计算社会科学
此词条暂由彩云小译翻译,未经人工整理和审校,带来阅读不便,请见谅。
本词条的框架如下,大家可以选取感兴趣的部分,在对应的位置,写上自己的名字
- 计算社会科学定义————
- 计算社会科学发展历史————
- 计算社会科学的研究方法————
- 计算社会科学的应用与分支————
翻译整理截止日期:2020.5.8 18:00
Computational social science refers to the academic sub-disciplines concerned with computational approaches to the social sciences. This means that computers are used to model, simulate, and analyze social phenomena. Fields include computational economics, computational sociology, cliodynamics, culturomics, and the automated analysis of contents, in social and traditional media. It focuses on investigating social and behavioral relationships and interactions through social simulation, modeling, network analysis, and media analysis.[1]
Computational social science refers to the academic sub-disciplines concerned with computational approaches to the social sciences. This means that computers are used to model, simulate, and analyze social phenomena. Fields include computational economics, computational sociology, cliodynamics, culturomics, and the automated analysis of contents, in social and traditional media. It focuses on investigating social and behavioral relationships and interactions through social simulation, modeling, network analysis, and media analysis.
计算社会科学是指与社会科学计算方法有关的学术分支学科。这意味着计算机被用来建模、模拟和分析社会现象。领域包括计算经济学、计算社会学、动态学、文化学以及社交和传统媒体的内容自动分析。它着重于通过社会模拟、建模、网络分析和媒体分析来调查社会和行为关系和互动。
Definitions
定义
定义
There are two terminologies that relate to each other: Social Science Computing (SSC) and Computational Social Science (CSS). In literature, CSS is referred to the field of social science that uses the computational approaches in studying the social phenomena.
There are two terminologies that relate to each other: Social Science Computing (SSC) and Computational Social Science (CSS). In literature, CSS is referred to the field of social science that uses the computational approaches in studying the social phenomena.
有两个相互关联的术语: 社会科学计算(SSC)和计算社会科学(CSS)。在文献中,CSS 指的是社会科学领域,它使用计算方法来研究社会现象。
On the other hand, SSC is the field in which computational methodologies are created to assist in explanations of social phenomena.
On the other hand, SSC is the field in which computational methodologies are created to assist in explanations of social phenomena.
另一方面,南南合作是一个领域,其中计算方法创建,以协助解释社会现象。
Computational social science revolutionizes both fundamental legs of the scientific method: empirical research, especially through big data, by analyzing the digital footprint left behind through social online activities; and scientific theory, especially through computer simulation model building through social simulation.[2][3] It is a multi-disciplinary and integrated approach to social survey focusing on information processing by means of advanced information technology. The computational tasks include the analysis of social networks, social geographic systems,[4] social media content and traditional media content.
Computational social science revolutionizes both fundamental legs of the scientific method: empirical research, especially through big data, by analyzing the digital footprint left behind through social online activities; and scientific theory, especially through computer simulation model building through social simulation. It is a multi-disciplinary and integrated approach to social survey focusing on information processing by means of advanced information technology. The computational tasks include the analysis of social networks, social geographic systems, social media content and traditional media content.
计算社会科学彻底改变了科学方法的两个基本支柱: 实证研究,特别是通过大数据,通过分析社会在线活动留下的数据痕迹; 科学理论,特别是通过社会模拟建立计算机模拟模型。利用先进的信息技术进行以信息处理为核心的社会调查是一种多学科、综合的方法。计算任务包括分析社交网络、社交地理系统、社交媒体内容和传统媒体内容。
Computational social science work increasingly relies on the greater availability of large databases, currently constructed and maintained by a number of interdisciplinary projects, including:
Computational social science work increasingly relies on the greater availability of large databases, currently constructed and maintained by a number of interdisciplinary projects, including:
计算社会科学工作越来越依赖于大型数据库的更大可用性,这些数据库目前由一些跨学科项目建立和维护,包括:
- The Seshat: Global History Databank, which systematically collects state-of-the-art accounts of the political and social organization of human groups and how societies have evolved through time into an authoritative databank.[5] Seshat is affiliated also with the Evolution Institute, a non-profit think-tank that "uses evolutionary science to solve real-world problems."
- D-PLACE: the Database of Places, Languages, Culture and Environment, which provides data on over 1,400 human social formations[6]
- The Atlas of Cultural Evolution, an archaeological database created by Peter N. Peregrine[7]
- CHIA: The Collaborative Information for Historical Analysis, a multidisciplinary collaborative endeavor hosted by the University of Pittsburgh with the goal of archiving historical information and linking data as well as academic/research institutions around the globe
- International Institute of Social History, which collects data on the global social history of labour relations, workers, and labour
- Human Relations Area Files eHRAF Archaeology[8]
- Human Relations Area Files eHRAF World Cultures[9]
- Clio-Infra a database of measures of economic performance and other aspects of societal well-being on a global sample of societies from 1800 CE to the present
- The Google Ngram Viewer, an online search engine that charts frequencies of sets of comma-delimited search strings using a yearly count of n-grams as found in the largest online body of human knowledge, the Google Books corpus.
The analysis of vast quantities of historical newspaper[10] and book content[11] have been pioneered in 2017, while other studies on similar data[12] showed how periodic structures can be automatically discovered in historical newspapers. A similar analysis was performed on social media, again revealing strongly periodic structures.[13]
The analysis of vast quantities of historical newspaper and book content have been pioneered in 2017, while other studies on similar data showed how periodic structures can be automatically discovered in historical newspapers. A similar analysis was performed on social media, again revealing strongly periodic structures.
对大量历史报纸和书籍内容的分析在2017年开创了先河,而对类似数据的其他研究表明,周期结构可以在历史报纸中自动发现。在社交媒体上也进行了类似的分析,再次揭示了强烈的周期性结构。
历史
历史
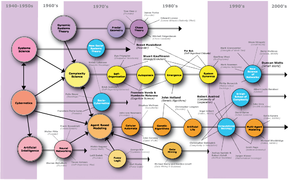
Background
In the past four decades, computational sociology has been introduced and gaining popularity 模板:According to whom. This has been used primarily for modeling or building explanations of social processes and are depending on the emergence of complex behavior from simple activities.[14] The idea behind emergence is that properties of any bigger system do not always have to be properties of the components that the system is made of.[15] The people responsible for the introduction of the idea of emergence are Alexander, Morgan, and Broad, who were classical emergentists. The time at which these emergentists came up with this concept and method was during the time of the early twentieth century. The aim of this method was to find a good enough accommodation between two different and extreme ontologies, which were reductionist materialism and dualism.[14]
While emergence has had a valuable and important role with the foundation of Computational Sociology, there are those who do not necessarily agree. One major leader in the field, Epstein, doubted the use because there were aspects that are unexplainable. Epstein put up a claim against emergentism, in which he says it "is precisely the generative sufficiency of the parts that constitutes the whole's explanation".[14]
Agent-based models have had a historical influence on Computational Sociology. These models first came around in the 1960s, and were used to simulate control and feedback processes in organizations, cities, etc. During the 1970s, the application introduced the use of individuals as the main units for the analyses and used bottom-up strategies for modeling behaviors. The last wave occurred in the 1980s. At this time, the models were still bottom-up; the only difference is that the agents interact interdependently.[14]
Systems theory and structural functionalism
In the post-war era, Vannevar Bush's differential analyser, John von Neumann's cellular automata, Norbert Wiener's cybernetics, and Claude Shannon's information theory became influential paradigms for modeling and understanding complexity in technical systems. In response, scientists in disciplines such as physics, biology, electronics, and economics began to articulate a general theory of systems in which all natural and physical phenomena are manifestations of interrelated elements in a system that has common patterns and properties. Following Émile Durkheim's call to analyze complex modern society sui generis,[16] post-war structural functionalist sociologists such as Talcott Parsons seized upon these theories of systematic and hierarchical interaction among constituent components to attempt to generate grand unified sociological theories, such as the AGIL paradigm.[17] Sociologists such as George Homans argued that sociological theories should be formalized into hierarchical structures of propositions and precise terminology from which other propositions and hypotheses could be derived and operationalized into empirical studies.[18] Because computer algorithms and programs had been used as early as 1956 to test and validate mathematical theorems, such as the four color theorem,[19] some scholars anticipated that similar computational approaches could "solve" and "prove" analogously formalized problems and theorems of social structures and dynamics.
系统论和功能主义
在战后时期,万尼瓦尔·布希的微分分析器、约翰·冯·诺伊曼的细胞自动机、诺伯特·维纳的模控学 与克劳德·夏农的信息论在技术系统中成为模拟与暸解复杂度具有影响力的典范。相对应地,在像是物理学、生物学、电子学,和经济学等学门的科学家开始表述一种一般性的系统理论,其中所有自然与物理现象皆为一个系统中具有相同模式与性质的相关元素的展现。 随着艾弥尔·涂尔干以实事求是的方式分析复杂现代社会的呼声[20] ,战后结构功能主义社会学家如塔尔科特·帕森斯利用这些构成元素之间系统化与阶层化互动的理论,来尝试生成宏大而统一的社会学理论,例如四种功能(AGIL paradigm)[17]。 如George Homans等社会学家辩称社会理论应该被形式化(正规化)(formalized),成为命题和精确术语的阶层结构,其他的命题与假设可以从中被推演出来并操作化以进行实证研究。[18] 由于电脑算法与程式早在1956年就已用来测试和验证数学定理,[21]例如四色定理,社会科学家与系统动力学家预期类似的计算取径可以类比地“解决”与“证明”正规化的问题,和社会结构与动力的理论。
Macrosimulation and microsimulation
By the late 1960s and early 1970s, social scientists used increasingly available computing technology to perform macro-simulations of control and feedback processes in organizations, industries, cities, and global populations. These models used differential equations to predict population distributions as holistic functions of other systematic factors such as inventory control, urban traffic, migration, and disease transmission.[22][23] Although simulations of social systems received substantial attention in the mid-1970s after the Club of Rome published reports predicting that policies promoting exponential economic growth would eventually bring global environmental catastrophe,[24] the inconvenient conclusions led many authors to seek to discredit the models, attempting to make the researchers themselves appear unscientific.[25][26] Hoping to avoid the same fate, many social scientists turned their attention toward micro-simulation models to make forecasts and study policy effects by modeling aggregate changes in state of individual-level entities rather than the changes in distribution at the population level.[27] However, these micro-simulation models did not permit individuals to interact or adapt and were not intended for basic theoretical research.[28]
宏观模拟与微观模拟
到了1960年代晚期与1970年代早期,社会科学家使用更为可得的电脑科技对组织、产业、城市,与全球人口进行控制与回馈过程的宏观模拟。这些模型使用微分方程,将人口分布视为其他系统性因素(如存货控管、都市交通、迁徙、疾病传染等)的整体计算型函数(holistic functions)来进行预测。罗马俱乐部根据对于全球经济的模拟而出版了预测全球环境浩劫的报告。[29][30] 尽管在这份报告发表后的1970年代中期,对社会体系的模拟因而得到了大量的关注,[31] 然而模型的结果被认为对于模型的假设非常敏感(在罗马俱乐部的例子中,仅有少数的证据支持),亦暂时使得这初生的领域失去可信度。[25][32] 对于利用计算工具来预测宏观的社会与经济行为产生的怀疑渐增,因此社会科学家将其注意力转向了微观模拟模型(microsimulation),借由模拟个人层级个体的状态渐进改变,而非人口层级的分布的改变,社会学家们作出了预测,也研究政策的效果[33]。 然而,这些微观模拟模型并未允许个体进行互动或适应,其目的也非基本理论研究[28]。
Cellular automata and agent-based modeling
The 1970s and 1980s were also a time when physicists and mathematicians were attempting to model and analyze how simple component units, such as atoms, give rise to global properties, such as complex material properties at low temperatures, in magnetic materials, and within turbulent flows.[34] Using cellular automata, scientists were able to specify systems consisting of a grid of cells in which each cell only occupied some finite states and changes between states were solely governed by the states of immediate neighbors. Along with advances in artificial intelligence and microcomputer power, these methods contributed to the development of "chaos theory" and "complexity theory" which, in turn, renewed interest in understanding complex physical and social systems across disciplinary boundaries.[25] Research organizations explicitly dedicated to the interdisciplinary study of complexity were also founded in this era: the Santa Fe Institute was established in 1984 by scientists based at Los Alamos National Laboratory and the BACH group at the University of Michigan likewise started in the mid-1980s.
This cellular automata paradigm gave rise to a third wave of social simulation emphasizing agent-based modeling. Like micro-simulations, these models emphasized bottom-up designs but adopted four key assumptions that diverged from microsimulation: autonomy, interdependency, simple rules, and adaptive behavior.[28] Agent-based models are less concerned with predictive accuracy and instead emphasize theoretical development.[35] In 1981, mathematician and political scientist Robert Axelrod and evolutionary biologist W.D. Hamilton published a major paper in Science titled "The Evolution of Cooperation" which used an agent-based modeling approach to demonstrate how social cooperation based upon reciprocity can be established and stabilized in a prisoner's dilemma game when agents followed simple rules of self-interest.[36] Axelrod and Hamilton demonstrated that individual agents following a simple rule set of (1) cooperate on the first turn and (2) thereafter replicate the partner's previous action were able to develop "norms" of cooperation and sanctioning in the absence of canonical sociological constructs such as demographics, values, religion, and culture as preconditions or mediators of cooperation.[37] Throughout the 1990s, scholars like William Sims Bainbridge, Kathleen Carley, Michael Macy, and John Skvoretz developed multi-agent-based models of generalized reciprocity, prejudice, social influence, and organizational information processing. In 1999, Nigel Gilbert published the first textbook on Social Simulation: Simulation for the social scientist and established its most relevant journal: the Journal of Artificial Societies and Social Simulation.
元胞自动机与基于主体的建模
20世纪七十到八十年代,数学家和物理学家尝试建模和分析怎样从简单的单元,比如原子中,产生全局现象,比如复杂材料才低温、磁场、和湍流中的属性。[38] 使用元胞自动机(Celluer Automata),设定了一个只由方格组成的系统,每个方格就是一个“元胞(cell)”。每个元胞只能有有限个状态,期额元胞在各个状态间的转换条件只由紧贴着该元胞的周围元胞状态决定。元胞自动机与人工智能技术和微型计算机的所获得的进步一道为混沌理论和复杂系统等研究领域的建立做出重大贡献,同时也冲洗唤起了人们在理解交叉学科的复杂物理和社会系统的兴趣。众多致力于研究复杂科学的科研组织也是建立于这个时候:圣塔菲研究所由一群来自洛斯阿拉莫斯国家实验室的物理学家在1984年发起,密歇根大学的BACH小组也是在八十年代中期成立的。
This cellular automata paradigm gave rise to a third wave of social simulation emphasizing agent-based modeling. Like micro-simulations, these models emphasized bottom-up designs but adopted four key assumptions that diverged from microsimulation: autonomy, interdependency, simple rules, and adaptive behavior.[28] Agent-based models are less concerned with predictive accuracy and instead emphasize theoretical development.[39] In 1981, mathematician and political scientist Robert Axelrod and evolutionary biologist W.D. Hamilton published a major paper in Science titled "The Evolution of Cooperation" which used an agent-based modeling approach to demonstrate how social cooperation based upon reciprocity can be established and stabilized in a prisoner's dilemma game when agents followed simple rules of self-interest.[40] Axelrod and Hamilton demonstrated that individual agents following a simple rule set of (1) cooperate on the first turn and (2) thereafter replicate the partner's previous action were able to develop "norms" of cooperation and sanctioning in the absence of canonical sociological constructs such as demographics, values, religion, and culture as preconditions or mediators of cooperation.[37] Throughout the 1990s, scholars like William Sims Bainbridge, Kathleen Carley, Michael Macy, and John Skvoretz developed multi-agent-based models of generalized reciprocity, prejudice, social influence, and organizational information processing. In 1999, Nigel Gilbert published the first textbook on Social Simulation: Simulation for the social scientist and established its most relevant journal: the Journal of Artificial Societies and Social Simulation.
这一轮元胞自动机的研究范式催生了使用基于主体建模(Agent-based Modeling)的第三次社会模拟浪潮。和宏观模拟类似,这些模型强调了自底向上的设计思想,但采用了四个不同于宏观建模的假设:自主(autonomy)、独立(interdependency)、简单规则(simple rules)、和适应性行为(adaptive behavior)。[28] 相比于预测的准确度,基于主体的建模更加强调理论的建立。[41] 在1981年,数学家与政治学家罗伯特·阿克塞尔罗德与演化生物学家威廉·汉密尔顿一同在《Science》杂志上发表了一篇名为《合作的进化(The Evolution of Cooperation)》的经典论文,其中使用了基于主体的建模来展示了在囚徒困境的博弈中,当主体们(agents)只遵循简单的、自利的规则时,也可以在互惠的原则上建立稳定的社会合作。[42] 阿克塞尔罗德和汉密尔顿展示了每个主体只要遵循(1)第一轮时选择合作(2)下一轮重复上一轮对方的做法这两条简单规则,就可以在没有社会权威的情况下建立起合作与惩罚的规范。[37] 九十年代学者们如William Sims Bainbridge, Kathleen Carley, Michael Macy,和John Skvoretz建立起了广义互惠、偏见、社会影响和组织信息处理等主题的基于主体的模型。在1999年,Nigel Gilbert发表了第一本关于社会模拟的教科书《Simulation for the social scientist》,并创立了与其相关的期刊《Journal of Artificial Societies and Social Simulation》。
Data mining and social network analysis
Independent from developments in computational models of social systems, social network analysis emerged in the 1970s and 1980s from advances in graph theory, statistics, and studies of social structure as a distinct analytical method and was articulated and employed by sociologists like James S. Coleman, Harrison White, Linton Freeman, J. Clyde Mitchell, Mark Granovetter, Ronald Burt, and Barry Wellman.[43] The increasing pervasiveness of computing and telecommunication technologies throughout the 1980s and 1990s demanded analytical techniques, such as network analysis and multilevel modeling, that could scale to increasingly complex and large data sets. The most recent wave of computational sociology, rather than employing simulations, uses network analysis and advanced statistical techniques to analyze large-scale computer databases of electronic proxies for behavioral data. Electronic records such as email and instant message records, hyperlinks on the World Wide Web, mobile phone usage, and discussion on Usenet allow social scientists to directly observe and analyze social behavior at multiple points in time and multiple levels of analysis without the constraints of traditional empirical methods such as interviews, participant observation, or survey instruments.[44] Continued improvements in machine learning algorithms likewise have permitted social scientists and entrepreneurs to use novel techniques to identify latent and meaningful patterns of social interaction and evolution in large electronic datasets.[45][46]
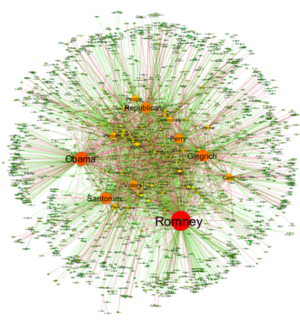
The automatic parsing of textual corpora has enabled the extraction of actors and their relational networks on a vast scale, turning textual data into network data. The resulting networks, which can contain thousands of nodes, are then analysed by using tools from Network theory to identify the key actors, the key communities or parties, and general properties such as robustness or structural stability of the overall network, or centrality of certain nodes.[48] This automates the approach introduced by quantitative narrative analysis,[49] whereby subject-verb-object triplets are identified with pairs of actors linked by an action, or pairs formed by actor-object.[47]
Computational content analysis
Content analysis has been a traditional part of social sciences and media studies for a long time. The automation of content analysis has allowed a "big data" revolution to take place in that field, with studies in social media and newspaper content that include millions of news items. Gender bias, readability, content similarity, reader preferences, and even mood have been analyzed based on text mining methods over millions of documents.[50][51][52][53][54] The analysis of readability, gender bias and topic bias was demonstrated in Flaounas et al.[55] showing how different topics have different gender biases and levels of readability; the possibility to detect mood shifts in a vast population by analysing Twitter content was demonstrated as well.[56]
The analysis of vast quantities of historical newspaper content has been pioneered by Dzogang et al.,[57] which showed how periodic structures can be automatically discovered in historical newspapers. A similar analysis was performed on social media, again revealing strongly periodic structures.[58]
研究方法
Methodologies
Methodologically, social complexity is theory-neutral, meaning that it accommodates both local and global approaches to sociological research.[59] The very idea of social complexity arises out of the historical-comparative methods of early sociologists; obviously, this method is important in developing, defining, and refining the theoretical construct of social complexity. As complex social systems have many parts and there are many possible relationships between those parts, appropriate methodologies are typically determined to some degree by the research level of analysis differentiated[60] by the researcher according to the level of description or explanation demanded by the research hypotheses.
At the most localized level of analysis, ethnographic, participant- or non-participant observation, content analysis and other qualitative research methods may be appropriate. More recently, highly sophisticated quantitative research methodologies are being developed and used in sociology at both local and global levels of analysis. Such methods include (but are not limited to) bifurcation diagrams, network analysis, non-linear modeling, and computational models including cellular automata programming, sociocybernetics and other methods of social simulation.
Complex social network analysis
Complex social network analysis is used to study the dynamics of large, complex social networks. Dynamic network analysis brings together traditional social network analysis, link analysis and multi-agent systems within network science and network theory.[61] Through the use of key concepts and methods in social network analysis, agent-based modeling, theoretical physics, and modern mathematics (particularly graph theory and fractal geometry), this method of inquiry brought insights into the dynamics and structure of social systems. New computational methods of localized social network analysis are coming out of the work of Duncan Watts, Albert-László Barabási, Nicholas A. Christakis, Kathleen Carley and others.
New methods of global network analysis are emerging from the work of John Urry and the sociological study of globalization, linked to the work of Manuel Castells and the later work of Immanuel Wallerstein. Since the late 1990s, Wallerstein increasingly makes use of complexity theory, particularly the work of Ilya Prigogine.[62][63][64] Dynamic social network analysis is linked to a variety of methodological traditions, above and beyond systems thinking, including graph theory, traditional social network analysis in sociology, and mathematical sociology. It also links to mathematical chaos and complex dynamics through the work of Duncan Watts and Steven Strogatz, as well as fractal geometry through Albert-László Barabási and his work on scale-free networks.
Computational sociology
The development of computational sociology involves such scholars as Nigel Gilbert, Klaus G. Troitzsch, Joshua M. Epstein, and others. The foci of methods in this field include social simulation and data-mining, both of which are sub-areas of computational sociology. Social simulation uses computers to create an artificial laboratory for the study of complex social systems; data-mining uses machine intelligence to search for non-trivial patterns of relations in large, complex, real-world databases. The emerging methods of socionics are a variant of computational sociology.[65][66]
Computational sociology is influenced by a number of micro-sociological areas as well as the macro-level traditions of systems science and systems thinking. The micro-level influences of symbolic interaction, exchange, and rational choice, along with the micro-level focus of computational political scientists, such as Robert Axelrod, helped to develop computational sociology's bottom-up, agent-based approach to modeling complex systems. This is what Joshua M. Epstein calls generative science.[66] Other important areas of influence include statistics, mathematical modeling and computer simulation.
Sociocybernetics
Sociocybernetics integrates sociology with second-order cybernetics and the work of Niklas Luhmann, along with the latest advances in complexity science. In terms of scholarly work, the focus of sociocybernetics has been primarily conceptual and only slightly methodological or empirical.[67] Sociocybernetics is directly tied to systems thought inside and outside of sociology, specifically in the area of second-order cybernetics.
应用与分支
Areas of application
As a middle-range theoretical platform, social complexity can be applied to any research in which social interaction or the outcomes of such interactions can be observed, but particularly where they can be measured and expressed as continuous or discrete data points. One common criticism often cited regarding the usefulness of complexity science in sociology is the difficulty of obtaining adequate data.[68] Nonetheless, application of the concept of social complexity and the analysis of such complexity has begun and continues to be an ongoing field of inquiry in sociology. From childhood friendships and teen pregnancy[59] to criminology[69] and counter-terrorism,[70] theories of social complexity are being applied in almost all areas of sociological research.
In the area of communications research and informetrics, the concept of self-organizing systems appears in mid-1990s research related to scientific communications.[71] Scientometrics and bibliometrics are areas of research in which discrete data are available, as are several other areas of social communications research such as sociolinguistics.[59] Social complexity is also a concept used in semiotics.[72]
In the first decade of the 21st century, the diversity of areas of application has grown[73] as more sophisticated methods have developed. Social complexity theory is applied in studies of social cooperation and public goods;[74] altruism;[75] voting behavior;[76][77] education;[78] global civil society [79] and global civil unrest;[80] collective action and social movements;[81][82] social inequality;[83] workforce and unemployment;[84][85] economic geography and economic sociology;[86] policy analysis;[87][88] health care systems;[89] and innovation and social change,[90][91] to name a few. A current international scientific research project, the Seshat: Global History Databank, was explicitly designed to analyze changes in social complexity from the Neolithic Revolution until the Industrial Revolution.
See also
参见
参见
References
参考资料
参考资料
- ↑ "The Computational Social Science Society of the Americas official website".
- ↑ DT&SC 7-1: . Introduction to e-Science: From the DT&SC online course at the University of California
- ↑ Hilbert, M. (2015). e-Science for Digital Development: ICT4ICT4D. Centre for Development Informatics, SEED, University of Manchester. ISBN 978-1-905469-54-3. Archived from the original on 2015-09-24. https://web.archive.org/web/20150924100018/http://www.seed.manchester.ac.uk/medialibrary/IDPM/working_papers/di/di-wp60.pdf.
- ↑ Cioffi-Revilla, Claudio (2010). "Computational social science". Wiley Interdisciplinary Reviews: Computational Statistics. 2 (3): 259–271. doi:10.1002/wics.95.
- ↑ Turchin, Peter; Brennan, Rob; Currie, Thomas E.; Feeney, Kevin C.; Francois, Pieter; Hoyer, Daniel; Manning, J. G.; Marciniak, Arkadiusz; Mullins, Daniel; Palmisano, Alessio; Peregrine, Peter; Turner, Edward A. L.; Whitehouse, Harvey (2015). "Seshat: The Global History Databank" (PDF). Cliodynamics. 6: 77. https://escholarship.org/uc/item/9qx38718
- ↑ Kirby, Kathryn R.; Gray, Russell D.; Greenhill, Simon J.; Jordan, Fiona M.; Gomes-Ng, Stephanie; Bibiko, Hans-Jörg; Blasi, Damián E.; Botero, Carlos A.; Bowern, Claire; Ember, Carol R.; Leehr, Dan; Low, Bobbi S.; McCarter, Joe; Divale, William (2016). "D-PLACE: A Global Database of Cultural, Linguistic and Environmental Diversity". PLOS One. 11 (7): e0158391. Bibcode:2016PLoSO..1158391K. doi:10.1371/journal.pone.0158391. PMC 4938595. PMID 27391016.
- ↑ Peter N. Peregrine, Atlas of Cultural Evolution, World Cultures 14(1), 2003
- ↑ "eHRAF Archaeology". Human Relations Area Files.
- ↑ "eHRAF World Cultures". Human Relations Area Files.
- ↑ Lansdall-Welfare, Thomas; Sudhahar, Saatviga; Thompson, James; Lewis, Justin; Team, FindMyPast Newspaper; Cristianini, Nello (2017-01-09). "Content analysis of 150 years of British periodicals". Proceedings of the National Academy of Sciences (in English). 114 (4): E457–E465. doi:10.1073/pnas.1606380114. ISSN 0027-8424. PMC 5278459. PMID 28069962.
- ↑ Roth, Steffen; et al. (2017). "Futures of a distributed memory. A global brain wave measurement (1800-2000)". Technological Forecasting and Social Change (in English). 118: 307–323. doi:10.1016/j.techfore.2017.02.031.
- ↑ Dzogang, Fabon; Lansdall-Welfare, Thomas; Team, FindMyPast Newspaper; Cristianini, Nello (2016-11-08). "Discovering Periodic Patterns in Historical News". PLOS One. 11 (11): e0165736. Bibcode:2016PLoSO..1165736D. doi:10.1371/journal.pone.0165736. ISSN 1932-6203. PMC 5100883. PMID 27824911.
- ↑ Seasonal Fluctuations in Collective Mood Revealed by Wikipedia Searches and Twitter Posts F Dzogang, T Lansdall-Welfare, N Cristianini - 2016 IEEE International Conference on Data Mining, Workshop on Data Mining in Human Activity Analysis
- ↑ 14.0 14.1 14.2 14.3 Salgado, Mauricio, and Nigel Gilbert. "Emergence and communication in computational sociology." Journal for the Theory of Social Behaviour 43.1 (2013): 87-110.
- ↑ Macy, Michael W., and Robert Willer. "From factors to actors: computational sociology and agent-based modeling." Annual review of sociology 28.1 (2002): 143-166.
- ↑ Durkheim, Émile. The Division of Labor in Society. New York, NY: Macmillan.
- ↑ 17.0 17.1 Bailey, Kenneth D. (2006). "Systems Theory". In Jonathan H. Turner. Handbook of Sociological Theory. New York, NY: Springer Science. pp. 379–404. ISBN 978-0-387-32458-6.
- ↑ 18.0 18.1 Bainbridge, William Sims (2007). "Computational Sociology". In Ritzer, George (ed.). Blackwell Encyclopedia of Sociology. Blackwell Reference Online. doi:10.1111/b.9781405124331.2007.x. hdl:10138/224218. ISBN 978-1-4051-2433-1.
- ↑ Crevier, D. (1993). AI: The Tumultuous History of the Search for Artificial Intelligence. New York, NY: Basic Books. https://archive.org/details/aitumultuoushist00crev.
- ↑ Durkheim, Émile. The Division of Labor in Society. New York, NY: Macmillan.
- ↑ Crevier, D. (1993). AI: The Tumultuous History of the Search for Artificial Intelligence. New York, NY: Basic Books. https://archive.org/details/aitumultuoushist00crev.
- ↑ Forrester, Jay (1971). World Dynamics. Cambridge, MA: MIT Press.
- ↑ Ignall, Edward J.; Kolesar, Peter; Walker, Warren E. (1978). "Using Simulation to Develop and Validate Analytic Models: Some Case Studies". Operations Research. 26 (2): 237–253. doi:10.1287/opre.26.2.237.
- ↑ Meadows, DL; Behrens, WW; Meadows, DH; Naill, RF; Randers, J; Zahn, EK (1974). The Dynamics of Growth in a Finite World. Cambridge, MA: MIT Press.
- ↑ 25.0 25.1 25.2 引用错误:无效
<ref>
标签;未给name属性为SfSS1
的引用提供文字 - ↑ "Computer View of Disaster Is Rebutted". The New York Times. October 18, 1974.
- ↑ Orcutt, Guy H. (1990). "From engineering to microsimulation : An autobiographical reflection". Journal of Economic Behavior & Organization. 14 (1): 5–27. doi:10.1016/0167-2681(90)90038-F.
- ↑ 28.0 28.1 28.2 28.3 28.4 引用错误:无效
<ref>
标签;未给name属性为MW
的引用提供文字 - ↑ Forrester, Jay (1971). World Dynamics. Cambridge, MA: MIT Press.
- ↑ Ignall, Edward J.; Kolesar, Peter; Walker, Warren E. (1978). "Using Simulation to Develop and Validate Analytic Models: Some Case Studies". Operations Research. 26 (2): 237–253. doi:10.1287/opre.26.2.237.
- ↑ Meadows, DL; Behrens, WW; Meadows, DH; Naill, RF; Randers, J; Zahn, EK (1974). The Dynamics of Growth in a Finite World. Cambridge, MA: MIT Press.
- ↑ "Computer View of Disaster Is Rebutted". The New York Times. October 18, 1974.
- ↑ Orcutt, Guy H. (1990). "From engineering to microsimulation : An autobiographical reflection". Journal of Economic Behavior & Organization. 14 (1): 5–27. doi:10.1016/0167-2681(90)90038-F.
- ↑ Toffoli, Tommaso; Margolus, Norman (1987). Cellular automata machines: a new environment for modeling. Cambridge, MA: MIT Press. https://archive.org/details/cellularautomata00toff.
- ↑ Gilbert, Nigel (1997). "A simulation of the structure of academic science". Sociological Research Online. 2 (2): 1–15. doi:10.5153/sro.85. Archived from the original on 1998-05-24. Retrieved 2009-12-16.
- ↑ Axelrod, Robert; Hamilton, William D. (March 27, 1981). "The Evolution of Cooperation". Science. 211 (4489): 1390–1396. Bibcode:1981Sci...211.1390A. doi:10.1126/science.7466396. PMID 7466396.
- ↑ 37.0 37.1 37.2 引用错误:无效
<ref>
标签;未给name属性为Cooperation
的引用提供文字 - ↑ Toffoli, Tommaso; Margolus, Norman (1987). Cellular automata machines: a new environment for modeling. Cambridge, MA: MIT Press. https://archive.org/details/cellularautomata00toff.
- ↑ Gilbert, Nigel (1997). "A simulation of the structure of academic science". Sociological Research Online. 2 (2): 1–15. doi:10.5153/sro.85. Archived from the original on 1998-05-24. Retrieved 2009-12-16.
- ↑ Axelrod, Robert; Hamilton, William D. (March 27, 1981). "The Evolution of Cooperation". Science. 211 (4489): 1390–1396. Bibcode:1981Sci...211.1390A. doi:10.1126/science.7466396. PMID 7466396.
- ↑ Gilbert, Nigel (1997). "A simulation of the structure of academic science". Sociological Research Online. 2 (2): 1–15. doi:10.5153/sro.85. Archived from the original on 1998-05-24. Retrieved 2009-12-16.
- ↑ Axelrod, Robert; Hamilton, William D. (March 27, 1981). "The Evolution of Cooperation". Science. 211 (4489): 1390–1396. Bibcode:1981Sci...211.1390A. doi:10.1126/science.7466396. PMID 7466396.
- ↑ Freeman, Linton C. (2004). The Development of Social Network Analysis: A Study in the Sociology of Science. Vancouver, BC: Empirical Press.
- ↑ Lazer, David; Pentland, Alex; Adamic, L; Aral, S; Barabasi, AL; Brewer, D; Christakis, N; Contractor, N; et al. (February 6, 2009). "Life in the network: the coming age of computational social science". Science. 323 (5915): 721–723. doi:10.1126/science.1167742. PMC 2745217. PMID 19197046.
- ↑ Srivastava, Jaideep; Cooley, Robert; Deshpande, Mukund; Tan, Pang-Ning (2000). "Web usage mining: discovery and applications of usage patterns from Web data". Proceedings of the ACM Conference on Knowledge Discovery and Data Mining. 1 (2): 12–23. doi:10.1145/846183.846188.
- ↑ Brin, Sergey; Page, Lawrence (April 1998). "The anatomy of a large-scale hypertextual Web search engine". Computer Networks and ISDN Systems. 30 (1–7): 107–117. CiteSeerX 10.1.1.115.5930. doi:10.1016/S0169-7552(98)00110-X.
- ↑ 47.0 47.1 S Sudhahar; GA Veltri; N Cristianini (2015). "Automated analysis of the US presidential elections using Big Data and network analysis". Big Data & Society. 2 (1): 1–28. doi:10.1177/2053951715572916.
- ↑ S Sudhahar; G De Fazio; R Franzosi; N Cristianini (2013). "Network analysis of narrative content in large corpora" (PDF). Natural Language Engineering. 21 (1): 1–32. doi:10.1017/S1351324913000247.
- ↑ Franzosi, Roberto (2010). Quantitative Narrative Analysis. Emory University.
- ↑ I. Flaounas; M. Turchi; O. Ali; N. Fyson; T. De Bie; N. Mosdell; J. Lewis; N. Cristianini (2010). "The Structure of EU Mediasphere" (PDF). PLOS One. 5 (12): e14243. Bibcode:2010PLoSO...514243F. doi:10.1371/journal.pone.0014243. PMC 2999531. PMID 21170383.
- ↑ V Lampos; N Cristianini (2012). "Nowcasting Events from the Social Web with Statistical Learning" (PDF). ACM Transactions on Intelligent Systems and Technology. 3 (4): 72. doi:10.1145/2337542.2337557.
- ↑ I. Flaounas; O. Ali; M. Turchi; T Snowsill; F Nicart; T De Bie; N Cristianini (2011). NOAM: news outlets analysis and monitoring system (PDF). Proc. of the 2011 ACM SIGMOD international conference on Management of data. doi:10.1145/1989323.1989474.
- ↑ N Cristianini (2011). "Automatic Discovery of Patterns in Media Content". Combinatorial Pattern Matching. Lecture Notes in Computer Science. 6661. pp. 2–13. doi:10.1007/978-3-642-21458-5_2. ISBN 978-3-642-21457-8.
- ↑ Lansdall-Welfare, Thomas; Sudhahar, Saatviga; Thompson, James; Lewis, Justin; Team, FindMyPast Newspaper; Cristianini, Nello (2017-01-09). "Content analysis of 150 years of British periodicals". Proceedings of the National Academy of Sciences (in English). 114 (4): E457–E465. doi:10.1073/pnas.1606380114. ISSN 0027-8424. PMC 5278459. PMID 28069962.
- ↑ I. Flaounas; O. Ali; M. Turchi; T. Lansdall-Welfare; T. De Bie; N. Mosdell; J. Lewis; N. Cristianini (2012). "Research methods in the age of digital journalism". Digital Journalism. 1: 102–116. doi:10.1080/21670811.2012.714928.
- ↑ T Lansdall-Welfare; V Lampos; N Cristianini. Effects of the Recession on Public Mood in the UK (PDF). Proceedings of the 21st International Conference on World Wide Web. Mining Social Network Dynamics (MSND) session on Social Media Applications. New York, NY, USA. pp. 1221–1226. doi:10.1145/2187980.2188264.
- ↑ Dzogang, Fabon; Lansdall-Welfare, Thomas; Team, FindMyPast Newspaper; Cristianini, Nello (2016-11-08). "Discovering Periodic Patterns in Historical News". PLOS One. 11 (11): e0165736. Bibcode:2016PLoSO..1165736D. doi:10.1371/journal.pone.0165736. ISSN 1932-6203. PMC 5100883. PMID 27824911.
- ↑ Seasonal Fluctuations in Collective Mood Revealed by Wikipedia Searches and Twitter Posts F Dzogang, T Lansdall-Welfare, N Cristianini - 2016 IEEE International Conference on Data Mining, Workshop on Data Mining in Human Activity Analysis
- ↑ 59.0 59.1 59.2 引用错误:无效
<ref>
标签;未给name属性为CCS-MMT
的引用提供文字 - ↑ Luhmann, Niklas (1982). The Differentiation of Society. New York, NY: Columbia University Press.
- ↑ Carley, Kathleen M. (2003), "Dynamic Network Analysis." Dynamic Social Network Modeling and Analysis: Workshop Summary and Papers, Ronald Breiger, Kathleen Carley, and Philippa Pattison (eds.), National Research Council (Committee on Human Factors): Washington, D.C.: 133–145.
- ↑ Barabási, Albert-László (2003). Linked: The New Science of Networks. Cambridge, MA: Perseus Publishing.
- ↑ Freeman, Linton C. (2004). The Development of Social Network Analysis: A Study in the Sociology of Science. Vancouver Canada: Empirical Press.
- ↑ Watts, Duncan J. (2004). "The New Science of Networks." Annual Review of Sociology, 30: 243–270.
- ↑ Gilbert, Nigel and Klaus G. Troitzsch (2005). Simulation for Social Scientists, 2nd Edition. New York, NY: Open University Press.
- ↑ 66.0 66.1 Epstein, Joshua M. (2007). Generative Social Science: Studies in Agent-Based Computational Modeling. Princeton, NJ: Princeton University Press.
- ↑ Geyer, Felix and Johannes van der Zouwen (1992). "Sociocybernetics." Handbook of Cybernetics, C.V. Negoita (ed.): 95–124. New York: Marcel Dekker.
- ↑ Stewart, Peter (2001). "Complexity Theories, Social Theory, and the Question of Social Complexity." Philosophy of the Social Sciences, 31(3): 323–360.
- ↑ Lee, Ju-Sung. (2001). "Evolving Drug Networks." Carnegie Mellon Center for Computational Analysis of Social and Organizational Systems (CASOS) Conference Presentation (unpublished).
- ↑ Carley, Kathleen (2003). "Destabilizing Terrorist Networks." Proceedings of the 8th International Command and Control Research and Technology Symposium. Conference held at the National Defense War College: Washington D.C., Evidence Based Research, Track 3. (Electronic Publication). -{zh-cn:互联网档案馆; zh-tw:網際網路檔案館; zh-hk:互聯網檔案館;}-的存檔,存档日期2004-12-18.
- ↑ Leydesdorff, Loet (1995). The Challenge of Scientometrics: The development, measurement, and self-organization of scientific communications. Leiden: DSWO Press, Leiden University.
- ↑ Dimitrov, Vladimir and Robert Woog (1997). "Studying Social Complexity: From Soft to Virtual Systems Methodology." Complex Systems, 11:(6).
- ↑ Saberi, Mohammad Karim, Alireza Isfandyari-Moghaddam and Sedigheh Mohamadesmaeil (2011). "Web Citations Analysis of the JASSS: the First Ten Years." Journal of Artificial Societies and Social Simulation, 14:(4), 22.
- ↑ Nowak, Martin and Roger Highfield (2011). Super Cooperators: Altruism, Evolution, and Why We Need Each Other to Succeed. New York, NY: Free Press.
- ↑ Hang, Ye, Fei Tan, Mei Ding, Yongmin Jia and Yefeng Chen (2011). "Sympathy and Punishment: Evolution of Cooperation in Public Goods Game." Journal of Artificial Societies and Social Simulation, 14(4): 20.
- ↑ Braha, D., & de Aguiar, M. A. (2016). Voting Contagion. arXiv preprint arXiv:1610.04406.
- ↑ Braha, D., & de Aguiar, M. A. (2017). Voting contagion: Modeling and analysis of a century of U.S. presidential elections. PLoS ONE 12(5): e0177970. https://doi.org/10.1371/journal.pone.0177970
- ↑ Mason, Mark (2008). Complexity Theory and the Philosophy of Education. Hoboken, NJ: Wiley-Blackwell (Educational Philosophy and Theory Special Issues).
- ↑ Castellani, Brian. (2018). "The Defiance of Global Commitment: A Complex Social Psychology. Routledge complexity in social science series." doi:10.4324/9781351137140.
- ↑ Braha, Dan. (2012). "Global Civil Unrest: Contagion, Self-Organization, and Prediction." PLoS ONE 7(10): e48596. doi:10.1371/journal.pone .0048596.
- ↑ Lohmann Susanne (1994). "Dynamics of Informational Cascades: The Monday Demonstrations in Leipzig, East Germany, 1989–1991," World Politics, 47: 42–101.
- ↑ Chesters, Graeme and Ian Welsh (2006). Complexity and Social Movements: Protest at the Edge of Chaos." London: Routledge (International Library of Sociology).
- ↑ Castellani, Brian et al. (2011). "Addressing the U.S. Financial/Housing Crisis: Pareto, Schelling and Social Mobility."Working Paper.
- ↑ Hedström, Peter and Yvonne Åberg (2011). "Social interaction and youth unemployment." Analytical Sociology and Social Mechanisms, Pierre Demeulenaere (ed.). Cambridge: Cambridge University Press.
- ↑ Yilmaz, Levent (2011). "Toward Multi-Level, Multi-Theoretical Model Portfolios for Scientific Enterprise Workforce Dynamics." Journal of Artificial Societies and Social Simulation, 14(4): 2.
- ↑ Dan Braha, Blake Stacey and Yaneer Bar-Yam. (2011). "Corporate Competition: A Self-Organizing Network." Social Networks, 33(3): 219-230.
- ↑ Jervis, Robert (1998). System Effects: Complexity in Political and Social Life. Princeton, NJ: Princeton University Press.
- ↑ Elliott, Euel and L. Douglas Kiel (eds.) (2000). Nonlinear Dynamics, Complexity and Public Policy. Hauppauge NY: Nova Science Publishers.
- ↑ Brian Castellani, Rajeev Rajaram, J. Galen Buckwalter, Michael Ball and Frederic Hafferty (2012). "Place and Health as Complex Systems: A Case Study and Empirical Test". SpringerBriefs in Public Health.
- ↑ Leydesdorff, Loet (2006). The Knowledge-Based Economy Modeled, Measured, Simulated. Boca Raton, FL: Universal-Publishers .
- ↑ Lane, D.; Pumain, D.; Leeuw, S.E. van der; West, G. (eds.) (2009). Complexity Perspectives in Innovation and Social Change. New York, NY: Springer (Methodos Series, Vol. 7).
External links
外部链接
外部链接
- PAAA: Pan-Asian Association for Agent-based Approach in Social Systems Sciences
- CSSSA: Computational Social Science Society of the Americas
- "Life in the network: the coming age of computational social science". Retrieved June 10, 2015.
Category:Social sciences
类别: 社会科学
Category:Computational science
类别: 计算科学
Category:Computational fields of study
类别: 研究的计算领域
This page was moved from wikipedia:en:Computational social science. Its edit history can be viewed at 计算社会科学/edithistory