物免疫系统为模型的。
- 粒子群优化 Particle swarm optimization (PSO), 一种群智能方法。
- Intelligent water drops (IWD),一种基于自然水滴在河流中流动的群优化算法
- 随机扩散搜索 Stochastic diffusion search (SDS),一种基于主体的概率全局搜索和优化技术,最适合于目标函数可分解为多个独立的部分函数的问题
历史
发明者是Frans Moyson和Bernard Manderick。该领域的先驱包括 Marco Dorigo, Luca Maria Gambardella.[116]
蚁群优化算法年表
- 1959年,Pierre Paul grasse发明了stigmergy理论来解释白蚁筑巢的行为
- 1983年,Deneubourg和他的同事研究了蚂蚁的集体行为 [117]
- 1988年,Moyson Manderick发表了一篇关于蚂蚁“自组织”的文章 [118]
- 1989年,Goss,Aron,Deneubourg和pastels关于“阿根廷蚂蚁的集体行为”的研究,这将为蚁群优化算法提供思路。[119]
- 1989年,Ebling 和他的同事们实施了一种食物行为模式。[120]
- 1991年,M.Dorigo在他的博士论文(发表于1992年[6])中提出了“蚂蚁系统”[121]五年后,由V. Maniezzo和A. Colorni共同撰写的从论文中摘录的一份技术报告发表了。 [25]
- 1994年,英国电信公司的Appleby和Steward发布了第一个应用于电信网络的应用程序 [122]
- 1995, Gambardella和Dorigo提议ant-q, [123] 蚁群系统作为蚁群系统第一延伸的初步版本 [25].
- 1996年,Gambardella和Dorigo提议蚁群系统 ant colony system [124]
- 1996, 发表关于蚂蚁系统的文章[25]
- 1996, Hoos和Stützle发明了 max-min ant system;[27]
- 1997, Dorigo和Gambardella提出了结合局部搜索的蚁群系统 [26]
- 1997, Schoonderwoerd 和他的同事发表了一个改进的应用程序到电信网络 [125]
- 1998,召开了第一次专门讨论ACO算法的会议[126]
- 1998, Stützle 提出最初的并行实现 parallel implementations;[127]
- 1999, Gambardella, Taillard 和 Agazzi proposed macs-vrptw, 这是第一个应用于带时间窗的车辆路径问题的多蚁群系统 [128]
- 1999, Bonabeau, Dorigo 和 Theraulaz 出版了一本主要讨论人工蚂蚁的书[129]
- 2000,《未来一代计算机系统杂志》关于蚂蚁算法的特刊 [130]
- 2000, 首次应用于调度,调度序列和约束满足 。
- 2000,Gutjahr为蚁群算法提供了收敛性的第一个证据 [131]
- 2001, 公司首次使用COA算法(Eurobios and AntOptima);
- 2001, Iredi 和他的同事发表了第一个“多目标”算法[132]
- 2002, 贝叶斯网络在进度计划设计中的首次应用
- 2002, Bianchi和她的同事提出了随机问题的第一个算法;[133]
- 2004, Dorigo和Stützle与麻省理工学院出版社出版了《蚁群优化》一书 [134]
- 2004, Zlochin和Dorigo证明了一些算法等价于随机梯度下降 stochastic gradient descent、交叉熵方法 cross-entropy method和估计分布的算法 algorithms to estimate distribution[32]
- 2005, 首次应用于蛋白质折叠问题
- 2012, Prabhakar和他的同事们发表了一项研究,内容涉及单个蚂蚁在没有信息素的情况下进行串联通信,这反映了计算机网络组织的原理。将通信模型与传输控制协议 Transmission Control Protocol进行了比较。[135]
- 2016, 首次应用于肽序列设计。[96]
- 2017, 多准则决策方法PROMETHEE成功集成到ACO算法中(HUMANT algorithm).[136]
参考 References
- ↑ Waldner, Jean-Baptiste (2008). Nanocomputers and Swarm Intelligence. London: ISTE Ltd John Wiley & Sons. p. 225. ISBN 978-1-84704-002-2.
- ↑ Monmarché Nicolas, Guinand Frédéric and Siarry Patrick (2010). Artificial Ants. Wiley-ISTE. ISBN 978-1-84821-194-0.
- ↑ Dorigo, Gambardella, M, L.M. (1997). "Learning Approach to the Traveling Salesman Problem". IEEE Transactions on Evolutionary Computation, 1 (1): 214.
{{cite journal}}
: Cite journal requires|journal=
(help) - ↑ Ant Colony Optimization by Marco Dorigo and Thomas Stützle, MIT Press, 2004.
- ↑ A. Colorni, M. Dorigo et V. Maniezzo, Distributed Optimization by Ant Colonies, actes de la première conférence européenne sur la vie artificielle, Paris, France, Elsevier Publishing, 134-142, 1991.
- ↑ 6.0 6.1 M. Dorigo, Optimization, Learning and Natural Algorithms, PhD thesis, Politecnico di Milano, Italy, 1992.
- ↑ Zlochin, Mark; Birattari, Mauro; Meuleau, Nicolas; Dorigo, Marco (1 October 2004). "Model-Based Search for Combinatorial Optimization: A Critical Survey". Annals of Operations Research (in English). 131 (1–4): 373–395. CiteSeerX 10.1.1.3.427. doi:10.1023/B:ANOR.0000039526.52305.af. ISSN 0254-5330. S2CID 63137.
- ↑ Fladerer, Johannes-Paul; Kurzmann, Ernst (November 2019). WISDOM OF THE MANY : how to create self -organisation and how to use collective... intelligence in companies and in society from mana.. BOOKS ON DEMAND. ISBN 9783750422421.
- ↑ Marco Dorigo and Thomas Stültze, Ant Colony Optimization, p.12. 2004.
- ↑ Waldner, Jean-Baptiste (2008). Nanocomputers and Swarm Intelligence. London: ISTE John Wiley & Sons. p. 214. ISBN 978-1-84704-002-2.
- ↑ Waldner, Jean-Baptiste (2007). Inventer l'Ordinateur du XXIème Siècle. London: Hermes Science. pp. 259–265. ISBN 978-2-7462-1516-0.
- ↑ Waldner, Jean-Baptiste (2008). Nanocomputers and Swarm Intelligence. London: ISTE John Wiley & Sons. p. 215. ISBN 978-1-84704-002-2.
- ↑ Lima, Danielli A., and Gina MB Oliveira. "A cellular automata ant memory model of foraging in a swarm of robots." Applied Mathematical Modelling 47, 2017: 551-572.
- ↑ Russell, R. Andrew. "Ant trails-an example for robots to follow?." Robotics and Automation, 1999. Proceedings. 1999 IEEE International Conference on. Vol. 4. IEEE, 1999.
- ↑ Fujisawa, Ryusuke, et al. "Designing pheromone communication in swarm robotics: Group foraging behavior mediated by chemical substance." Swarm Intelligence 8.3 (2014): 227-246.
- ↑ Sakakibara, Toshiki, and Daisuke Kurabayashi. "Artificial pheromone system using rfid for navigation of autonomous robots." Journal of Bionic Engineering 4.4 (2007): 245-253.
- ↑ Arvin, Farshad, et al. "Investigation of cue-based aggregation in static and dynamic environments with a mobile robot swarm." Adaptive Behavior (2016): 1-17.
- ↑ Farshad Arvin, et al. "Imitation of honeybee aggregation with collective behavior of swarm robots." International Journal of Computational Intelligence Systems 4.4 (2011): 739-748.
- ↑ Schmickl, Thomas, et al. "Get in touch: cooperative decision making based on robot-to-robot collisions." Autonomous Agents and Multi-Agent Systems 18.1 (2009): 133-155.
- ↑ Garnier, Simon, et al. "Do ants need to estimate the geometrical properties of trail bifurcations to find an efficient route? A swarm robotics test bed." PLoS Comput Biol 9.3 (2013): e1002903.
- ↑ Arvin, Farshad, et al. "Cue-based aggregation with a mobile robot swarm: a novel fuzzy-based method." Adaptive Behavior 22.3 (2014): 189-206.
- ↑ Garnier, Simon, et al. "Alice in pheromone land: An experimental setup for the study of ant-like robots." 2007 IEEE Swarm Intelligence Symposium. IEEE, 2007.
- ↑ Farshad Arvin et al. "COSΦ: artificial pheromone system for robotic swarms research." IEEE/RSJ International Conference on Intelligent Robots and Systems (IROS) 2015.
- ↑ Krajník, Tomáš, et al. "A practical multirobot localization system." Journal of Intelligent & Robotic Systems 76.3-4 (2014): 539-562.
- ↑ 25.0 25.1 25.2 25.3 25.4 M. Dorigo, V. Maniezzo, et A. Colorni, Ant system: optimization by a colony of cooperating agents, IEEE Transactions on Systems, Man, and Cybernetics--Part B , volume 26, numéro 1, pages 29-41, 1996.
- ↑ 26.0 26.1 M. Dorigo et L.M. Gambardella, Ant Colony System : A Cooperative Learning Approach to the Traveling Salesman Problem, IEEE Transactions on Evolutionary Computation, volume 1, numéro 1, pages 53-66, 1997.
- ↑ 27.0 27.1 T. Stützle et H.H. Hoos, MAX MIN Ant System, Future Generation Computer Systems, volume 16, pages 889-914, 2000
- ↑ X Hu, J Zhang, and Y Li (2008). Orthogonal methods based ant colony search for solving continuous optimization problems. Journal of Computer Science and Technology, 23(1), pp.2-18.
- ↑ Gupta, D.K.; Arora, Y.; Singh, U.K.; Gupta, J.P., "Recursive Ant Colony Optimization for estimation of parameters of a function," Recent Advances in Information Technology (RAIT), 2012 1st International Conference on , vol., no., pp.448-454, 15–17 March 2012
- ↑ Gupta, D.K.; Gupta, J.P.; Arora, Y.; Shankar, U., "Recursive ant colony optimization: a new technique for the estimation of function parameters from geophysical field data," Near Surface Geophysics , vol. 11, no. 3, pp.325-339
- ↑ V.K.Ojha, A. Abraham and V. Snasel, ACO for Continuous Function Optimization: A Performance Analysis, 14th International Conference on Intelligent Systems Design and Applications (ISDA), Japan, Page 145 - 150, 2017, 978-1-4799-7938-7/14 2014 IEEE.
- ↑ 32.0 32.1 M. Zlochin, M. Birattari, N. Meuleau, et M. Dorigo, Model-based search for combinatorial optimization: A critical survey, Annals of Operations Research, vol. 131, pp. 373-395, 2004.
- ↑ L.M. Gambardella, M. Dorigo, "An Ant Colony System Hybridized with a New Local Search for the Sequential Ordering Problem", INFORMS Journal on Computing, vol.12(3), pp. 237-255, 2000.
- ↑ D. Martens, M. De Backer, R. Haesen, J. Vanthienen, M. Snoeck, B. Baesens, Classification with Ant Colony Optimization, IEEE Transactions on Evolutionary Computation, volume 11, number 5, pages 651—665, 2007.
- ↑ B. Pfahring, "Multi-agent search for open scheduling: adapting the Ant-Q formalism," Technical report TR-96-09, 1996.
- ↑ C. Blem, "Beam-ACO, Hybridizing ant colony optimization with beam search. An application to open shop scheduling," Technical report TR/IRIDIA/2003-17, 2003.
- ↑ T. Stützle, "An ant approach to the flow shop problem," Technical report AIDA-97-07, 1997.
- ↑ A. Bauer, B. Bullnheimer, R. F. Hartl and C. Strauss, "Minimizing total tardiness on a single machine using ant colony optimization," Central European Journal for Operations Research and Economics, vol.8, no.2, pp.125-141, 2000.
- ↑ M. den Besten, "Ants for the single machine total weighted tardiness problem," Master's thesis, University of Amsterdam, 2000.
- ↑ M, den Bseten, T. Stützle and M. Dorigo, "Ant colony optimization for the total weighted tardiness problem," Proceedings of PPSN-VI, Sixth International Conference on Parallel Problem Solving from Nature, vol. 1917 of Lecture Notes in Computer Science, pp.611-620, 2000.
- ↑ D. Merkle and M. Middendorf, "An ant algorithm with a new pheromone evaluation rule for total tardiness problems," Real World Applications of Evolutionary Computing, vol. 1803 of Lecture Notes in Computer Science, pp.287-296, 2000.
- ↑ D. Merkle, M. Middendorf and H. Schmeck, "Ant colony optimization for resource-constrained project scheduling," Proceedings of the Genetic and Evolutionary Computation Conference (GECCO 2000), pp.893-900, 2000.
- ↑ C. Blum, "ACO applied to group shop scheduling: a case study on intensification and diversificationhttps://en.wikipedia.org/wiki/Defekte_Weblinks?dwl={{{url}}} Seite nicht mehr abrufbar], Suche in Webarchiven: Kategorie:Wikipedia:Weblink offline (andere Namensräume)[http://timetravel.mementoweb.org/list/2010/Kategorie:Wikipedia:Vorlagenfehler/Vorlage:Toter Link/URL_fehlt ," Proceedings of ANTS 2002, vol. 2463 of Lecture Notes in Computer Science, pp.14-27, 2002.
- ↑ C. Gagné, W. L. Price and M. Gravel, "Comparing an ACO algorithm with other heuristics for the single machine scheduling problem with sequence-dependent setup times," Journal of the Operational Research Society, vol.53, pp.895-906, 2002.
- ↑ A. V. Donati, V. Darley, B. Ramachandran, "An Ant-Bidding Algorithm for Multistage Flowshop Scheduling Problem: Optimization and Phase Transitions", book chapter in Advances in Metaheuristics for Hard Optimization, Springer, pp.111-138, 2008.
- ↑ Toth, Paolo; Vigo, Daniele (2002). "Models, relaxations and exact approaches for the capacitated vehicle routing problem". Discrete Applied Mathematics. 123 (1–3): 487–512. doi:10.1016/S0166-218X(01)00351-1.
- ↑ J. M. Belenguer, and E. Benavent, "A cutting plane algorithm for capacitated arc routing problem," Computers & Operations Research, vol.30, no.5, pp.705-728, 2003.
- ↑ T. K. Ralphs, "Parallel branch and cut for capacitated vehicle routing," Parallel Computing, vol.29, pp.607-629, 2003.
- ↑ Salhi, S.; Sari, M. (1997). "A multi-level composite heuristic for the multi-depot vehicle fleet mix problem". European Journal of Operational Research. 103: 95–112. doi:10.1016/S0377-2217(96)00253-6.
- ↑ Angelelli, Enrico; Speranza, Maria Grazia (2002). "The periodic vehicle routing problem with intermediate facilities". European Journal of Operational Research. 137 (2): 233–247. doi:10.1016/S0377-2217(01)00206-5.
{{cite journal}}
: Cite has empty unknown parameter:|1=
(help) - ↑ Ho, Sin C.; Haugland, Dag (2002). "A Tabu Search Heuristic for the Vehicle Routing Problem with Time Windows and Split Deliveries". Computers and Operations Research. 31 (12): 1947–1964. CiteSeerX 10.1.1.8.7096. doi:10.1016/S0305-0548(03)00155-2.
- ↑ Secomandi, Nicola. "Comparing neuro-dynamic programming algorithms for the vehicle routing problem with stochastic demands". Computers & Operations Research: 2000. CiteSeerX 10.1.1.392.4034.
- ↑ W. P. Nanry and J. W. Barnes, "Solving the pickup and delivery problem with time windows using reactive tabu search," Transportation Research Part B, vol.34, no. 2, pp.107-121, 2000.
- ↑ R. Bent and P.V. Hentenryck, "A two-stage hybrid algorithm for pickup and delivery vehicle routing problems with time windows," Computers & Operations Research, vol.33, no.4, pp.875-893, 2003.
- ↑ L.M. Gambardella, E. Taillard, G. Agazzi, "MACS-VRPTW: A Multiple Ant Colony System for Vehicle Routing Problems with Time Windows", In D. Corne, M. Dorigo and F. Glover, editors, New Ideas in Optimization, McGraw-Hill, London, UK, pp. 63-76, 1999.
- ↑ Bachem, A.; Hochstättler, W.; Malich, M. (1996). "The simulated trading heuristic for solving vehicle routing problems". Discrete Applied Mathematics. 65 (1–3): 47–72. doi:10.1016/0166-218X(95)00027-O.
- ↑ Hong, Sung-Chul; Park, Yang-Byung (1999). "A heuristic for bi-objective vehicle routing with time window constraints". International Journal of Production Economics. 62 (3): 249–258. doi:10.1016/S0925-5273(98)00250-3.
- ↑ Russell, Robert A.; Chiang, Wen-Chyuan (2006). "Scatter search for the vehicle routing problem with time windows". European Journal of Operational Research. 169 (2): 606–622. doi:10.1016/j.ejor.2004.08.018.
- ↑ A. V. Donati, R. Montemanni, N. Casagrande, A. E. Rizzoli, L. M. Gambardella, "Time Dependent Vehicle Routing Problem with a Multi Ant Colony System", European Journal of Operational Research, vol.185, no.3, pp.1174–1191, 2008.
- ↑ "MAX-MIN Ant System for Quadratic Assignment Problems". 1997. CiteSeerX 10.1.1.47.5167.
{{cite journal}}
: Cite journal requires|journal=
(help) - ↑ R. Lourenço and D. Serra "Adaptive search heuristics for the generalized assignment problem," Mathware & soft computing, vol.9, no.2-3, 2002.
- ↑ M. Yagiura, T. Ibaraki and F. Glover, "An ejection chain approach for the generalized assignment problem," INFORMS Journal on Computing, vol. 16, no. 2, pp. 133–151, 2004.
- ↑ K. I. Aardal, S. P. M. van Hoesel, A. M. C. A. Koster, C. Mannino and Antonio. Sassano, "Models and solution techniques for the frequency assignment problem," A Quarterly Journal of Operations Research, vol.1, no.4, pp.261-317, 2001.
- ↑ Y. C. Liang and A. E. Smith, "An ant colony optimization algorithm for the redundancy allocation problem (RAP)," IEEE Transactions on Reliability, vol.53, no.3, pp.417-423, 2004.
- ↑ G. Leguizamon and Z. Michalewicz, "A new version of ant system for subset problems," Proceedings of the 1999 Congress on Evolutionary Computation(CEC 99), vol.2, pp.1458-1464, 1999.
- ↑ R. Hadji, M. Rahoual, E. Talbi and V. Bachelet "Ant colonies for the set covering problem," Abstract proceedings of ANTS2000, pp.63-66, 2000.
- ↑ V Maniezzo and M Milandri, "An ant-based framework for very strongly constrained problems," Proceedings of ANTS2000, pp.222-227, 2002.
- ↑ R. Cordone and F. Maffioli,"Colored Ant System and local search to design local telecommunication networkshttps://en.wikipedia.org/wiki/Defekte_Weblinks?dwl={{{url}}} Seite nicht mehr abrufbar], Suche in Webarchiven: Kategorie:Wikipedia:Weblink offline (andere Namensräume)[http://timetravel.mementoweb.org/list/2010/Kategorie:Wikipedia:Vorlagenfehler/Vorlage:Toter Link/URL_fehlt ," Applications of Evolutionary Computing: Proceedings of Evo Workshops, vol.2037, pp.60-69, 2001.
- ↑ C. Blum and M.J. Blesa, "Metaheuristics for the edge-weighted k-cardinality tree problem," Technical Report TR/IRIDIA/2003-02, IRIDIA, 2003.
- ↑ S. Fidanova, "ACO algorithm for MKP using various heuristic information", Numerical Methods and Applications, vol.2542, pp.438-444, 2003.
- ↑ G. Leguizamon, Z. Michalewicz and Martin Schutz, "An ant system for the maximum independent set problem," Proceedings of the 2001 Argentinian Congress on Computer Science, vol.2, pp.1027-1040, 2001.
- ↑ O. Okobiah, S. P. Mohanty, and E. Kougianos, "Ordinary Kriging Metamodel-Assisted Ant Colony Algorithm for Fast Analog Design Optimization -{zh-cn:互联网档案馆; zh-tw:網際網路檔案館; zh-hk:互聯網檔案館;}-的存檔,存档日期March 4, 2016,.", in Proceedings of the 13th IEEE International Symposium on Quality Electronic Design (ISQED), pp. 458--463, 2012.
- ↑ M. Sarkar, P. Ghosal, and S. P. Mohanty, "Reversible Circuit Synthesis Using ACO and SA based Quinne-McCluskey Method -{zh-cn:互联网档案馆; zh-tw:網際網路檔案館; zh-hk:互聯網檔案館;}-的存檔,存档日期July 29, 2014,.", in Proceedings of the 56th IEEE International Midwest Symposium on Circuits & Systems (MWSCAS), 2013, pp. 416--419.
- ↑ 74.0 74.1 74.2 Ermolaev S.Y., Slyusar V.I. Antenna synthesis based on the ant colony optimization algorithm.// Proc. ICATT’2009, Lviv, Ukraine 6 - 9 Octobre, 2009. - Pages 298 - 300 [1]
- ↑ Marcus Randall, Andrew Lewis, Amir Galehdar, David Thiel. Using Ant Colony Optimisation to Improve the Efficiency of Small Meander Line RFID Antennas.// In 3rd IEEE International e-Science and Grid Computing Conference [2], 2007
- ↑ S. Meshoul and M Batouche, "Ant colony system with extremal dynamics for point matching and pose estimation," Proceedings of the 16th International Conference on Pattern Recognition, vol.3, pp.823-826, 2002.
- ↑ H. Nezamabadi-pour, S. Saryazdi, and E. Rashedi, "Edge detection using ant algorithms", Soft Computing, vol. 10, no.7, pp. 623-628, 2006.
- ↑ Tian, Jing; Yu, Weiyu; Xie, Shengli (2008). 2008 IEEE Congress on Evolutionary Computation (IEEE World Congress on Computational Intelligence). pp. 751–756. doi:10.1109/CEC.2008.4630880. ISBN 978-1-4244-1822-0.
- ↑ Gupta, Charu; Gupta, Sunanda. "Edge Detection of an Image based on Ant ColonyOptimization Technique".
- ↑ Jevtić, A.; Quintanilla-Dominguez, J.; Cortina-Januchs, M.G.; Andina, D. (2009). Edge detection using ant colony search algorithm and multiscale contrast enhancement. pp. 2193–2198. doi:10.1109/ICSMC.2009.5345922. ISBN 978-1-4244-2793-2.
- ↑ "File Exchange [[:模板:Ndash]] Ant Colony Optimization (ACO)". MATLAB Central.
{{cite web}}
: URL–wikilink conflict (help) - ↑ Jevtić, A.; Melgar, I.; Andina, D. (2009). 2009 35th Annual Conference of IEEE Industrial Electronics. 35th Annual Conference of IEEE Industrial Electronics, 2009. IECON '09.. pp. 3353–3358. doi:10.1109/IECON.2009.5415195. ISBN 978-1-4244-4648-3.
- ↑ Zhang, Y. (2013). "A Rule-Based Model for Bankruptcy Prediction Based on an Improved Genetic Ant Colony Algorithm". Mathematical Problems in Engineering. 2013: 753251. doi:10.1155/2013/753251.
- ↑ 84.0 84.1 D. Martens, M. De Backer, R. Haesen, J. Vanthienen, M. Snoeck, B. Baesens, "Classification with Ant Colony Optimization", IEEE Transactions on Evolutionary Computation, volume 11, number 5, pages 651—665, 2007.
- ↑ G. D. Caro and M. Dorigo, "Extending AntNet for best-effort quality-of-service routing," Proceedings of the First International Workshop on Ant Colony Optimization (ANTS’98), 1998.
- ↑ G.D. Caro and M. Dorigo "AntNet: a mobile agents approach to adaptive routing," Proceedings of the Thirty-First Hawaii International Conference on System Science, vol.7, pp.74-83, 1998.
- ↑ G. D. Caro and M. Dorigo, "Two ant colony algorithms for best-effort routing in datagram networks," Proceedings of the Tenth IASTED International Conference on Parallel and Distributed Computing and Systems (PDCS’98), pp.541-546, 1998.
- ↑ D. Martens, B. Baesens, T. Fawcett "Editorial Survey: Swarm Intelligence for Data Mining," Machine Learning, volume 82, number 1, pp. 1-42, 2011
- ↑ R. S. Parpinelli, H. S. Lopes and A. A Freitas, "An ant colony algorithm for classification rule discovery," Data Mining: A heuristic Approach, pp.191-209, 2002.
- ↑ R. S. Parpinelli, H. S. Lopes and A. A Freitas, "Data mining with an ant colony optimization algorithm," IEEE Transactions on Evolutionary Computation, vol.6, no.4, pp.321-332, 2002.
- ↑ W. N. Chen, J. ZHANG and H. Chung, "Optimizing Discounted Cash Flows in Project Scheduling--An Ant Colony Optimization Approach", IEEE Transactions on Systems, Man, and Cybernetics--Part C: Applications and Reviews Vol.40 No.5 pp.64-77, Jan. 2010.
- ↑ D. Picard, A. Revel, M. Cord, "An Application of Swarm Intelligence to Distributed Image Retrieval", Information Sciences, 2010
- ↑ D. Picard, M. Cord, A. Revel, "Image Retrieval over Networks : Active Learning using Ant Algorithm", IEEE Transactions on Multimedia, vol. 10, no. 7, pp. 1356--1365 - nov 2008
- ↑ Warner, Lars; Vogel, Ute (2008). Optimization of energy supply networks using ant colony optimization (PDF). Environmental Informatics and Industrial Ecology — 22nd International Conference on Informatics for Environmental Protection. Aachen, Germany: Shaker Verlag. ISBN 978-3-8322-7313-2. Retrieved 2018-10-09.
- ↑ W. N. Chen and J. ZHANG "Ant Colony Optimization Approach to Grid Workflow Scheduling Problem with Various QoS Requirements", IEEE Transactions on Systems, Man, and Cybernetics--Part C: Applications and Reviews, Vol. 31, No. 1,pp.29-43,Jan 2009.
- ↑ 96.0 96.1 Zaidman, Daniel; Wolfson, Haim J. (2016-08-01). "PinaColada: peptide–inhibitor ant colony ad-hoc design algorithm". Bioinformatics. 32 (15): 2289–2296. doi:10.1093/bioinformatics/btw133. ISSN 1367-4803. PMID 27153578.
- ↑ Xiao. M.Hu, J. ZHANG, and H. Chung, "An Intelligent Testing System Embedded with an Ant Colony Optimization Based Test Composition Method", IEEE Transactions on Systems, Man, and Cybernetics--Part C: Applications and Reviews, Vol. 39, No. 6, pp. 659-669, Dec 2009.
- ↑ J. ZHANG, H. Chung, W. L. Lo, and T. Huang, "Extended Ant Colony Optimization Algorithm for Power Electronic Circuit Design", IEEE Transactions on Power Electronic. Vol.24,No.1, pp.147-162, Jan 2009.
- ↑ J. ZHANG, H. Chung, W. L. Lo, and T. Huang, "Extended Ant Colony Optimization Algorithm for Power Electronic Circuit Design", IEEE Transactions on Power Electronic. Vol.24,No.1, pp.147-162, Jan 2009.
- ↑ X. M. Hu, J. ZHANG,J. Xiao and Y. Li, "Protein Folding in Hydrophobic-Polar Lattice Model: A Flexible Ant- Colony Optimization Approach ", Protein and Peptide Letters, Volume 15, Number 5, 2008, Pp. 469-477.
- ↑ A. Shmygelska, R. A. Hernández and H. H. Hoos, "An ant colony optimization algorithm for the 2D HP protein folding problem," Proceedings of the 3rd International Workshop on Ant Algorithms/ANTS 2002, Lecture Notes in Computer Science, vol.2463, pp.40-52, 2002.
- ↑ M. Nardelli; L. Tedesco; A. Bechini (2013). Cross-lattice behavior of general ACO folding for proteins in the HP model. pp. 1320–1327. doi:10.1145/2480362.2480611. ISBN 9781450316569. https://zenodo.org/record/3445092.
- ↑ L. Wang and Q. D. Wu, "Linear system parameters identification based on ant system algorithm," Proceedings of the IEEE Conference on Control Applications, pp. 401-406, 2001.
- ↑ K. C. Abbaspour, R. Schulin, M. T. Van Genuchten, "Estimating unsaturated soil hydraulic parameters using ant colony optimization," Advances In Water Resources, vol. 24, no. 8, pp. 827-841, 2001.
- ↑ Shmygelska, Alena; Hoos, Holger H. (2005). "An ant colony optimisation algorithm for the 2D and 3D hydrophobic polar protein folding problem". BMC Bioinformatics. 6: 30. doi:10.1186/1471-2105-6-30. PMC 555464. PMID 15710037.
- ↑ Fred W. Glover,Gary A. Kochenberger, Handbook of Metaheuristics, [3], Springer (2003)
- ↑ http://www.multiagent.fr/extensions/ICAPManager/pdf/LauriCharpillet2006.pdf
- ↑ WJ Gutjahr , ACO algorithms with guaranteed convergence to the optimal solution, [4], (2002)
- ↑ Santpal Singh Dhillon , Ant Routing, Searching and Topology Estimation Algorithms for Ad Hoc Networks, [5], IOS Press, (2008)
- ↑ A. Ajith; G. Crina; R. Vitorino (éditeurs), Stigmergic Optimization, Studies in Computational Intelligence , volume 31, 299 pages, 2006.
- ↑ A. Ajith; G. Crina; R. Vitorino (éditeurs), Stigmergic Optimization, Studies in Computational Intelligence , volume 31, 299 pages, 2006.
- ↑ Pelikan, Martin; Goldberg, David E.; Cantú-Paz, Erick (1 January 1999). BOA: The Bayesian Optimization Algorithm. Gecco'99. pp. 525–532. ISBN 9781558606111. http://dl.acm.org/citation.cfm?id=2933973.
- ↑ Pelikan, Martin (2005). Hierarchical Bayesian optimization algorithm : toward a new generation of evolutionary algorithms (1st ed.). Berlin [u.a.]: Springer. ISBN 978-3-540-23774-7.
- ↑ Thierens, Dirk (11 September 2010). "The Linkage Tree Genetic Algorithm" (in en). pp. 264–273. doi:10.1007/978-3-642-15844-5_27. ISBN 978-3-642-15843-8.
- ↑ Martins, Jean P.; Fonseca, Carlos M.; Delbem, Alexandre C. B. (25 December 2014). "On the performance of linkage-tree genetic algorithms for the multidimensional knapsack problem". Neurocomputing. 146: 17–29. doi:10.1016/j.neucom.2014.04.069.
- ↑ Manderick, Moyson, Bernard, Frans (1988). "The collective behavior of ants: An example of self-organization in massive parallelism". Stanford: Proceedings of the AAAI Spring Symposium on Parallel Models of Intelligence.
{{cite journal}}
: Cite journal requires|journal=
(help) - ↑ J.L. Denebourg, J.M. Pasteels et J.C. Verhaeghe, Probabilistic Behaviour in Ants : a Strategy of Errors?, Journal of Theoretical Biology, numéro 105, 1983.
- ↑ F. Moyson, B. Manderick, The collective behaviour of Ants : an Example of Self-Organization in Massive Parallelism, Actes de AAAI Spring Symposium on Parallel Models of Intelligence, Stanford, Californie, 1988.
- ↑ S. Goss, S. Aron, J.-L. Deneubourg et J.-M. Pasteels, Self-organized shortcuts in the Argentine ant, Naturwissenschaften, volume 76, pages 579-581, 1989
- ↑ M. Ebling, M. Di Loreto, M. Presley, F. Wieland, et D. Jefferson,An Ant Foraging Model Implemented on the Time Warp Operating System, Proceedings of the SCS Multiconference on Distributed Simulation, 1989
- ↑ Dorigo M., V. Maniezzo et A. Colorni, Positive feedback as a search strategy, rapport technique numéro 91-016, Dip. Elettronica, Politecnico di Milano, Italy, 1991
- ↑ Appleby, S. & Steward, S. Mobile software agents for control in telecommunications networks, BT Technol. J., 12(2):104–113, April 1994
- ↑ L.M. Gambardella and M. Dorigo, "Ant-Q: a reinforcement learning approach to the traveling salesman problem", Proceedings of ML-95, Twelfth International Conference on Machine Learning, A. Prieditis and S. Russell (Eds.), Morgan Kaufmann, pp. 252–260, 1995
- ↑ L.M. Gambardella and M. Dorigo, "Solving Symmetric and Asymmetric TSPs by Ant Colonies", Proceedings of the IEEE Conference on Evolutionary Computation, ICEC96, Nagoya, Japan, May 20-22, pp. 622-627, 1996;
- ↑ R. Schoonderwoerd, O. Holland, J. Bruten et L. Rothkrantz, Ant-based load balancing in telecommunication networks, Adaptive Behaviour, volume 5, numéro 2, pages 169-207, 1997
- ↑ M. Dorigo, ANTS’ 98, From Ant Colonies to Artificial Ants : First International Workshop on Ant Colony Optimization, ANTS 98, Bruxelles, Belgique, octobre 1998.
- ↑ T. Stützle, Parallelization Strategies for Ant Colony Optimization, Proceedings of PPSN-V, Fifth International Conference on Parallel Problem Solving from Nature, Springer-Verlag, volume 1498, pages 722-731, 1998.
- ↑ L.M. Gambardella, E. Taillard, G. Agazzi, "MACS-VRPTW: A Multiple Ant Colony System for Vehicle Routing Problems with Time Windows", In D. Corne, M. Dorigo and F. Glover, editors, New Ideas in Optimization, McGraw-Hill, London, UK, pp. 63-76, 1999.
- ↑ É. Bonabeau, M. Dorigo et G. Theraulaz, Swarm intelligence, Oxford University Press, 1999.
- ↑ M. Dorigo , G. Di Caro et T. Stützle, Special issue on "Ant Algorithms", Future Generation Computer Systems, volume 16, numéro 8, 2000
- ↑ W.J. Gutjahr, A graph-based Ant System and its convergence, Future Generation Computer Systems, volume 16, pages 873-888, 2000.
- ↑ S. Iredi, D. Merkle et M. Middendorf, Bi-Criterion Optimization with Multi Colony Ant Algorithms, Evolutionary Multi-Criterion Optimization, First International Conference (EMO’01), Zurich, Springer Verlag, pages 359-372, 2001.
- ↑ L. Bianchi, L.M. Gambardella et M.Dorigo, An ant colony optimization approach to the probabilistic traveling salesman problem, PPSN-VII, Seventh International Conference on Parallel Problem Solving from Nature, Lecture Notes in Computer Science, Springer Verlag, Berlin, Allemagne, 2002.
- ↑ M. Dorigo and T. Stützle, Ant Colony Optimization, MIT Press, 2004.
- ↑ B. Prabhakar, K. N. Dektar, D. M. Gordon, "The regulation of ant colony foraging activity without spatial information ", PLOS Computational Biology, 2012. URL: http://www.ploscompbiol.org/article/info%3Adoi%2F10.1371%2Fjournal.pcbi.1002670
- ↑ Mladineo, Marko; Veza, Ivica; Gjeldum, Nikola (2017). "Solving partner selection problem in cyber-physical production networks using the HUMANT algorithm". International Journal of Production Research. 55 (9): 2506–2521. doi:10.1080/00207543.2016.1234084. S2CID 114390939.
出版物 Publications (selected)
- M. Dorigo, 1992. Optimization, Learning and Natural Algorithms, PhD thesis, Politecnico di Milano, Italy.
- M. Dorigo, V. Maniezzo & A. Colorni, 1996. "Ant System: Optimization by a Colony of Cooperating Agents", IEEE Transactions on Systems, Man, and Cybernetics–Part B, 26 (1): 29–41.
本中文词条由Henry翻译,Lincent审校,薄荷欢迎在讨论页面留言。
本词条内容源自公开资料,遵守 CC3.0协议。