神经雪崩
此词条由神经动力学读书会词条梳理志愿者Spidey0o0Zheng翻译审校,未经专家审核,带来阅读不便,请见谅。
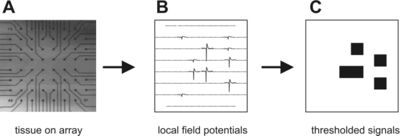
神经雪崩是神经元网络中的一连串爆发性活动,其大小分布可以用幂律来近似,如临界沙堆模型(Bak等人,1987)。神经雪崩见于培养的和急性皮质切片(Beggs和Plenz,2003;2004)。在这些新皮层切片中,活动的特点是持续几十毫秒的短暂爆发,中间有几秒钟的静止期。当用多电极阵列观察时,在爆发期间被驱动超过阈值的电极数量近似于幂律分布。虽然这种现象具有高度的稳定性和可重复性,但它与完整大脑中的生理过程的关系目前还不清楚。
实验观察
幂律尺寸分布
这个片段说明,多通道数据可以被分解为没有活动的帧和至少有一个活动电极的帧,这些电极可能接收来自几个神经元的活动。由非活动帧包围的连续活动帧序列可以称为雪崩。所示的雪崩例子的大小为9,因为这是被驱动超过阈值的电极总数。雪崩大小的分布方式几乎符合幂律分布。由于阵列中的电极数量有限,幂律在阵列大小为60之前就开始向下弯曲切断,但是对于更大的电极阵列,可以看到幂律会延伸得更远。
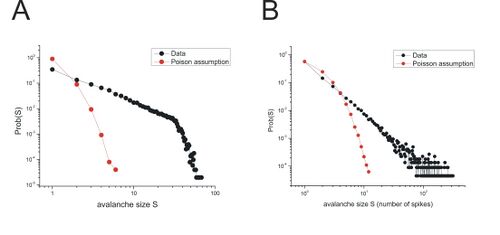
幂律分布的公式是:
- [math]\displaystyle{ P(S)=kS^{-\alpha}\, }[/math]
其中[math]\displaystyle{ P(S) }[/math]是观察到大小为[math]\displaystyle{ S }[/math]的雪崩的概率,[math]\displaystyle{ \alpha }[/math]是给出对数图中幂律斜率的指数,[math]\displaystyle{ k }[/math]是比例常数。对于切片培养实验,局部场电位雪崩的大小分布的指数[math]\displaystyle{ \alpha\approx 1.5 }[/math],但在不同阵列的尖峰记录中,指数[math]\displaystyle{ \alpha\approx2.1 }[/math]。指数差异背后的原因仍在探索中。需要注意的是,如果每个电极上的活动是独立驱动的,则幂律分布不是预期的分布。非耦合类泊松过程的集合将导致事件大小的指数分布。此外,虽然神经科学多年来在单个时间序列数据的时间相关性中报告了幂律(例如,脑电图的功率谱(Linkenkaer-Hansen等人,2001;Worrell等人,2002),峰值计数统计中的Fano或Allan因子(Teich等人,1997),神经递质分泌时间(Lowen等人,1997),离子通道波动(Toib等人,1998),神经元培养中的爆发间期(Segev等人,2002)),从多电极数据中观察到的相互作用中未观察到。因此,神经雪崩是从分布式网络中的集体过程中产生的。
重复的雪崩模式
临界沙堆模型中的雪崩在其形成的模式中是随机的,与之相比,局部场电位的雪崩发生的时空模式比预期的偶然性更频繁(Beggs和Plenz,2004)。图中显示了一个急性皮层切片的几个这样的模式。这些模式在长达10个小时的时间内是可重复的,其时间精度为4ms(Beggs和Plenz,2004)。这些模式的稳定性和精确性表明,神经雪崩可以被神经网络用作存储信息的基底。在这个意义上,雪崩似乎与在动物执行认知任务时在体内观察到的动作电位序列相似。目前还不清楚体内数据的重复活动模式是否也是雪崩。
普遍性
在上述示例中,雪崩是在浸泡在培养基中的皮层切片培养中产生的,但当急性皮层切片在含有多巴胺激动剂和NMDA(Beggs和Plenz,2003;Stewart和Plenz,2006)或高K+和低Mg2+的人工脑脊液中浸泡时,也可能在急性皮层切片中产生雪崩。诱发雪崩的不同方式表明,它们不仅仅局限于一组实验条件。
其他系统中的初步报告
在离体水蛭神经节(V.Torre,conference talk)的棘波和分离皮层培养的棘波(L.Bettencourt;R.Alessio,personal communications)中也观察到序列大小的幂律分布,这表明雪崩现象可能在体外制剂中相当普遍。初步报告还表明,在清醒和休息的灵长类动物的表层皮层中存在雪崩(Petermann等人,2006年)。这些报告尚未发表,在此仅表明研究人员目前正在探索各种制剂中的雪崩概念。
雪崩模型
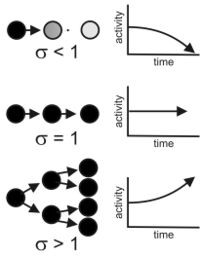
明确预测神经活动雪崩的模型包括Herz和Hopfield(1995)的工作,该模型将神经网络中的混响与地震大小的幂律分布联系起来。同样值得注意的是Eurich、Hermann和Ernst(2002)的工作,他们预测来自全局耦合的非线性阈值元素网络的雪崩大小分布应该有一个[math]\displaystyle{ \alpha=1.5 }[/math]的指数。值得注意的是,这个指数与实验报告相吻合(Beggs和Plenz,2003)。
这里更详细地描述了一个分支过程模型(Harris, 1989; Beggs and Plenz, 2003; Haldeman and Beggs, 2005; reviewed in Vogels et al, 2005),因为它既能捕捉到雪崩大小的幂律分布,又能观察到数据中可重复的活动序列。在该模型中,在一个时间步长处于活动状态的处理单元将在下一个时间步长中平均产生[math]\displaystyle{ \sigma }[/math]处理单元中的活动。[math]\displaystyle{ \sigma }[/math]被称为==分支参数==,可以被认为是这个比率的预期值。
- [math]\displaystyle{ \sigma=\frac{\mbox{Descendants}}{\mbox{Ancestors}} }[/math]
where Ancestors is the number of processing units active at time step t and Descendants is the number of processing units active at time step t + 1. There are three general regimes for [math]\displaystyle{ \sigma\ , }[/math] as shown in the figure.
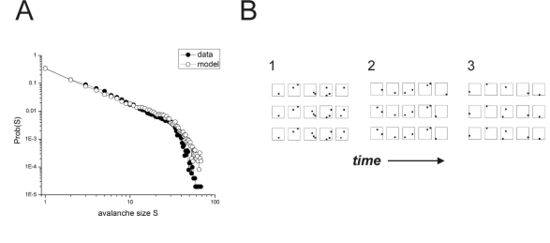
At the level of a single processing unit in the network, the branching parameter [math]\displaystyle{ \sigma }[/math] is set by the following relationship:
- [math]\displaystyle{ \sigma_i=\sum_{j=1}^\mathit{N} \mathit{p_{ij}} }[/math]
where [math]\displaystyle{ \sigma_i }[/math] is the expected number of descendant processing units activated by unit [math]\displaystyle{ i\ , }[/math] [math]\displaystyle{ N }[/math] is the number of units that unit [math]\displaystyle{ i }[/math] connects to, and [math]\displaystyle{ p_{ij} }[/math] is the probability that activity in unit [math]\displaystyle{ i }[/math] will transmit to unit [math]\displaystyle{ j\ . }[/math] Because some transmission probabilities are greater than others, preferred paths of transmission may occur, leading to reproducible avalanche patterns. Both the power law distribution of avalanche sizes and the repeating avalanches are qualitatively captured by this model when [math]\displaystyle{ \sigma }[/math] is tuned to the critical point ([math]\displaystyle{ \sigma=1 }[/math]), as shown in the figure (Haldeman and Beggs, 2005). When the model is tuned moderately above ([math]\displaystyle{ \sigma\gt 1 }[/math]) or below ([math]\displaystyle{ \sigma\lt 1 }[/math]) the critical point, it fails to produce a power law distribution of avalanche sizes. This phenomenological model does not explicitly state the cellular or synaptic mechanisms that may underlie the branching process, and many of this model's predictions need to be tested.
雪崩的影响
当一个可调谐系统运行在一个产生幂律分布的系统中时,它被说成是运行在临界点。严格地说,只有无限大的系统才能在临界点上运行,但这里的"临界"一词是用来描述有限系统的行为,如果它们被扩展到无限大,就会接近临界点。幂律雪崩大小分布对这四个领域的神经网络的信息处理有潜在的影响。
- 信息传输。当神经网络调整到临界点时,它们具有最佳的信息传输(Beggs和Plenz,2003;Bertschinger和Natschlager,2004;Kinouchi和Copelli,2006),因为在强信号传播和抗饱和之间存在平衡。
- 信息存储。当一个基于分支过程的递归网络被调整到临界点时,显著重复的雪崩模式的数量被最大化(Haldeman和Beggs,2005)。在临界点,存在着强连接和弱连接的混合,允许各种独立稳定的活动模式。
- 计算能力。通过改变尖峰网络模型中突触重量的变化,Bertschinger和Natschlager(Bertschinger和Natschlager 2004)能够产生显示出衰减、持续和扩张活动的网络。这些区域分别对应于亚临界、临界和超临界动力学。他们发现,在广泛的计算任务中,调谐到临界点的网络比调谐到亚临界或超临界动态的网络更有效。
- 稳定性。当一个循环分支网络模型被调整到临界点时,它产生的轨迹基本上是平行的,这意味着该网络处于稳定的边缘(Bertschinger和Natschlager,2004;Haldeman和Beggs,2005)。在这种情况下,轨迹仍然是稳定的,但可以通过较小的校正输入进行控制。
当网络在发生神经雪崩的临界点附近运行时,可以同时优化所有这些信息处理任务。
神经雪崩与其他系统的关系
Power law distributions of event sizes are often seen in complex phenomena including earthquakes, phase transitions, percolation, forest fires, financial market fluctuations, avalanches in the game of life and a host of others (Bak, 1996). In some specific cases, this similarity appears to be more than superficial. For example, earthquake models incorporate local rules in which forces at one site are distributed to nearest neighbors without dissipation. This conservation of forces is similar to the conservation of probabilities in the critical branching model described above. This suggests that conservation of synaptic strengths, as reported in (Royer and Pare, 2003) could be a mechanism responsible for maintaining a network near the critical point. In a related idea, simulations indicate that networks can be kept nearly critical when the total sum of synaptic strengths hovers near a constant value (Hsu and Beggs, 2006). This could be accomplished through a mechanism like synaptic scaling (Turrigiano and Nelson, 2000), which has been observed experimentally. Finally, recently "burned" areas in forest fire models are refractory, while unburned areas are more likely to ignite. This balance of refractoriness and excitability combine to maintain the system near the critical point. Recent models of neuronal avalanches (Levina, Herrmann and Geisel, 2005) have suggested that short-term synaptic depression and facilitation may also serve to drive neuronal networks toward the critical point where avalanches occur. Thus, an understanding of power laws in diverse complex systems can suggest mechanisms that might underlie criticality in neuronal networks.
A simple electronic model of avalanche generation consists of a two-dimensional array of neon lamps, each one connected to a resistor towards a global DC control voltage and capacitively coupled to its von Neumann neighbors. Neon lamps possess rich dynamical properties: as the applied voltage changes, the transition between the "on" and "off" phases is at the same time significantly hysteretic and stochastic (Dance, 1968). The system displays two phases, [math]\displaystyle{ I }[/math] and [math]\displaystyle{ II }[/math], respectively characterized by low and high event rate and spatiotemporal order: the transition between them is strongly hysteretic, hence unequivocally first-order. Nevertheless, close to the spinal point of the [math]\displaystyle{ I\rightarrow II }[/math] transition, critical precursors emerge in the form of avalanches (Fig. 8) having the same scaling exponents characterizing neural activity, namely [math]\displaystyle{ \alpha\approx3/2 }[/math] for size and [math]\displaystyle{ \alpha\approx2 }[/math] for duration (Minati et al., 2016).
外部链接和致谢
这项工作的撰写和图中的实验得到了美国国家科学基金会的资助和印第安纳大学的资助,约翰·贝格斯(John Beggs)的资助号为0343636。关于神经雪崩的最初工作是在迪特玛·普伦茨(Dietmar Plenz)的实验室完成的,由美国国立卫生研究院的院内研究项目资助。
参考文献
- Bak, P. (1996). How nature works: the science of self-organized criticality. New York, NY, USA, Copernicus.
- Bak P, Tang C, Wiesenfeld K (1987). Self-organized criticality: an explanation of the 1/f noise. Phys Rev Lett 59:381–384.
- Beggs, J. M. and D. Plenz (2003). Neuronal avalanches in neocortical circuits. J Neurosci 23(35): 11167-77.
- Beggs, J. M. and D. Plenz (2004). Neuronal avalanches are diverse and precise activity patterns that are stable for many hours in cortical slice cultures. J Neurosci 24(22): 5216-29.
- Bertschinger, N. and T. Natschlager (2004). "Real-time computation at the edge of chaos in recurrent neural networks." Neural Comput 16(7): 1413-36.
- Dance J. N. (1968). "Cold Cathode Tubes." Iliffe, London, UK.
- Eurich CW, Herrmann JM, Ernst UA (2002). Finite-size effects of avalanche dynamics. Phys Rev E Stat Nonlin Soft Matter Phys 66:066137.
- Haldeman, C, Beggs, JM (2005). Critical branching captures activity in living neural networks and maximizes the number of metastable states. Phys Rev Lett, 94: 058101.
- Harris TE (1989). The theory of branching processes. New York: Dover.
- Herz AV, Hopfield JJ (1995). Earthquake cycles and neural reverberations: collective oscillations in systems with pulse-coupled threshold elements. Phys Rev Lett 75:1222–1225.
- Hsu D, Beggs JM (2006). Neuronal avalanches and criticality: A dynamical model for homeostasis. Neurocomputing 69(1134-1136).
- Kinouchi O, Copelli M (2006). Optimal dynamic range of excitable networks at criticality. Nature Physics 2:348-351.
- Levina A, Herrmann JM, Geisel T (2005). Dynamical synapses give rise to a power law distribution of neuronal avalanches. Neural Information Processing Systems (NIPS) http://books.nips.cc/papers/files/nips18/NIPS2005_0646.pdf
- Linkenkaer-Hansen K, Nikouline VV, Palva JM, Ilmoniemi RJ (2001). Long-range temporal correlations and scaling behavior in human brain oscillations. J Neurosci 21:1370–1377.
- Lowen SB, Cash SS, Poo MM, Teich MC (1997). Quantal neurotransmitter secretion rate exhibits fractal behavior. J Neurosci 17:5666–5677.
- Minati, L; de Candia, A; Scarpetta, S. "Critical phenomena at a first-order phase transition in a lattice of glow lamps: Experimental findings and analogy to neural activity". Chaos. 26: 073103. doi:10.1063/1.4954879.
- Petermann T, Lebedev M, Nicolelis M, Plenz D (2006). Neuronal avalanches in vivo. Society for Neuroscience Abstracts.
- Royer S, Pare D (2003). Conservation of total synaptic weight through balanced synaptic depression and potentiation. Nature 422(6931):518-22.
- Segev R, Benveniste M, Hulata E, Cohen N, Palevski A, Kapon E, Shapira Y, Ben Jacob E (2002). Long term behavior of lithographically prepared in vitro neuronal networks. Phys Rev Lett 88:118102.
- Stewart CV, Plenz D (2006). Inverted-U profile of dopamine-NMDA-mediated spontaneous avalanche recurrence in superficial layers of rat prefrontal cortex. J Neurosci. 2006 Aug 2;26(31):8148-59.
- Teich MC, Heneghan C, Lowen SB, Ozaki T, Kaplan E (1997). Fractal character of the neural spike train in the visual system of the cat. J Opt Soc Am A Opt Image Sci Vis. 1997 Mar;14(3):529-46.
- Toib A, Lyakhov V, Marom S (1998). Interaction between duration of activity and time course of recovery from slow inactivation in mammalian brain Na_ channels. J Neurosci 18:1893–1903.
- Torre V (2006) Talk at NeuroIT conference in Genoa, Italy: http://www.neuroengineering.it/download/20.Torre.pdf
- Turrigiano GG, and Nelson SB (2000). Hebb and homeostasis in neuronal plasticity. Curr Opin Neurobiol 10:358-364.
- Vogels, T. P., K. Rajan and L. F. Abbott (2005). Neural network dynamics. Annu Rev Neurosci 28: 357-76.
- Worrell GA, Cranstoun SD, Echauz J, Litt B (2002). Evidence for self-organized criticality in human epileptic hippocampus. NeuroReport 13:2017–2021.
Internal references
- Valentino Braitenberg (2007) Brain. Scholarpedia, 2(11):2918.
- Eugene M. Izhikevich (2006) Bursting. Scholarpedia, 1(3):1300.
- Olaf Sporns (2007) Complexity. Scholarpedia, 2(10):1623.
- Gregoire Nicolis and Catherine Rouvas-Nicolis (2007) Complex systems. Scholarpedia, 2(11):1473.
- James Meiss (2007) Dynamical systems. Scholarpedia, 2(2):1629.
- Paul L. Nunez and Ramesh Srinivasan (2007) Electroencephalogram. Scholarpedia, 2(2):1348.
- Robert Kozma (2007) Neuropercolation. Scholarpedia, 2(8):1360.
- Philip Holmes and Eric T. Shea-Brown (2006) Stability. Scholarpedia, 1(10):1838.
参见
复杂性, 复杂系统, 生命游戏, Neuropercolation, 自组织临界性, Statistical Mechanics of Neocortex